Master Data Management in Banking: Key Insights
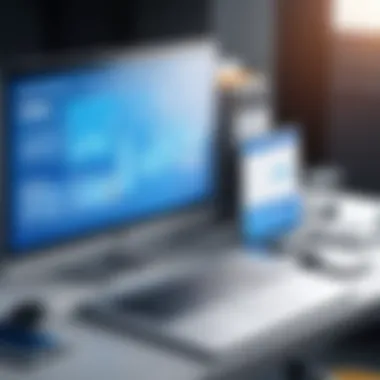
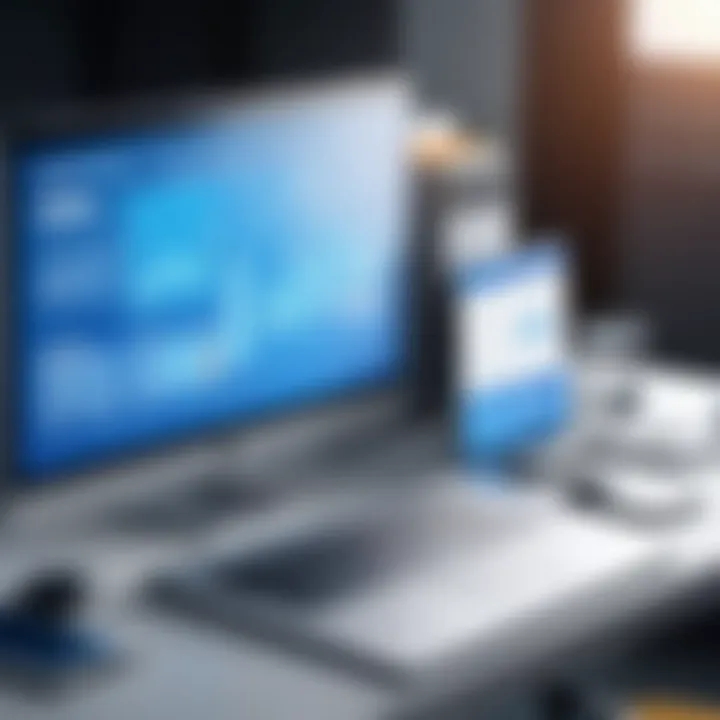
Intro
In today's banking environment, where information flows at lightning speed and the demand for accuracy is paramount, Master Data Management (MDM) plays a pivotal role. Amidst the myriad of transactions, regulatory requirements, and customer interactions, the necessity for a single source of truth cannot be overstated. MDM serves as this reliable foundation, ensuring that all data across different domains are synchronized and consistent.
The goal of this article is to facilitate a nuanced understanding of master data management within banks. From recognizing the challenges banks face in data acquisition to illustrating best practices through case studies, we will cover it all comprehensively. This understanding is not only beneficial for IT and data professionals but also for decision-makers in small and large financial institutions. They all need to understand how proper data management can drive operational efficiency and ultimately elevate customer service.
Master Data Management is not merely about data storage or processing; it’s about thoughtful integration and governance of data assets. It’s the cornerstone that supports properly functioning banking systems, ensuring that data is reliable and trustworthy.
Understanding Master Data Management
Understanding Master Data Management (MDM) is crucial for anyone in the banking industry aiming to enhance operational efficiency and data integrity. MDM serves as the backbone for effective data governance, facilitating accurate, consistent, and reliable information across the institution.
Definition of Master Data Management
Master Data Management involves processes and technologies that ensure corporate data is accurate and consistent across various systems. At its core, MDM identifies key business entities, such as customers, accounts, and products, consolidating these data sources into a single, reliable version of the truth. This definition highlights the discipline's focus on improving data usability, facilitating better decision-making, and ensuring the bank operates with the most up-to-date information.
Key Components of
Several components are essential to a successful MDM approach. Each plays a unique role in ensuring that data remains a valuable asset rather than a persistent problem.
Data Governance
Data governance is the structure that guides data management processes. It establishes policies and standards, ensures compliance with regulations, and delineates roles and responsibilities around data usage. A solid data governance framework can be a game-changer, enabling banks to trust their data while reducing the risk of errors and compliance issues. One key characteristic of Data Governance is accountability. It ensures that all data owners are aware of their responsibilities when it comes to data accuracy and security. This creates a culture of care around data, benefitting the overall data management strategy. However, its unique feature, such as requiring ongoing revisions to adapt to business changes, can pose challenges in adoption.
Data Quality
Data quality focuses on the condition of data, concerning accuracy, completeness, and reliability. Ensuring that this data meets high standards is fundamental, as faulty information can lead to flawed financial decisions and a loss of customer trust. A notable characteristic of Data Quality is the need for continuous monitoring and improvement. In banking, where data often originates from various channels, maintaining a consistent quality can be tougher than it seems. One advantage of prioritizing data quality is that it helps banks build a better reputation with customers, leading to long-term relationships. However, maintaining high data quality may require significant investment in technology and training.
Data Integration
Data integration involves combining data from disparate sources to provide a uniform view of information. This is especially significant in banking, where numerous systems may hold different parts of customer or transaction information. The key characteristic of Data Integration is its ability to aggregate and harmonize data into a comprehensible format for users. MDM's focus on integration allows banks to break down data silos and furnish comprehensive insights. Yet, one downside is that integrating legacy systems can often encounter hurdles that require thoughtful management.
Data Lifecycle Management
Data Lifecycle Management refers to the policies and procedures governing data from creation to deletion. It ensures data is appropriately classified and retains its relevance throughout its lifecycle. A major benefit of implementing effective Data Lifecycle Management is that it enables banks to optimize their storage costs, reducing the waste associated with unnecessary data retention. Moreover, this component supports compliance by ensuring that data is preserved and disposed of according to policy. The challenge, however, often lies in balancing the costs of maintaining extensive data versus the need for quick access to vital information.
Significance of in the Banking Sector
The significance of MDM in the banking sector cannot be overstated. It enhances operational efficiency by streamlining processes and reducing duplication. In a world where accurate data can drive a competitive edge, MDM allows banks to confidently understand their customer base and refine their strategies accordingly. As compliance regulations become increasingly stringent, MDM systems are also indispensable in managing risk and adhering to necessary laws. In summary, the incorporation of MDM is not merely beneficial; it is essential for modern banking that aspires to compete effectively in an increasingly data-driven landscape.
The Role of Data in Banking
Data isn't just the undercurrent of banking; it's the lifeblood that fuels all operations, decision-making processes, and customer interactions. In the banking sector, the role of data is profound and multifaceted. From streamlining operations to enhancing customer service and ensuring compliance, understanding the various types of data and the challenges surrounding their management is essential. This section sheds light on why data plays such a pivotal role in banking, examining specific data types and the challenges faced while handling them.
Types of Data in Banking
In the banking realm, data can generally be categorized into three main types: Customer Data, Transaction Data, and Regulatory Data. Each of these data types serves a unique purpose and reflects different aspects of banking operations.
Customer Data
Customer data encompasses information regarding the bank's clientele including their demographics, preferences, and activity patterns. It's essential for personalized services and targeted marketing. A key characteristic of customer data is its dynamic nature; it evolves as customer behaviors and preferences shift. This makes it a valuable yet challenging aspect to manage.
One standout feature of customer data is its ability to provide insights into customer needs, thus enabling the bank to tailor its products and services accordingly. For instance, by analyzing how often a customer uses their credit card, a bank can offer customized rewards or alerts. However, the downside lies in maintaining data accuracy and privacy. If customer data isn't kept up-to-date, it can lead to missed opportunities or compliance breaches.
Transaction Data
Transaction data refers to records of all transactions executed by clients, from deposits to withdrawals and transfers. This data is crucial for monitoring daily banking functions as well as for fraud detection. What sets transaction data apart is its sheer volume and the speed at which it is generated. Banks deal with millions of transactions daily, making real-time analytics a necessity.
The primary advantage of transaction data is its usefulness in detecting patterns and anomalies. This feature allows banks to identify potentially fraudulent activities or to optimize operational efficiencies. However, the complexity of managing large volumes of transaction data can pose a challenge, especially in ensuring that data integrity is preserved across various systems.
Regulatory Data
Regulatory data includes information required to ensure compliance with laws and regulations governing the banking industry. This type is critical as it dictates how banks operate, conduct audits, and submit reports to authorities. The key characteristic of regulatory data is its rigid structure; it often must conform to certain formats or standards imposed by governing bodies.
One unique feature of regulatory data is that it serves to safeguard not just the institution, but also the customers' interests. By adhering to compliance requirements based on this data, banks can mitigate risks. On the flip side, the challenge with regulatory data lies in its often bureaucratic handling. Keeping it accurate and accessible across various departments can be a Herculean task.
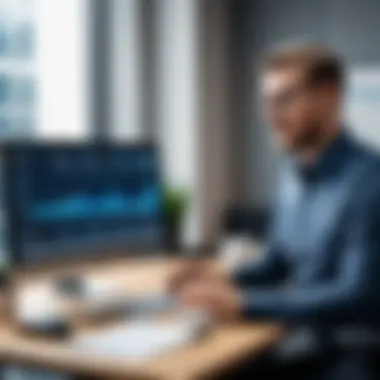
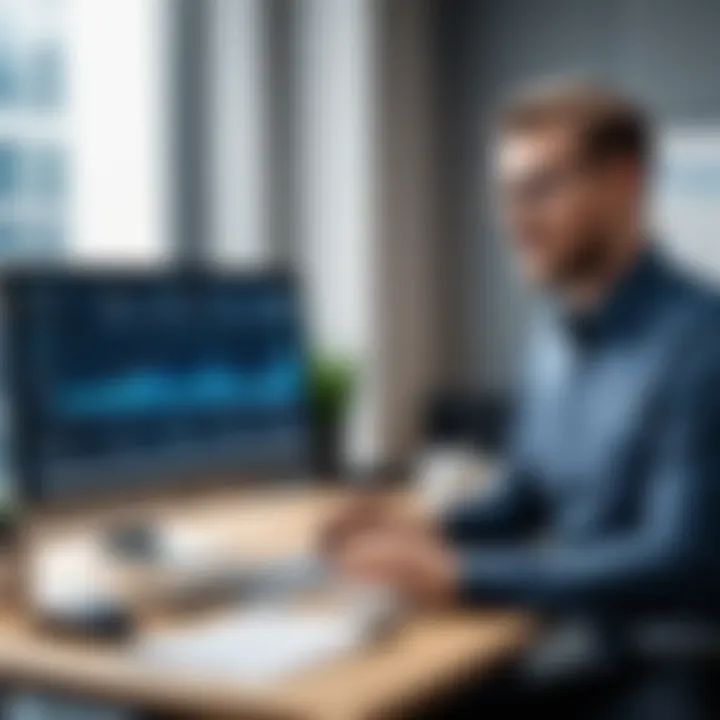
Challenges with Data Management
The effective management of data also presents several challenges that banks must contend with. The presence of data silos, inconsistent data, and the necessity for regulatory compliance are three major hurdles that financial institutions face today.
Data Silos
Data silos refer to isolated pockets of data that hinder comprehensive analysis across an organization. In a bank, different departments may keep their own versions of data, leading to inconsistencies. This characteristic is both a flaw and a common occurrence in banking setups. The advantage of having specific departmental data is apparent in task-focused analysis, but it also creates hurdles. Siloed data can lead to duplication, fragmented insights, and ultimately, poorer decision-making.
The unique threat of data silos is that they limit the bank’s holistic view of its operations. Without a unified approach to data, providing seamless customer experiences and effective risk management becomes challenging.
Inconsistent Data
Inconsistent data arises when different sources produce varying versions of the same information. This can be particularly damaging for banks that rely on data for compliance, analytics, and customer service. A key characteristic of inconsistent data is its unpredictability; it can emerge due to human errors, system failures, or lack of standardized procedures.
The advantage of having multiple data sources is that it can enrich the overall information set. However, without reconciling inconsistencies, operational efficiency is jeopardized. When data discrepancies erect barriers to accurate reporting or analysis, it can lead to mistrust among stakeholders.
Regulatory Compliance
Regulatory compliance involves adhering to a multitude of laws and standards governing the banking industry. The key characteristic of regulatory compliance is that it evolves constantly; banks must keep abreast of changes to stay compliant. It's beneficial in establishing trust and safety for clients but daunting in the continuously shifting landscape.
The unique feature of regulatory compliance is that it not only safeguards the bank but protects consumers from risks. Nevertheless, the challenge lies in ensuring comprehensive data coverage that meets compliance requirements, which often demands intricate systems and extensive resources.
"Effective data management is more than just maintaining integrity; it’s about fostering an environment where reliable insights can flourish."
In summary, data plays an irreplaceable role in the banking sector. By understanding the various types of data and the challenges faced in managing them, banks can create effective strategies to harness data's full potential for operational efficiency and enhanced customer service.
End
Grasping the significance of data types and the challenges linked to their management lays the foundation for a robust Master Data Management framework in banking. By addressing the nuances of each data type, financial institutions can better navigate the complexities of data management and drive positive outcomes.
Implementing Master Data Management in Banks
Implementing Master Data Management (MDM) in banks is no small feat, but its necessity can't be overstated. MDM acts as the backbone to an institution's data strategy. In an industry where precision and trust are paramount, the successful application of MDM can bolster operational effectiveness and improve customer relations. This section outlines the strategic importance of embarking on an MDM journey, focusing on the hurdles that need navigation, the processes involved, and the ultimate benefits attained.
Developing a Business Case for
Building a sound business case for MDM in a banking context goes beyond just understanding the technicalities. It encapsulates identifying the pain points within current data management practices. One needs to acknowledge the disparities in data quality and the consequent effects on decision-making. By presenting a compelling argument captured through data-centric analytics, stakeholders can be convinced of the necessity for MDM. Not only does this justify investment, but it also paves the way for support throughout the implementation.
Strategic Planning for Implementation
Stakeholder Engagement
Engaging stakeholders effectively is crucial for MDM implementation. This aspect not only involves gaining support but also ensuring everyone's perspectives are taken into account. The key characteristic of stakeholder engagement is transparency. Eyes should be kept on the goal, and that requires open communication around what MDM can bring to the table. Many top-tier banks have turned their implementation into a team effort, thus making the MDM journey a shared responsibility. One advantage of such engagement is that it cultivates a culture of accountability and commitment among team members, although it can sometimes slow down the process due to the need for continuous dialogue.
Resource Allocation
Resource allocation is another critical element in the successful rollout of MDM strategies. This includes the distribution of financial, human, and technological resources. The primary consideration here has to be a balanced approach—ensuring there are enough resources without breaking the budget. A beneficial facet of sound resource allocation is that it allows for a tailor-made solution to specific banking needs. However, if done poorly, it could lead to either overcapacity or, conversely, a lack of essential skills within the team, which hampers the entire initiative.
Timeline Development
Establishing a clear timeline for MDM implementation shakes hands closely with strategic planning. A well-drafted timeline outlines project milestones and court dates ahead of time. By setting realistic deadlines and adhering to them, banks can maintain a clear focus, minimizing delays in the overall program. Notably, a unique feature of effective timeline development is the iterative approach; project phases can be broken down into manageable tasks. While it creates a clear pathway forward, challenges may arise in keeping the momentum when intermediate timelines are missed.
Choosing the Right Solution
Choosing an appropriate MDM solution is pivotal in the success of MDM endeavors. With numerous vendors in the market, banks must navigate a labyrinth of choices to find the right fit for their specific needs.
Evaluating Vendors
Vendor evaluation plays a vital role in the MDM selection process. It requires not just a checklist of features but also an understanding of how a vendor’s solution aligns with the bank's philosophy and goals. The key characteristic of effective vendor evaluation is adaptability. A vendor that can evolve with changing market dynamics could be invaluable. However, it’s important to evaluate the long-term viability of your chosen partner, as a bad choice could lead to further complications down the road.
Cost Consideration
Cost considerations cannot be overlooked. Pricing models vary greatly between MDM solutions, and their impact on the overall budget is significant. What’s beneficial is understanding the total cost of ownership rather than just the upfront expenses. This includes maintenance, scalability, and potential integration with existing systems. A careful balance is needed here; while one could cut back on the budget, this may lead to long-term trade-offs that can be detrimental.
Scalability Requirements
Finally, evaluating scalability requirements is essential. MDM must cater not just to current needs but also to future growth projections. The key characteristic of effective scalability is flexibility. Solutions that can be easily adapted or expanded without significant reinvestment offer a substantial advantage. This aspect often dictates whether an MDM initiative stands the test of time or withers away due to inability to cope with growth.

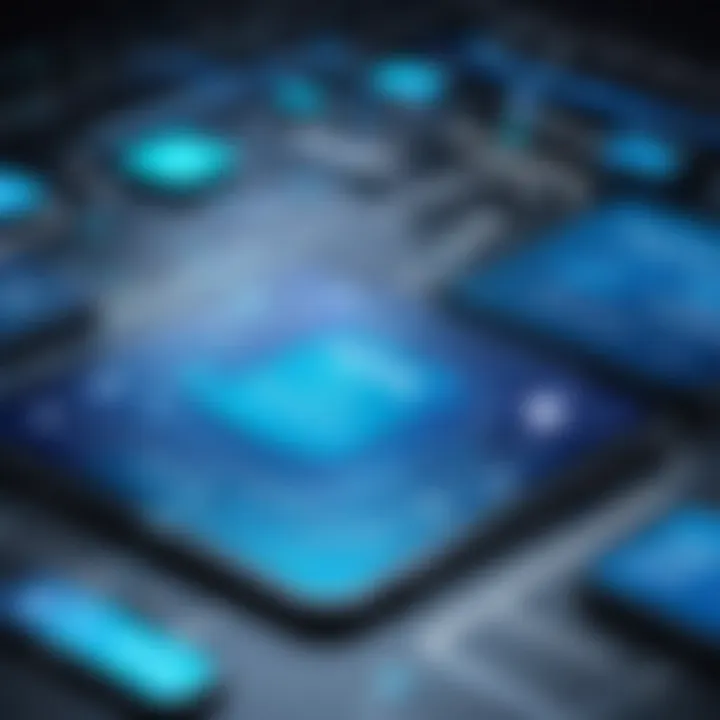
As we delve deeper into the implementation phase of MDM, it becomes clear that making informed decisions around these central themes will lead to greater satisfaction down the line. The upfront effort pays off significantly as banks navigate this complex yet rewarding landscape.
Best Practices in for Banking
Best Practices in Master Data Management (MDM) are the bedrock on which efficient data handling is built in banking. To navigate a landscape brimming with regulatory scrutiny, complex customer needs, and massive datasets, banks must lean into best practices. These practices not only help in streamlining operations but also ensure compliance and enhance customer experience.
A core aspect of these best practices is data governance frameworks. Clear governance structures lay out who can do what with data, helping mitigate risks associated with data misuse or breaches. An effective data governance framework doesn’t just focus on compliance; it creates a culture of accountability and transparency. After all, when everyone knows the rules of the road, the journey is smoother.
Data Governance Frameworks
In a banking context, data governance frameworks serve as essential guides. They provide a structured approach to managing the data lifecycle, helping ensure that data remains accurate, accessible, and secure.
- Clarity of Roles: One of the main characteristics of a good governance framework is the clear definition of roles and responsibilities. When all employees understand their data-related duties, the likelihood of errors decreases significantly.
- Regulatory Compliance: Another critical feature of governance frameworks is their alignment with legal requirements. Financial institutions operate under intense scrutiny. Therefore, incorporating compliance into governance ensures that the bank's practices are not only efficient but also legal.
- Continuous Improvement: Good governance is not static; it evolves. A unique characteristic of robust governance practices is their focus on ongoing enhancements, making them adaptable to new legal requirements and changing business landscapes.
Ensuring Data Quality
At the heart of effective MDM lies the assurance of data quality. Poor data quality can lead to a myriad of issues, making it imperative that banks implement stringent quality controls.
Data Profiling
Data profiling involves examining data for quality assessment. This process allows banks to understand their data’s characteristics better, spotting inconsistencies and anomalies before they create problems.
- Key Characteristic: The key strength of data profiling is its ability to provide insights into the data's current state. This insight enables informed decision-making.
- Beneficial Choice: Its importance in identifying data quality issues early on makes data profiling a wise choice for this article.
- Unique Feature: One unique feature of data profiling is its capacity to automate detection of data anomalies, freeing up human resources for more strategic tasks. However, reliance on automated tools can sometimes overlook nuanced human insights.
Data Cleansing
Data cleansing is the process of correcting or removing corrupt data. It's a crucial component of ensuring data integrity.
- Core Role: A pivotal aspect of data cleansing is ensuring that only accurate and relevant data enters the system.
- Well-Chosen Method: Given its importance in maintaining data reliability, it stands as a beneficial strategy in our exploration.
- Unique Feature: This process often includes deduplication and correction procedures, maximizing the value derived from data. Yet, constant cleansing can become resource-intensive and require ongoing human oversight.
Data Validation
Data validation checks the accuracy and quality of data before it gets used. It helps identify whether the data meets certain requirements.
- Purpose: The main purpose of data validation is to ensure that data is both accurate and appropriate for its intended use.
- Popular Choice: Its role in minimizing erroneous data entry makes data validation a popular practice in this narrative.
- Unique Feature: Data validation processes are often integrated within data entry applications, catching errors at the source. Nevertheless, their reliance on correct initial input means that without user diligence, errors could slip through.
Ongoing Data Maintenance Strategies
Maintaining data health is an ongoing endeavor. Banks should not just fix data issues; they should continuously seek to manage and monitor data effectively.
Monitoring Processes
Monitoring processes are integral to ensuring long-term data health. These systems track data through its lifecycle, identifying issues as they arise.
- Key Trait: A pivotal trait of robust monitoring is its ability to provide real-time insights, allowing for swift corrective actions.
- Significance: Regular monitoring is beneficial because it keeps data relevant and reliable.
- Unique Feature: Utilizing machine learning in monitoring can enhance the effectiveness of these processes. Yet, reliance on automated systems needs careful oversight to prevent blindness to emerging issues.
Feedback Mechanisms
Feedback mechanisms facilitate the collection of user input regarding the effectiveness of data operations.
- Core Functionality: Their core function lies in identifying gaps in data management processes and offering improvement opportunities.
- Wisdom in Practice: Making feedback mechanisms an embedded part of data systems ensures continuous growth and informed decision-making benefits this article.
- Unique Aspect: By gathering user experiences and outcomes, banks can adopt a more user-centered approach. However, implementing feedback systems requires a commitment to action; gathering data means little without meaningful application.
In summary, the best practices in MDM for banking, from solid governance frameworks to rigourous quality assurance and proactive maintenance strategies, enable financial institutions to stay competitive. These practices support effective operations while cultivating trust in their data-driven decisions.
Case Studies of in Banking
Case studies provide real-world insights into how Master Data Management (MDM) is effectively applied in the banking sector. They serve to bridge the gap between theoretical concepts and their practical application, showcasing both successes and failures. By examining these case studies, banks can draw valuable lessons that inform their own MDM strategies. Moreover, these examples highlight the tangible benefits of robust MDM systems, such as improved data quality, enhanced regulatory compliance, and superior customer experiences.
A Large Global Bank Implementation
Challenges Faced
In the journey of implementing MDM, large global banks often encounter significant challenges. One of the most pressing issues faced by a prominent bank was data silos. These silos exist when different departments within the bank store and manage data independently, leading to fragmentation and inconsistency. The critical characteristic of this challenge is its pervasive nature. It not only hampers effective data access but also obstructs holistic decision-making that relies on unified data sources.
The unique feature of this challenge is how it manifests across various levels. For instance, marketing might have a different set of customer information than compliance departments, creating discrepancies that affect business operations. Understanding and overcoming the threat of data silos is essential for improving data integrity in any MDM initiative.
Solutions Adopted
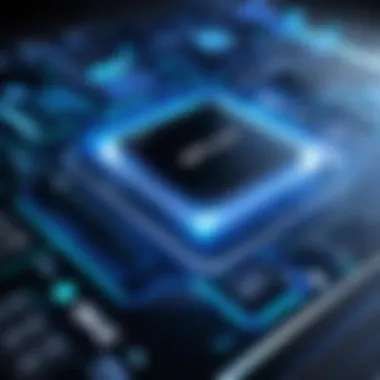
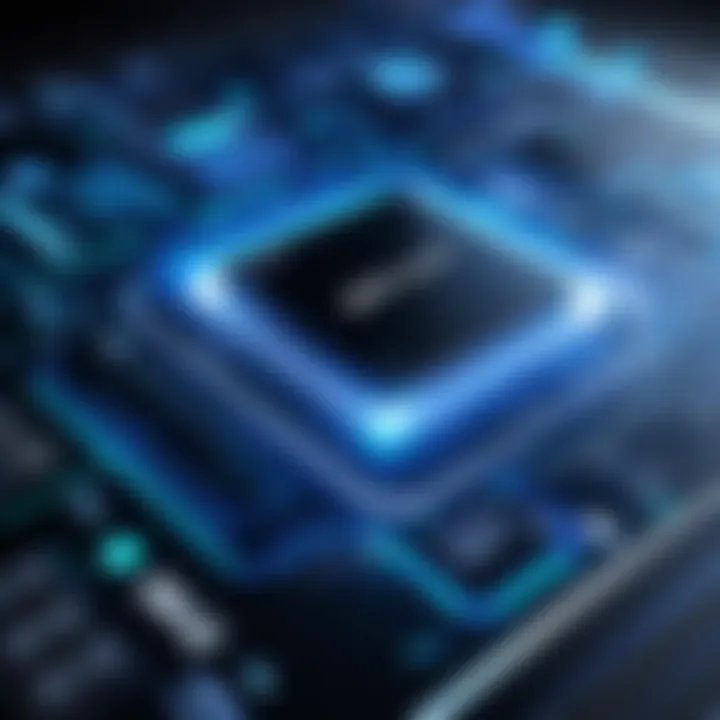
To counter these challenges, the global bank adopted a comprehensive data governance strategy. This approach involved forming cross-departmental teams aimed at creating a unified view of data. This method is beneficial as it directly addresses the root of data silos by ensuring consistent data definitions across the organization. The distinctive aspect of this solution is its emphasis on collaboration, which fosters a culture of shared responsibility towards data accuracy.
What stands out as an advantage here is the facility of seamless data integration across departments. However, it's worth noting that this solution required significant time investment and a cultural shift within the organization. Balancing these demands while maintaining everyday operations was a delicate task.
Results Achieved
Implementing the data governance strategy led to notable improvements in data accuracy and faster decision-making processes. The critical outcome was that the global bank enhanced its ability to comply with regulatory requirements, which was crucial in the highly regulated banking environment. Furthermore, the unified data view allowed the bank to improve customer service by leveraging comprehensive customer profiles for personalized offerings.
The unique feature of these results is their sustainability; the steps taken to resolve the challenges have fostered a more agile data culture. Nonetheless, while these improvements were significant, maintaining momentum and continued adaptation to new data management paradigms posed an ongoing challenge.
Implementing in a Regional Bank
Initial Setup
For a regional bank, the initial setup of MDM was a crucial phase. The bank focused on establishing a foundational framework to manage its data effectively from the get-go. This setup included identifying key data sources and setting clear objectives for data management. A primary characteristic of this initial setup was its simplicity. By starting small, the bank could gradually build upon their MDM efforts without overwhelming staff or resources.
The unique feature here was that the regional bank utilized existing tools and software solutions instead of implementing an entirely new system. This approach helped in minimizing costs and easing the transition. However, a potential disadvantage of this strategy was the need for ongoing adjustments as the system evolved to meet expanding requirements.
Key Learnings
After the initial implementation, the regional bank gained several key learnings that would guide their future MDM efforts. One pivotal realization was the importance of stakeholder involvement. Engaging with employees across departments helped the bank understand their data management needs better. This characteristic is crucial as it fosters a sense of ownership, making the data governance process more effective.
The key takeaway is that consistent communication and education are vital for the successful adoption of MDM practices. A challenge, however, is the continuous need for training sessions to keep staff updated on best practices.
Customer Impact
Ultimately, the impact of MDM implementation on customers was positive. The regional bank could now offer better services and personalized interactions due to improved access to accurate customer data. This enhanced customer experience resulted in higher customer satisfaction rates and loyalty. The defining characteristic of this impact is its long-term potential; satisfied customers are less likely to switch banks.
A significant feature is that the improved understanding of customer profiles also allowed for targeted marketing campaigns, which increased the effectiveness of their outreach. Nevertheless, while the outcomes were favorable, the bank faced the challenge of ensuring that data privacy protocols were stringent to uphold customer trust.
Future Trends in Master Data Management
The landscape of Master Data Management (MDM) in banking is set to evolve rapidly. New technologies and regulations are not just shaping how data is managed but also redefining its strategic importance. This section will explore the future trends that are poised to influence MDM significantly, examining the integration of AI and machine learning, the impact of regulatory changes, and the adaptation to digital transformation.
Integration of AI and Machine Learning
Artificial intelligence and machine learning are creating waves in data management. Banks can now leverage these technologies to automate data processing and improve accuracy. For instance, machine learning algorithms can analyze vast datasets swiftly, helping in identifying patterns and anomalies that might have gone unnoticed. This predictive capability can assist in credit assessments or risk management, allowing for smarter decisions and more effective customer service.
One key advantage of integrating AI into MDM processes is the enhancement of data quality. Algorithms can monitor data consistency and detect errors in real-time, ensuring that the data accessed across the organization is accurate and reliable.
Moreover, AI tools can also allow banks to personalize services. By analyzing customer behavior data, banks can tailor offerings which increases customer satisfaction and loyalty. Therefore, AI and machine learning empower banks not just to manage data, but to craft meaningful customer experiences based on solid data foundations.
Impact of Regulatory Changes
With constantly shifting regulatory frameworks, banks must remain agile and adaptable. Regulatory compliance has always been a key aspect of data management for banks, but future trends indicate that upcoming regulations will demand even stricter controls regarding data handling and storage. For example, the implementation of the General Data Protection Regulation (GDPR) has set a precedent for rigorous data protection laws.
As regulations evolve, banks will need to fine-tune their MDM strategies to ensure compliance. This involves adopting robust governance frameworks that outline clear policies and procedures for data usage.
Additionally, the trends suggest that organizations might need to invest in technologies that facilitate compliance reporting. By implementing automated solutions that capture and compile necessary compliance-related data, banks can minimize the risk of non-compliance penalties, ensuring both security and integrity in operations.
Adaptation to Digital Transformation
Digital transformation is no longer optional in the banking sector; it’s a necessity. Customers expect seamless, omnichannel experiences. Here is where MDM plays a crucial role. As banks transition towards more digital and mobile-based services, having a unified view of data becomes paramount.
Multichannel data integration is essential to achieving a comprehensive customer profile. By doing so, banks can derive insights from various touchpoints, subsequently improving service delivery. This unified approach aids not only in streamlining operations but also in enhancing customer engagement strategies.
Moreover, adapting to digital transformation means embracing cloud-based MDM solutions. The agility and scalability of cloud services enable banks to adjust their MDM strategies to align with rapidly changing business requirements. This will foster innovation while also driving down operational costs.
"The integration of emerging technologies into Master Data Management not only streamlines processes but also strengthens data governance, ultimately leading to a more secure and efficient banking environment."
In summary, the future of Master Data Management in banking looks promising yet challenging. Embracing advancements in AI and machine learning, staying ahead of regulatory changes, and adapting to digital transformation are essential for banks seeking to maintain their competitive edge. As these trends continue to unfold, banks must equip themselves with the right tools and strategies to harness the power of data effectively.
End
Master Data Management (MDM) holds a crucial position in the banking industry, weaving a strong thread of accuracy and coherence into the fabric of operations. As banks wrestle with the diversification of data coming from various sources, the significance of MDM cannot be overstated. By leveraging MDM, financial institutions can streamline how they handle their data, ensuring it remains consistent, timely, and relevant.
Recap of Importance in Banking
In the realm of banking, where customer trust and regulatory compliance are paramount, the stakes are incredibly high. The implementation of MDM is central to overcoming the hurdles banks face with data management. Relying on a single trusted source of data allows institutions to mitigate risks tied to data inconsistencies and facilitates improving overall data quality. Here are some key points to consider:
- Enhancing Customer Experiences: Customers expect seamless experiences across various channels. MDM enables a holistic view of customer data, paving the path for tailored services that boost satisfaction.
- Regulatory Compliance: Keeping up with regulatory demands is a tall order, but MDM assists in maintaining accuracy and completeness of data. This not only helps avoid hefty fines but also boosts the bank's reputation.
- Operational Efficiency: By centralizing core data, banks can eliminate redundancies and improve productivity. This leads to better decision-making as management has access to accurate, relevant data.
"Without high-quality data, banks risk failing to meet customer expectations as well as regulatory requirements, jeopardizing their very existence."