Enhancing Financial Management with Predictive Analytics
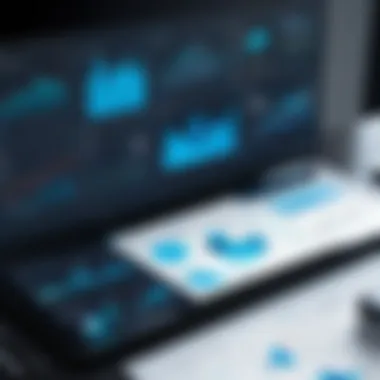
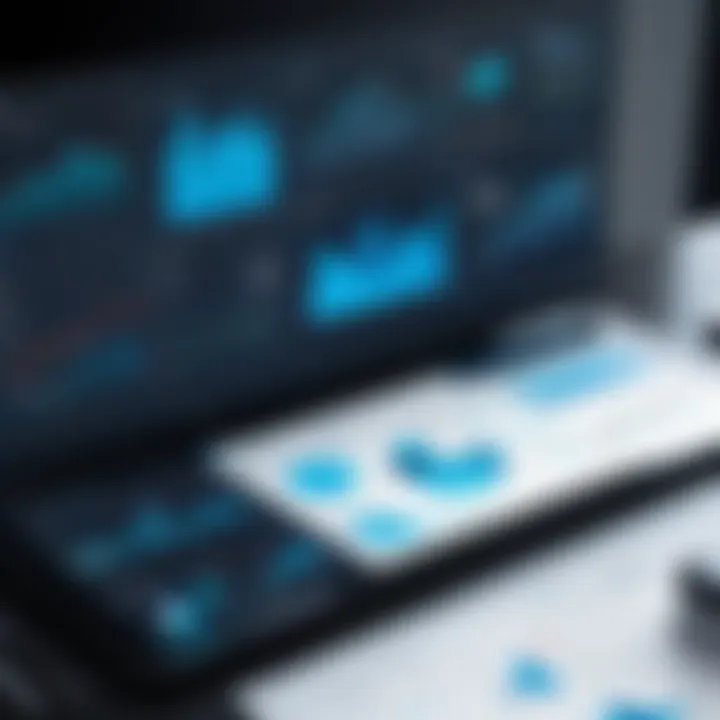
Intro
In the realm of financial management, the role of accounts payable often exists in the shadows. Yet, like a well-oiled machine humming silently, it has the potential to drive significant efficiencies when paired with modern analytical tools. Predictive analytics in accounts payable reveals patterns, forecasts cash flows, and empowers organizations to make informed decisions that can transform their financial strategies.
The competitive landscape today requires businesses to pivot quickly, utilizing data not just for hindsight but for foresight. Effective integration of predictive analytics can allow companies to not only streamline their operations but also enhance their strategic positioning. This is not just about crunching numbers; it’s about harvesting insights from the data to shape future profits.
As we navigate through the various elements of this toolkit, we will explore how organizations can harness this innovative approach to not only improve their accounts payable processes but also lead to an overall enhancement in financial management.
Brief Description
Overview of Accounts Payable Predictive Analytics
Accounts payable predictive analytics is a method of using historical data and statistical algorithms to predict future outcomes related to unpaid invoices and expenditures. This analytical approach enables organizations to foresee patterns that may inform when payments can be made, how much cash flow is anticipated, or identify potential issues with suppliers. This foresight can unlock a multitude of advantages.
Key Features and Functionalities
The utility of predictive analytics in accounts payable lays in its array of features:
- Forecasting Payment Trends: By analyzing past payment behaviors, businesses can predict future regularities and manage cash flow better.
- Risk Assessment: This tool can assess the likelihood of late payments or defaults from suppliers, minimizing supply chain risks.
- Supplier Management: Organizations can evaluate supplier performance and partnerships over time, directing negotiations and possibly realizing cost savings.
- Process Optimization: It provides valuable insights that can help streamline workflows, ensuring that nothing slips through the cracks.
Realizing the potential of these features can significantly shift the way financial teams operate, directly impacting overall financial health and organizational success.
System Requirements
Hardware Requirements
For implementing an effective predictive analytics solution, some minimum hardware requirements typically include:
- Modern multi-core processor for efficient data processing.
- At least 16GB of RAM to handle the computational load of advanced analytics.
- Sufficient storage (SSD recommended) for vast amounts of structured and unstructured data.
Software Compatibility
Most predictive analytics solutions thrive in an ecosystem supporting various software applications. Here are the essentials:
- Operating System: A recent version of Windows or Linux to ensure compatibility with analytics software.
- Database Management Systems: DBMS like Oracle, Microsoft SQL Server, or MySQL should be fully compatible to manage the underlying data.
Getting the right system in place is paramount; it serves as the backbone for data-driven insights and decision-making. Without the right resources, the potential of predictive analytics may not be fully unlocked.
"Data is the new oil, but like oil, it must be refined to be useful."
Preamble to Accounts Payable Predictive Analytics
In the fast-paced realm of finance, the incorporation of predictive analytics into accounts payable processes is not just a passing trend; it’s becoming a necessity for organizations striving to remain competitive. Within this context, accounts payable predictive analytics encompasses formulated strategies that employ historical data to forecast future outcomes. This approach not only aids in streamlining financial operations but also enhances decision-making processes, empowering businesses to take a proactive stance in their financial management practices.
Definition and Scope
Accounts payable predictive analytics can be described as the application of statistical algorithms and machine learning techniques to analyze historical spending data, vendor performance, and payment trends. This analysis leads to the derivation of actionable insights regarding future cash flows and liabilities. The scope of this analytics approach extends across various dimensions: forecasting payment cycles, understanding vendor behavior, and spotting potential late payments or disputes before they occur. Organizations can leverage this data-derived knowledge to optimize their cash management strategies, ensuring they don’t fall behind in their financial obligations while also identifying opportunities for early payment discounts.
Importance in Financial Management
The significance of predictive analytics in accounts payable cannot be understated. Here are some key reasons why it holds such importance in modern financial management:
- Enhanced Decision-Making: Organizations equipped with predictive insights can make informed decisions, reducing uncertainty and risk associated with cash flow management.
- Operational Efficiency: By anticipating payment obligations, businesses can streamline their accounts payable process, minimizing bottlenecks that often hinder operational flow.
- Strengthened Vendor Relationships: Understanding vendor patterns fosters better engagement, allowing organizations to negotiate favorable terms and build trust.
"In the world of finance, dancing with data is no longer optional; it's a competitive advantage."
With these elements in mind, it's evident that accounts payable predictive analytics serves as a backbone for strategic financial management. By using this approach, businesses can not only achieve short-term gains but also secure their long-term stability in an ever-evolving market landscape. The journey into accounts payable predictive analytics paves the way for a more insightful and data-driven era of financial organization.
Foundational Concepts of Predictive Analytics
Understanding the foundational concepts of predictive analytics is essential when it comes to enhancing financial management within the realm of accounts payable. These concepts not only provide the structure upon which predictive models are built but also clarify the processes and methodologies that underpin effective financial decision-making. By grasping these elements, organizations can better harness the power of predictive analytics to streamline their operations, minimize risks, and foster stronger vendor relationships.
Understanding Predictive Modeling
Predictive modeling is the bedrock of predictive analytics and involves the use of data, statistical algorithms, and machine learning techniques to identify the likelihood of future outcomes based on historical data. In the context of accounts payable, this modeling can be crucial in forecasting spending patterns, identifying potential late payments, or predicting cash flow fluctuations.
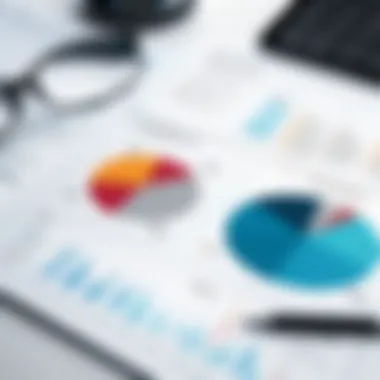
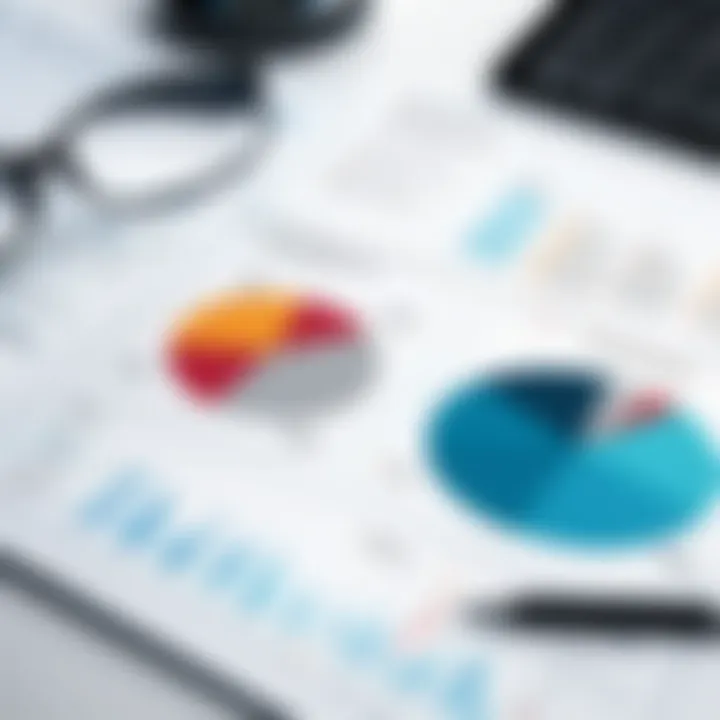
Consider this example: if a company has data showing that it consistently pays suppliers late at the end of each quarter, predictive modeling could analyze these trends to forecast future delays. By recognizing these patterns, managers can take proactive measures—such as negotiating extended payment terms or adjusting cash reserves—leading to improved financial stability.
The process typically involves several key steps:
- Data Collection: Gathering historical data is the first step. This includes invoices, payment histories, and even broader economic indicators that might influence cash flow.
- Data Preparation: Cleaning and organizing the data is crucial. Incomplete or inconsistent data can lead to misguided predictions.
- Model Selection: Choosing the right algorithm is vital. Different situations may call for regression analysis, decision trees, or neural networks.
- Validation: Testing the model to ensure it accurately predicts outcomes is essential before implementation.
- Deployment: Once validated, the model can be used in real-time decision-making processes, continuously improving as new data comes in.
Overall, predictive modeling empowers finance teams to step away from reactive measures and toward strategic, informed decision-making. By providing insights grounded in quantitative analysis, firms can make moves that not only secure their current financial footing but also position them for future growth.
Data Sources for Predictive Analytics
Data is the lifeblood of predictive analytics. Without accurate, relevant, and comprehensive data, models lose their effectiveness and insights become mere conjectures. In the realm of accounts payable, numerous data sources can feed into predictive analytics, thus shaping the decision-making processes.
- Internal Data: This includes all financial records, supplier invoices, payment histories, and employee expenses. Keeping meticulous records is indispensable as this data is often the first line of input for predictive models.
- External Market Data: Information about market trends, economic indicators, and sector-specific performance can provide critical insights that would influence accounts payable decisions. For instance, if a successful supplier is trending downwards in their market performance, a firm might need to reassess their terms of engagement.
- Social Data: With the rise of social media, businesses can gain insights into supplier reputation and customer satisfaction. Platforms such as Facebook and Reddit allow firms to monitor sentiments toward their suppliers which can influence payment strategies.
- Spend Analytics Software: Using tools like Coupa or SAP Ariba can streamline the data collection process, further enhancing compliance and visibility into spending.
- Artificial Intelligence and Machine Learning: Increasingly, organizations are incorporating AI to mine vast amounts of data. AI can detect patterns in datasets that may not be immediately apparent, providing deeper insights for predictive analytics.
Key Insight: The accuracy of predictions is directly influenced by the quality and variety of the data used. Investing in robust data management practices is, therefore, a prerequisite for success.
In summary, understanding predictive modeling and elucidating the critical data sources available creates a firm foundation for effectively harnessing accounts payable predictive analytics. This knowledge empowers organizations to make informed choices that enhance their financial management outlook.
Key Benefits of Implementing Predictive Analytics in Accounts Payable
The adoption of predictive analytics in accounts payable is not just a trend; it's a game changer for organizations aiming to enhance their financial management. By harnessing data effectively, businesses can anticipate issues before they arise, make informed decisions, and streamline their processes. The incorporation of predictive analytics offers key advantages that are crucial for financial stability, risk management, and supplier relations.
Enhanced Cash Flow Management
Cash flow is the lifeblood of any organization. Managing it well involves not just tracking what comes in and goes out, but also anticipating future movements. Predictive analytics aids in cash flow management by analyzing historical data, identifying patterns, and forecasting future expenditures.
For example, if a company regularly pays its suppliers on a 30-day cycle, using predictive analytics helps in predicting when cash outflows might peak, aligning it with anticipated cash inflows. This foresight allows businesses to maintain optimal cash levels, ensuring they are not caught short when significant payments are due.
Furthermore, adjusting payments based on the insights gained can also lead to potential discounts for early payments or improved relationships with vendors. The ability to model various scenarios can significantly boost an organization’s agility in financial planning.
Risk Mitigation
In the world of finance, risk is often seen as a necessary evil. However, companies can take proactive measures to mitigate risks using predictive analytics. By scrutinizing past payment patterns, organizations can identify red flags such as late payments or frequent disputes with certain vendors, which may indicate underlying issues.
Developing predictive models for these risks allows businesses to prepare and strategize accordingly. For instance, if a company realizes that a particular supplier has a history of late shipments, they can take steps to diversify their vendor base or negotiate more favorable terms before issues occur.
Data analysis can also point to anomalies that may suggest fraudulent activities. Noticing an unusually high expense from a vendor suddenly can prompt immediate internal reviews, sparing the company from potentially significant losses. As a result, implementing predictive analytics becomes a vital tool in the financial toolkit, not merely for compliance but as a strategic imperative in risk reduction.
Improved Vendor Relationship Management
In any business, maintaining good relationships with vendors is essential for smooth operations. Predictive analytics aids this by presenting a clearer picture of spending habits and supplier performance. By utilizing analytical tools, organizations can evaluate vendor reliability, consistency, and pricing trends over time.
For instance, if a business identifies that a vendor usually falls short on delivery but offers the best prices, it can make informed choices on how to approach future contracts. Furthermore, the insights gained can help in negotiating better terms based on historical performances, creating a win-win situation.
Moreover, consistent performance evaluations pave the way for building trust. Organizations can engage actively with their vendors, addressing issues highlighted by data while recognizing areas of excellence. A dynamic relationship built on data-driven insights can lead to collaborative improvements and innovation, thus enhancing the overall effectiveness of the supply chain.
The blend of predictive analytics and accounts payable isn’t just about numbers; it’s about crafting a narrative through data, creating opportunities for both financial foresight and strategic vendor partnerships.
In summary, the key benefits of implementing predictive analytics in accounts payable include enhanced cash flow management, risk mitigation, and improved vendor relationship management. Each aspect contributes significantly to a company’s bottom line and overall financial health, thus making it a crucial element of modern financial management.
Challenges in Integrating Predictive Analytics
Integrating predictive analytics into accounts payable isn’t all sunshine and rainbows. There are several hurdles that organizations face as they navigate this transformation. Understanding these challenges is key to leveraging predictive analytics effectively. Not only does each of these obstacles pose risks, but they also offer opportunities for growth and enhancement in financial management practices.
Data Quality and Consistency Issues
When it comes to predictive analytics, data quality is paramount. Without reliable data, the insights drawn from predictive models can be misleading, leading decision-makers down the wrong path. Inaccurate or inconsistent data can stem from various sources like duplicated entries, outdated information, or lack of standardization across departments.
To put it in simple terms, if your data is wonky, your predictions will be too. An organization may miss critical patterns that could be indicative of cash flow issues, late payments, or vendor reliability concerns. Moreover, a lack of cohesive data can hinder the effectiveness of machine learning algorithms, as they thrive on rich, clean datasets.
Some strategies to tackle these data quality issues include:
- Regular Audits: Periodically check and clean the dataset to ensure its accuracy and reliability.
- Standardization: Create data entry guidelines to ensure consistency across the board.
- Investment in Tools: Utilize proper tools and technologies designed for data management to automate the cleansing process.
Ultimately, addressing data quality can dramatically improve the predictive capabilities of accounts payable systems.
Resistance to Change in Organizational Culture
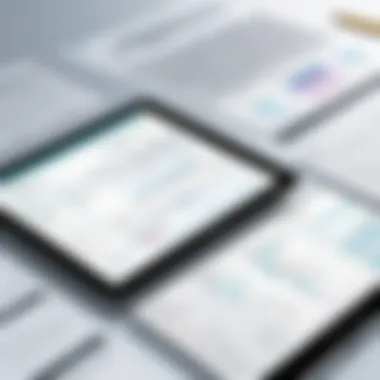
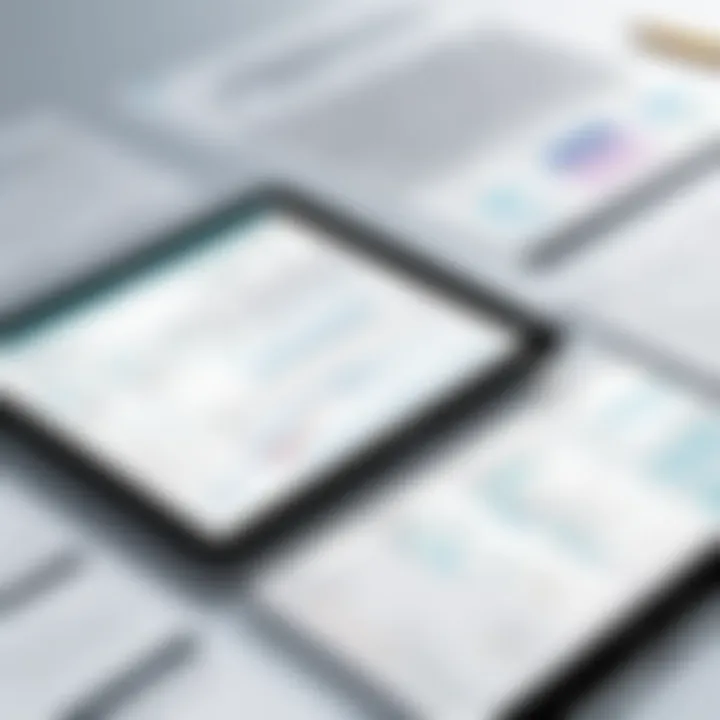
Change is tough, especially in well-established organizations with set ways of doing things. Integrating predictive analytics often clashes with traditional mindsets. Employees might feel uneasy about shifting from manual processes or be skeptical about relying on algorithms for critical financial insights.
This resistance is rooted in fear of the unknown. Employees may worry about job security or that they lack the necessary skills to adapt to new technologies. Such sentiments can create a rift within the team, hindering collaboration initiatives intended to streamline accounts payable processes.
Engaging stakeholders early in the process can help mitigate these concerns. It is essential to:
- Communicate the Benefits: Clearly articulate how predictive analytics can simplify workloads and enhance decision-making.
- Provide Training: Offer comprehensive training sessions to ease the transition. Help team members feel confident in leveraging new tools and navigating data-driven insights.
- Celebrate Small Wins: Acknowledge initial successes to foster a sense of achievement and encourage a culture open to change.
Effective integration of predictive analytics demands a culture that embraces continuous learning and adaptation.
Navigating these challenges is no easy feat but recognizing the importance of data quality and addressing cultural resistance can significantly facilitate a smoother transition toward leveraging predictive analytics in accounts payable. It’s all about turning obstacles into stepping stones for improved financial management.
Case Studies: Successful Implementation of Predictive Analytics
In the world of finance, theory only takes you so far. Nothing resonates quite like real-world examples that showcase the tangible benefits of predictive analytics in accounts payable. These case studies serve as a roadmap, illustrating how different organizations have embraced this technology to transform their financial management practices. By dissecting specific implementations, we gain valuable insights into their challenges, the strategies they adopted, and the overall results they achieved. This deeper understanding not only demystifies the application of predictive analytics but also paves the way for other businesses to follow suit.
Corporate Example One
Let's take a closer look at a prominent retail chain, Target Corporation. Target faced the annual challenge of managing extensive vendor relationships and optimizing cash flow. Compounded by the complexity of numerous transactions, the company sought a better way to predict payment timings and identify potential liquidity issues.
By implementing a predictive analytics tool, Target was able to analyze historical payment data and use it to forecast future liabilities with remarkable precision. They utilized machine learning algorithms, analyzing patterns based on payment terms, seasonal trends, and economic indicators. The results were staggering:
- Improved Cash Flow: Target reduced instances of late payments, thus avoiding penalties and strengthening relationships with suppliers.
- Tailored Payment Strategies: The analytics enabled the finance team to adopt more strategic payment schedules, balancing cash flow while taking advantage of early payment discounts.
The transformation didn’t happen overnight. Target needed to train their employees on the new systems and also ensure data quality was high. However, the initiative has significantly optimized their accounts payable operations, leading to a more agile financial framework.
Corporate Example Two
Next, let’s consider a large manufacturing firm, Siemens. Their sprawling global operations meant they had thousands of transactions across various currencies and regulatory environments. Siemens aimed at harmonizing their financial processes while ensuring compliance with local regulations.
Through the integration of a predictive analytics platform, Siemens could consolidate data from multiple sources, delivering real-time insights into their accounts payable. They specifically harnessed natural language processing to sift through unstructured data from invoices and contracts. Here’s what they achieved:
- Streamlined Processes: Significant reduction in processing time for invoices led to increased efficiency in managing payables.
- Risk Management: The predictive capability allowed Siemens to identify vendors at risk of financial distress, enabling proactive risk mitigation strategies.
The real achievement at Siemens was the cultural shift. Employees began to understand the value of data in driving business decisions, thus fostering a culture of data-driven decision-making. By showcasing success stories like these, other organizations can draw inspiration and build their path toward leveraging predictive analytics effectively.
"The application of predictive analytics in accounts payable has not only enhanced operational efficiency but also reinforced financial discipline across various sectors."
Tools and Technologies for Accounts Payable Predictive Analytics
In the realm of financial management, the significance of utilizing the right tools and technologies for accounts payable predictive analytics cannot be overstated. These solutions serve as the backbone for data-driven decisions, enabling organizations to efficiently manage their cash flows and optimize operational performance.
Organizations must grasp that not all tools are created equal. Finding the right fit goes beyond just purchasing something off the shelf; it involves a thorough evaluation of needs, budget, and integration capabilities. Companies must look for solutions that not only provide robust analytics capabilities but also seamlessly integrate with existing systems.
The ever-evolving tech landscape means staying ahead of the curve is necessary. Technologies such as cloud computing and artificial intelligence are game changers in accounts payable processes. Leveraging these innovations can lead to enhanced accuracy in predicting cash flow trends and streamlining operations.
Software Solutions Overview
When it comes to software solutions for accounts payable predictive analytics, several players stand out. Major platforms like SAP Ariba, Oracle NetSuite, and Coupa offer comprehensive tools that provide detailed insights through advanced analytics features.
- SAP Ariba: Known for its supplier management functionalities, it combines procurement and financial management, offering predictive analytics to optimize spending and cash flow.
- Oracle NetSuite: This cloud-based solution provides real-time data for better decision-making in accounts payable, accounting, and finance.
- Coupa: Focused on visibility and control over spending, Coupa integrates predictive analytics to forecast trends based on historical financial data.
Each of these platforms brings something unique, addressing diverse organizational needs. It's crucial to evaluate these based on specific requirements, such as scalability, ease of integration, and user-friendliness.
Emerging Technologies to Watch
The future of accounts payable predictive analytics lies in the hands of several emerging technologies that are set to reshape how organizations manage their financial processes. Understanding these can provide a competitive edge.
- Artificial Intelligence (AI): AI is rapidly altering how predictive analytics functions. Machine learning algorithms analyze sprawling datasets, providing insights that were previously unattainable.
- Blockchain Technology: While often associated with cryptocurrencies, blockchain offers integrity and transparency in transactions, enhancing trust amongst providers.
- Robotic Process Automation (RPA): RPA can streamline manual tasks in accounts payable, reducing errors and enabling employees to focus on strategic activities.
These technologies converge to create a more efficient financial ecosystem, enhancing predictive capabilities and enabling smarter decision-making.
Important Insight: Technologies like machine learning and blockchain are not merely trends; they represent the future of financial management. Organizations that adapt early can secure a substantial advantage in their operational effectiveness.
Ultimately, the right tools and technologies for accounts payable predictive analytics transform financial management practices, enabling organizations to navigate challenges with greater agility and precision. Investing in robust software solutions and keeping an eye on emerging technologies can help pave the way for enhanced financial performance.
Future Trends in Accounts Payable Predictive Analytics
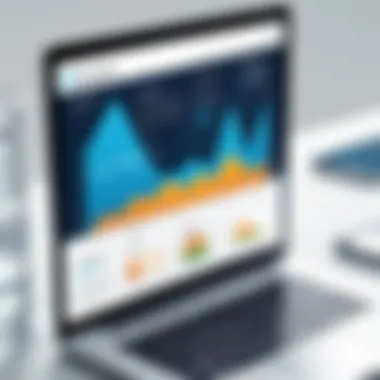
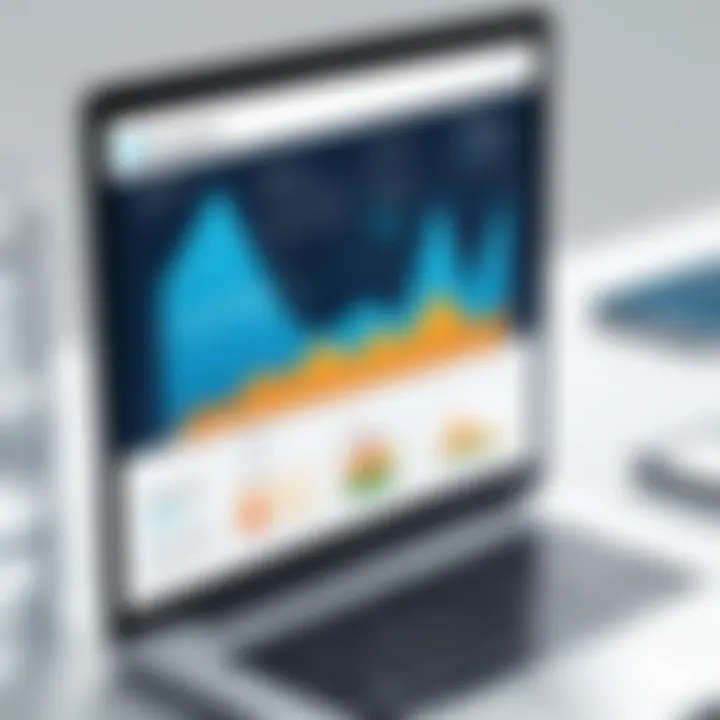
In the rapidly evolving world of finance, the role of predictive analytics in accounts payable is becoming increasingly apparent. Companies now find themselves at a juncture where adopting advanced analytics not only enhances operational efficiency but also drives strategic decision-making. Future trends in accounts payable predictive analytics are set to reshape how organizations approach financial management and streamline processes. The significance of keeping an eye on these emerging trends cannot be underestimated, as they present new avenues for businesses to gain a competitive edge.
AI and Machine Learning Innovations
Artificial Intelligence and machine learning are not just buzzwords; they represent a formidable shift in how data is utilized in financial operations. With the ability to analyze vast amounts of data quickly and accurately, these technologies offer substantial promise for accounts payable functions. There are several key advantages to leveraging AI and machine learning in this context:
- Enhanced Forecasting: By utilizing historical data combined with machine learning algorithms, companies can predict future expenses more accurately. This allows businesses to manage cash flow effectively and avoid unexpected financial pitfalls.
- Automated Decision-Making: AI can identify anomalies and make decisions in real-time, ensuring that potential issues, such as duplicate payments or fraudulent invoices, are flagged immediately. This layer of security not only saves time but also mitigates risk.
- Dynamic Vendor Management: By analyzing vendor performance and payment terms, organizations can adjust their strategies for negotiating contracts and managing cash flow to optimize financial outcomes.
Furthermore, the integration of AI enables a more adaptive approach to financial management. For example, suppose a large manufacturing firm experiences an increase in direct material costs. In that case, machine learning can quickly analyze supplier trends and suggest renegotiation prompts or alternative sourcing approaches to maintain competitive pricing. This responsiveness is crucial in an uncertain economic landscape.
Increased Automation in Financial Processes
Automation is another formidable trend that significantly influences accounts payable predictive analytics. As manual processes become obsolete, businesses are turning to automated solutions to tackle routine tasks and redundancies. Key benefits of increased automation include:
- Time Efficiency: Automating data entry, approval processes, and invoice matching reduces the man-hours involved in conventional accounts payable workflows. This makes the entire process faster and more efficient, allowing teams to focus on strategic initiatives rather than mundane tasks.
- Cost Reduction: By minimizing human intervention, companies can lower labor costs and reduce the risk of errors associated with manual processing. Over time, this translates into substantial savings.
- Scalability: As organizations grow, their transaction volumes can increase significantly. Automated systems can easily handle this expanded workload without the need for additional resources. This scalability is essential for both small and large businesses alike, ensuring they can adapt seamlessly as their operations evolve.
To illustrate, consider a mid-sized retail company that has implemented an automated accounts payable system. By doing so, the company is able to process invoices three times faster while also achieving greater accuracy. This allows the finance team to focus efforts on strategic vendor negotiations rather than getting bogged down in paperwork.
In summary, as predictive analytics in accounts payable continues to evolve, it brings forth significant innovations in AI and automation. Keeping a pulse on these advancements is vital for organizations seeking to harness the full potential of their financial management capabilities. Embracing these trends not only fosters operational excellence but also builds a foundation for making informed, data-driven decisions.
"Incorporating AI and automation can act like adding nitrous oxide to a car engine: it supercharges performance and accelerates growth in ways previously thought impossible."
By integrating these emerging technologies, businesses can navigate the complexities of financial management with greater agility and insight.
Best Practices for Implementation
Implementing accounts payable predictive analytics is not merely a technical exercise; it’s a fundamental shift in financial management strategy. By embracing best practices in this domain, organizations can effectively harness the insights generated from their data, reduce inefficiencies, and bolster decision-making processes. Let’s delve into some essential elements that shape successful implementation.
Data Collection and Preparation
The bedrock of any predictive analytics initiative rests on the quality of data harvested. Collecting and preparing the right data is where the journey begins.
First off, organizations must ensure that their data sources are not just numerous but relevant too. This could include invoices, payment histories, vendor contracts, and even external data like market trends. Accurate, complete, and appropriately structured data lays a strong foundation for predictive models.
Employing data cleansing techniques cannot be overstated. This involves identifying errors or inconsistencies in the data, such as duplicate entries or outdated vendor information, which can lead to inaccuracies in modeling. Clean data fosters reliable algorithms, which are, in essence, the engines driving predictive insights.
Here are several steps for effective data collection and preparation:
- Identify Key Data Sources: Understand where your relevant data lies, from internal systems like ERP software to external sources like regulatory data.
- Standardize Data Formats: Ensure all data is logged in a consistent format. This not only makes it easier to analyze but also prevents confusion down the line.
- Periodic Audits: Regular checks on data integrity and completeness go a long way to maintaining healthy data flows.
Engaging Stakeholders and Training Teams
The human element is often the most overlooked aspect when implementing predictive analytics. Successfully leveraging analytics tools requires not just data but also the buy-in and skillset of key stakeholders.
Fostering an inclusive environment starts with identifying all relevant stakeholders across departments—finance, IT, and operations. Each group plays a critical role, and their insights can help shape a more effective analytics strategy.
Engagement can happen through workshops and brainstorming sessions to demystify predictive analytics and highlight its potential benefits. Educational initiatives can address concerns and resistance, shifting perceptions to view analytics as an ally for their daily operations.
Training teams on the specifics of the predictive tools being implemented is imperative. This could involve:
- Hands-on Workshops: Allow team members to explore the analytics tools firsthand, enhancing their comfort and confidence.
- Onboarding Programs: Prepare tailored onboarding materials for new hires which can introduce predictive tools and their applications in the organization.
- Continuous Learning: Keep the dialogue open with periodic refresher courses to keep data handling skills sharp.
"The world of predictive analytics is evolving, and by integrating stakeholder engagement and training, organizations position themselves ahead of the curve."
In summary, best practices in implementing accounts payable predictive analytics hinge on meticulous data collection and the strategic engagement of stakeholders. As organizations navigate this sophisticated landscape, making informed decisions is pivotal. Think of it as building a house; a solid structure relies on both robust foundations and the right skilled architects.
Finale and Recommendations
The concluding section of this article on accounts payable predictive analytics is pivotal. It encapsulates the insights gleaned throughout, offering clarity on how predictive analytics can redefine financial management within businesses of various sizes. Embracing this paradigm shift leads to enhanced decision-making, better vendor relations, and ultimately, a healthier bottom line. The crux of the discussion revolves around leveraging data intelligently to not only understand past performance but to anticipate future trends.
"Data drives insights. Insights shape actions. Actions generate value."
Summary of Key Insights
- Critical Role of Predictive Analytics: Companies leveraging predictive analytics in accounts payable stand on firmer ground. It informs cash flow management more reliably and helps mitigate risks associated with late payments or vendor issues.
- Types of Data Sources: Kickstarting predictive analytics requires understanding where data comes from—enterprise resource planning (ERP) systems, purchase orders, vendor invoices, and payment histories are just a few crucial touchpoints.
- Implementation Challenges: Addressing data quality and consistency issues is paramount for success. Organizations must also be prepared for potential cultural resistance to changing established processes. However, the long-term reward outweighs the initial resistance.
- Tools and Technologies: Familiarity with available software solutions and emerging technologies like AI can offer a significant advantage. Deploying robust tools can make a notable difference in efficiency.
- Future of Financial Management: It’s not just about adopting technology; it’s about adapting to trends. Automation and AI are shaping the future of accounts payable, making it easier to handle complex tasks swiftly.
Next Steps for Organizations
Moving forward, organizations eager to improve their accounts payable processes through predictive analytics should consider the following steps:
- Assess Current Data Practices: Take stock of existing data practices to identify gaps and areas for improvement.
- Invest in Training: Empower employees through training sessions focused on the use of predictive analytics tools and methodologies. When teams know how to utilize data effectively, the benefits multiply.
- Pilot Programs: Before full implementation, organizations might want to launch pilot programs within selected departments. This approach allows for the refining of processes before widescale rollout.
- Create a Feedback Loop: Developing feedback mechanisms to assess the effectiveness of predictive analytics can enable ongoing improvements.
- Stay Informed: Keep up with the advancements in predictive analytics technologies. Engaging with industry publications and conferences can be invaluable.
In summary, accounts payable predictive analytics is more than a buzzword; it is a crucial component for advancing financial management practices. By understanding the insights and following the recommended steps, organizations can enhance their competitiveness and achieve their financial goals.