Speech Recognition Technologies: Evolution and Impact
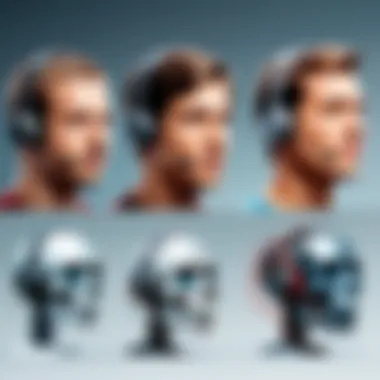
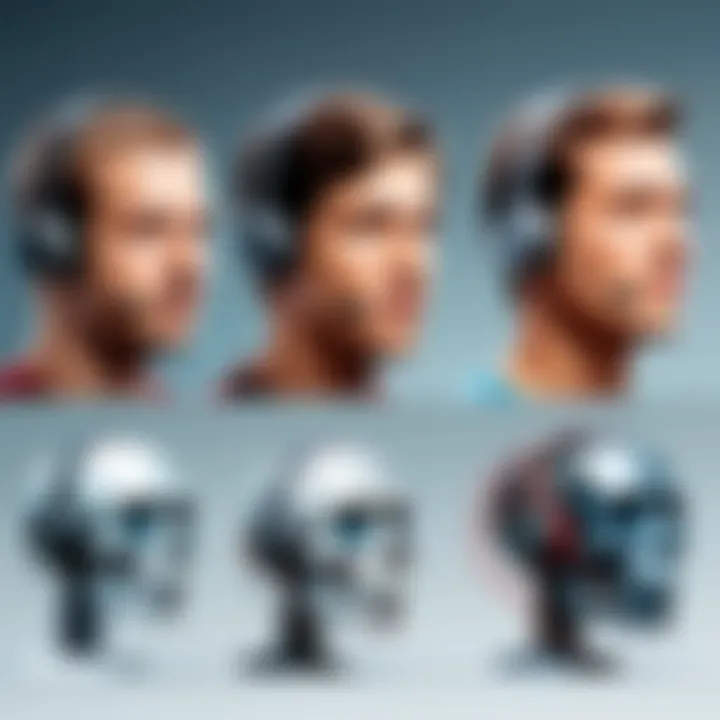
Intro
Speech recognition technology has seen remarkable progress since its inception. Initially seen as a novelty, it has evolved into an indispensable tool across various industries. From automated customer service to personal virtual assistants, the applications are vast and growing. As we delve into the facets of this technology, we aim to illuminate its significance, current capabilities, and the ethical discussions it prompts while simultaneously spotting its future trajectory.
To fully grasp how far speech recognition has come, it's essential to examine its evolution, understand its core technologies, and explore its practical uses and challenges. These understandings will help professionals from various sectors navigate the intricate landscape of speech recognition and appreciate its potential.
"The rise of conversational interfaces has prompted a deeper examination of speech recognition as a multifaceted technology that impacts user experience and functionality across platforms."
Brief Description
Overview of the Software
Speech recognition software enables computers to understand and process spoken language. It translates voice into text, allowing for hands-free control and automation of tasks. Companies like Nuance and Google have pioneered these technologies, introducing systems that harness deep learning, natural language processing, and sophisticated algorithms to achieve high accuracy. This technology ranges from simple voice-activated commands to complex natural interactions that can understand context and user intent.
Key Features and Functionalities
The main features of modern speech recognition technologies include:
- Voice Commands: Enable users to control devices or services through speech, enhancing user interaction.
- Real-Time Transcription: Offers instant conversion of spoken words into text, beneficial for meetings and lectures.
- Contextual Understanding: Some systems leverage machine learning to improve understanding based on context, providing more accurate responses.
- Multi-Language Support: Many platforms can handle various languages and dialects, making them accessible globally.
These cutting-edge functionalities see applications extending from corporate settings to healthcare, education, and customer service.
System Requirements
Hardware Requirements
Deploying speech recognition software often necessitates specific hardware specifications to ensure efficient performance:
- Microphone Quality: A noise-canceling microphone is vital for clear input.
- Processor: A quad-core processor or better is recommended to handle real-time processing.
- RAM: At least 8GB of RAM is standard for smooth operation, though 16GB is ideal for more intensive use.
Software Compatibility
Compatibility varies between different solutions, but typical requirements include:
- Operating System: Most major platforms support Windows and macOS. Linux compatibility varies, depending on the software.
- Updates: Regular updates are essential for maintaining performance and security, ensuring users have access to the latest features and improvements.
The intersection of solid hardware and compatible software creates an optimal environment for utilizing advanced speech recognition technologies effectively.
Preamble to Speech Recognition
Understanding speech recognition is like deciphering a new language that bridges humans and machines. This technology allows computers to understand spoken language, turning voice commands into actions. In this age of information where speed is crucial, speech recognition opens the door to seamless interaction. Think about it—navigating devices and applications has become more intuitive, removing the barriers of typing and clicking. The benefits stretch far and wide across diverse sectors, making it an essential focus for businesses, developers, and technology enthusiasts alike.
Defining Speech Recognition
At its core, speech recognition is the capability of a machine or program to identify words spoken aloud and convert them into text. This process involves several complex systems working in unison, including signal processing, linguistic modeling, and machine learning. You could say it’s the intersection of linguistics and engineering. When you speak into a device, it's essentially an intricate dance of algorithms analyzing the sound waves produced, identifying patterns, and matching them to a database of known language.
Importance in Modern Society
The relevance of speech recognition in today’s world cannot be overstated.
- Integration into Daily Life: With the rise of smart assistants like Google's Assistant and Amazon's Alexa, speaking to devices has become second nature. Saying, "Hey Siri, set a reminder," isn't just a command; it's a lifestyle choice that illustrates our dependency on technology.
- Accessibility: For individuals with disabilities, speech recognition technology can be a game-changer. It opens possibilities that were previously unimaginable, allowing users to navigate the digital realm hands-free.
- Efficiency in the Workplace: Think about how much quicker a person can draft an email or generate a report simply by speaking. This not only boosts productivity but also enables multitasking, which is invaluable in fast-paced business environments.
"Speech recognition technology has shifted from being a luxury into a necessity, reflecting our desire for speed and efficiency."
Historical Context
Understanding the historical context of speech recognition technology is vital for grasping its present application and future potential. The evolutionary path of this technology reflects not just scientific advancement, but also cultural shifts in how we interact with machines. Over the decades, the landscape has transformed significantly, driven by key innovations and a growing recognition of the technology's value across different industries.
Early Developments
The roots of speech recognition can be traced back to the early 20th century. One of the first systems was developed in the 1950s, when Bell Labs created a machine called "Audrey". It could recognize digits spoken by a single voice. This was revolutionary! It showcased the basic possibilities but also highlighted limitations like voice individuality and limited vocabulary.
As decades rolled on, research in the field saw some ups and downs. The 1970s introduced the concept of Hidden Markov Models, a statistical model that began to shape the way machines processed sounds and turned them into understandable speech. During this time, scientists started to grasp the importance of acoustic properties and how they could be leveraged to enhance transcription accuracy.
In the 1980s, the introduction of continuous speech recognition systems marked a significant leap forward. Machines could now recognize several words in succession, reducing previous constraints considerably. Then came the 1990s with the advent of more sophisticated algorithms, paving the way for improved speech recognition applications.
Milestones in Technology
As the technology matured, certain milestones became pivotal in its journey. The release of IBM's ViaVoice in 1997 and Dragon NaturallySpeaking in 1999 set a new standard in voice recognition. These systems could understand natural language more fluently, making them accessible to a larger audience.
Another noteworthy advancement was the introduction of deep learning in the early 2010s. This paradigm shift not only improved accuracy rates by leaps and bounds but also shifted how developers approached speech recognition challenges. The rise of neural networks allowed systems to grasp context better, recognizing not just the words but the meaning behind them.
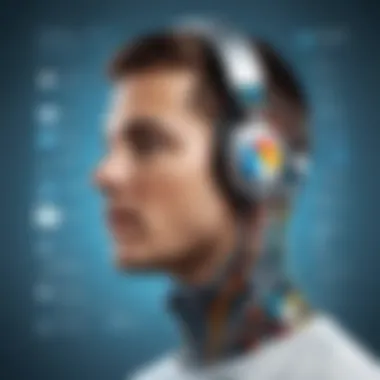
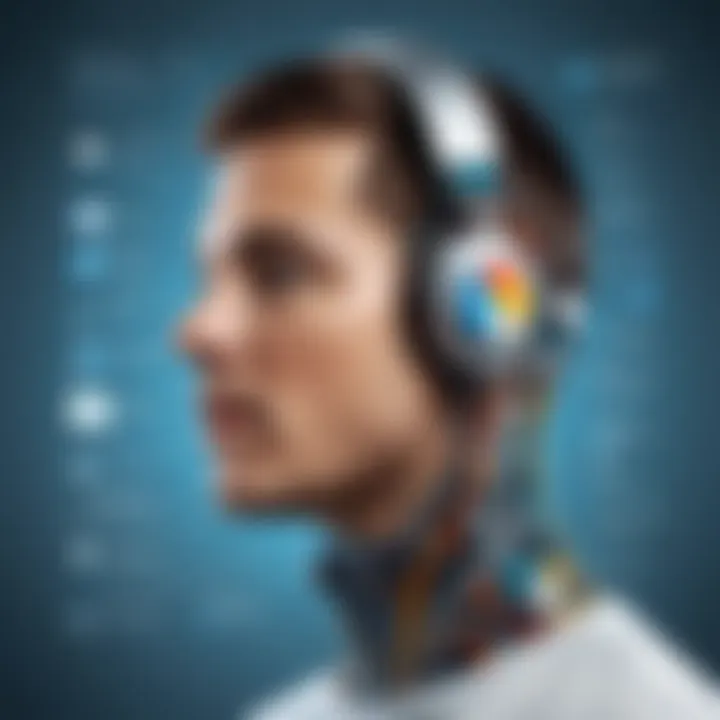
Moreover, with big data becoming more and more available, algorithms could be trained on vast amounts of diverse speech data, letting them learn from countless accents and dialects. This innovation opened new doors for applications—from voice-activated assistants to automated transcription services that we're familiar with today.
"The ability to speak to machines as if they were human has moved from sci-fi fantasy to daily reality, thanks to decades of rich research and development."
By observing these milestones, it becomes evident how each step laid the groundwork for the robust systems we see today. The evolution is not merely technical; it signifies a broader societal acceptance of technology as an integral part of our daily interactions. As we delve further into the nuances of speech recognition, provided insights will serve to frame the ongoing evolution, challenges, and future outlook of this compelling line of technology.
Key Technologies Behind Speech Recognition
The backbone of speech recognition lies in a variety of key technologies that work together seamlessly to convert spoken language into digital text. Understanding these components not only gives clarity on how the systems operate, but also highlights their unique contributions and challenges. They are foundational and serve crucial roles in the functionality of speech recognition systems. Here, we will explore three essential technologies: acoustic models, language models, and feature extraction techniques.
Acoustic Models
Acoustic models are at the heart of speech recognition systems. They are designed to understand the relationship between phonemes, the smallest units of sound, and the corresponding audio signals. Essentially, an acoustic model breaks down the audio input into smaller components, allowing the system to decipher sounds more accurately. Think of these models as the ear of the technology, processing the intricacies of sound, like inflections or tones.
These models rely on statistical representation and typically use techniques such as Hidden Markov Models (HMMs) or neural networks. HMMs continue to be popular, thanks to their strong performance in recognizing continuous speech. However, deep learning methods have made a substantial impact, allowing for more sophisticated handling of complex patterns in data.
- Benefits:
- Improved accuracy in understanding diverse accents.
- Capability to learn from vast datasets, adapting to various voices and environments.
Nonetheless, challenges remain. Acoustic models may struggle with variations in pronunciation or background noise. Moreover, the sheer amount of data required to train them can be daunting.
Language Models
Once the sounds have been interpreted, language models come into play. These models predict the probability of a sequence of words, ensuring that what’s being recognized makes logical sense. In simple terms, they help the system to predict which words should follow others based on language rules and contextual understanding.
For example, if the system hears "I want to go to the…" the language model can accurately predict "store" as a likely next word, instead of something wildly different like "elephant." This predictive capability ensures that speech recognition systems are not only accurate in terms of sound recognition but also in generating coherent text.
Language models can be categorized primarily into two types—statistical and neural. Statistical models have been widely used, but with the rise of machine learning, neural language models have gained prominence, offering superior contextual understanding and flexibility.
- Considerations:
- Ensures coherent speech output.
- Adjustments required for specific jargon or domain-specific language.
Feature Extraction Techniques
Feature extraction is another crucial component of speech recognition technology. This process involves isolating significant attributes from the raw audio signals that give vital clues about what’s being said. Features such as Mel-frequency cepstral coefficients (MFCCs) play a central role in simplifying the complexity of audio signals while retaining remarkable amounts of information.
Think of this as sifting through a clutter of voices to find the key phrases that stand out. Effective feature extraction makes it simpler for subsequent models to understand and categorize speech, thus enhancing the overall accuracy.
- Common Techniques:
- Fourier Transform: Converts signals to the frequency domain.
- Wavelet Transform: Offers time-frequency analysis.
While these techniques greatly enhance performance, they are sensitive to background noise. Sometimes, they might extract irrelevant features, which can lead to confusion or errors in recognition.
Prominent Algorithms in Speech Recognition
In the landscape of speech recognition, algorithms lay the groundwork for converting spoken language into machine-readable text. These algorithms not only enhance accuracy but also significantly influence the user experience. Understanding the different algorithms is vital for professionals, ranging from developers to business strategists, as they decide the right technology for specific applications.
The choice of algorithm determines the effectiveness of speech recognition systems. Besides accuracy, they also affect latency, processing requirements, and adaptability. By grasping the underlying principles of these algorithms, one can appreciate their impact on real-world applications—so let’s explore a few heavy hitters.
Hidden Markov Models
Hidden Markov Models (HMMs) have been a cornerstone in the realm of speech recognition for quite some time. They function on the basis of probabilities and assumptions that the sequence of spoken words can be represented as a sequence of states. To put it simply, imagine walking through a muddy field. Each step you take is influenced by where you were before, similar to how HMMs predict the next word based on previous words.
HMMs break down a speech signal into distinct components called phonemes, which are the smallest units of sound. Once these phonemes are recognized, the algorithm stitches them back together using probabilities to produce coherent output. While HMMs have been largely successful, they may struggle with variations in speech, clustered sounds, or unexpected pauses. Hence, they often serve as a solid foundation but need to work alongside others for enhanced performance.
Deep Learning Approaches
In recent years, deep learning has transcended traditional methods, offering a fresh approach that capitalizes on large datasets and powerful computation. Utilizing artificial neural networks, algorithms can learn directly from raw speech input. This can be likened to training a puppy: the more experience you let it have (in this case, data exposure), the better it learns to respond accurately.
Deep learning models, such as Convolutional Neural Networks (CNNs) and Recurrent Neural Networks (RNNs), explore complex patterns in speech data, capturing nuances that simpler models might miss. They handle variations in accent, tone, and even background noise with surprising efficacy. They make possible scenarios like real-time translation or voice-activated controls—two applications that are reshaping industries.
However, deep learning comes with its own set of challenges. Training these models require a vast amount of labeled data, and they can be computationally expensive, so organizations must weigh these considerations against the significant potential benefits.
Reinforcement Learning Techniques
Reinforcement learning (RL) introduces an intriguing twist. Unlike traditional models that learn from pre-labeled data, RL systems learn by making decisions and receiving feedback based on their actions. It's somewhat like playing a video game; the more you play, the better you get at navigating the challenges—whether that's avoiding obstacles or maximizing points.
In the context of speech recognition, RL helps enhance the responsiveness and adaptability of systems. For example, in chatbots or virtual assistants, the more they interact with users, the better they understand context and user intent, adjusting their responses accordingly. As such, RL exemplifies a significant leap toward more human-like interaction with machines.
Despite its promise, statistical rigor is crucial in reinforcement learning, as poor feedback loops can lead to less-than-ideal performance. Moreover, the convergence time—the time it takes for the algorithm to become effective—can be lengthy, necessitating substantial computational resources.
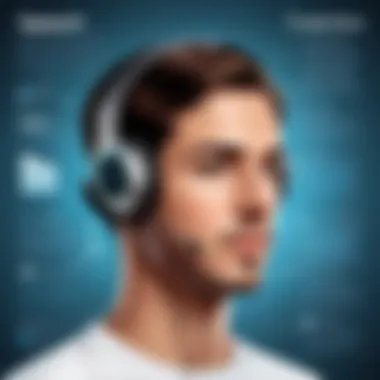
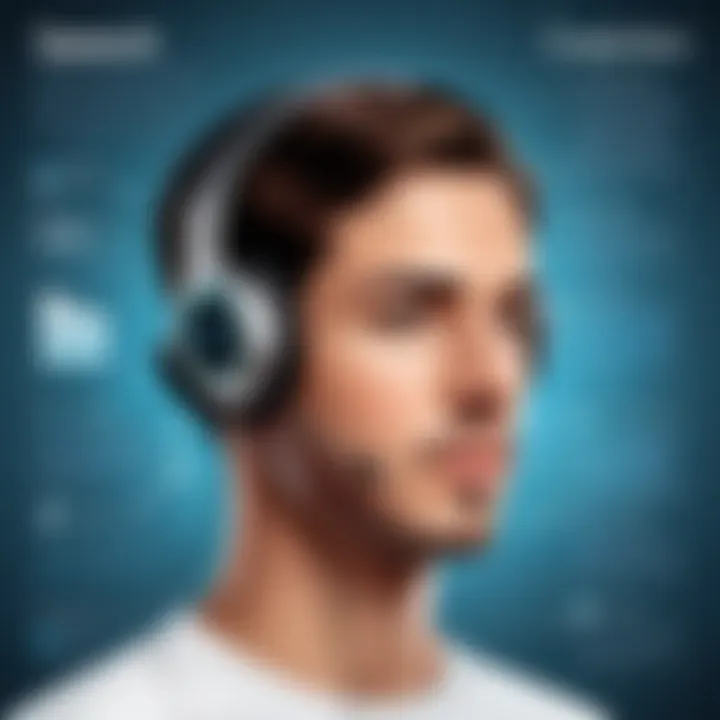
In summary, prominent algorithms like Hidden Markov Models, deep learning techniques, and reinforcement approaches play individualized roles in shaping speech recognition technologies. They each come with distinct characteristics, benefits, and challenges, enriching the technology landscape, and providing IT professionals and businesses with nuanced choices tailored to end users' needs.
Practical Applications of Speech Recognition
In today’s fast-paced digital landscape, speech recognition technology has spread its roots far and wide, becoming firmly embedded in various industries. The applications are as diverse as they are transformative. From enhancing convenience in daily tasks to revolutionizing customer interactions, the practical uses of speech recognition go beyond mere novelty—it's about creating efficiency and improving user experience.
Voice Assistants
Voice assistants like Amazon's Alexa, Apple's Siri, and Google Assistant have changed the way we navigate the digital world. They aren’t just gadgets for the tech-savvy; they've become household names. Users request everything from setting reminders to playing their favorite songs, undeterred by the complexities of the technology behind them. These assistants leverage speech recognition to interpret and respond to user queries, exhibiting remarkable flexibility.
Such systems rely on natural language processing, which aids in understanding not only words but the context around them. As a result, they are learning, adapting, and becoming more efficient at anticipating user needs. For instance, a user might simply say, "What's the weather like today?" and receive an instant reply, enabling seamless planning for their day. The integration of speech recognition in these personal assistants also leads to data-driven analytics, helping businesses improve their services based on user preferences and behaviors.
Transcription Services
Transcription services exemplify another cornerstone of speech recognition's practical applications. Manual transcription can be labor-intensive and prone to error. Tools such as Otter.ai and Rev harness the power of speech recognition to provide fast and accurate transcriptions, which has become indispensable across various fields—journalism, legal, and academic, to name a few.
With advanced algorithms to discern the nuances in dialogue, these services can transcribe conversations with minimal oversight. This not only saves time and reduces costs but helps in virtually eliminating transcription errors. Users simply upload audio files and receive clean, organized written content in return. Thus, businesses can ensure effective communication while alleviating the burden on employees tasked with meeting tight deadlines.
Customer Service Automation
The rise of speech recognition in customer service automation presents an intriguing shift from traditional methods. Chatbots and automated phone systems can handle customer inquiries without human intervention, employing voice recognition to provide quick responses and solutions.
Consider the scenario where a customer calls a support line. Instead of an immense wait time or the need to sift through endless options, speech recognition helps in identifying the customer's needs right off the bat. Visionary companies have implemented these systems, allowing them to scale up support while enhancing overall customer satisfaction. This automated service is not only cost-effective but can also operate around the clock, addressing customers in different time zones.
Accessibility Technologies
Accessibility technologies underline the important role speech recognition plays in promoting inclusivity. These technologies are vital for individuals with disabilities, as they assist in breaking down barriers. For example, speech-to-text programs provide individuals who are deaf or hard of hearing with access to verbal communication through real-time translations.
Moreover, voice recognition helps those with mobility impairments operate devices without the need for physical interaction. This can empower users to control smart home devices, send messages, or browse the internet just by talking. The continuous advancements in this domain signal that technology is indeed heading towards a more inclusive future, one where everyone can participate unconstrained by their physical limitations.
"Accessibility is not a feature, it’s a social trend that we can no longer ignore."
Through these diverse applications of speech recognition technology, it's clear that the benefits extend far beyond convenience. They embody a significant leap towards efficiency, quality service, and inclusivity. The ways in which businesses and individuals harness this technology will likely shape the future landscape of various sectors, influencing how we interact with technology on a daily basis.
User Experience in Speech Recognition
User experience (UX) in speech recognition is an essential aspect that intertwines technology with human interaction. The goal is to create systems that not only understand spoken language but also respond in a manner that feels intuitive and engaging to users. When a device can seamlessly interpret commands or questions, it fosters a sense of ease that is hard to beat, enhancing overall satisfaction.
In today’s tech-savvy world, where convenience often reigns supreme, an excellent user experience can make or break a speech recognition system’s adoption. When users find technology too challenging to navigate or unsatisfactory in its responses, they are likely to abandon it altogether, leading to substantial financial losses for developers. Therefore, focusing on UX is imperative for sustainable growth in the field of speech technology.
User Interface Design
The design of the user interface (UI) significantly shapes the user’s experience with speech recognition technologies. A well-crafted interface includes various elements that facilitate interaction, clarity, and responsiveness. Here are some key considerations:
- Visual Clarity: The layout should be intuitive. Users should not feel like they are wandering in a maze when trying to communicate with the system. Clear icons, simple navigation paths, and meaningful feedback can enhance the usability.
- Input Flexibility: Offering users multiple ways to engage with the system, like voice, touch, or gesture, ensures a broader reach and caters to varying preferences. This flexibility creates a user-friendly environment.
- Accessibility: Designing with all abilities in mind is critical. Speech recognition should be usable by individuals with disabilities, and UI elements should accommodate various needs.
For example, a smart home application may allow users to turn on lights via voice commands, but it should also let them control things through an app that has large tap targets and clear indicators showing when lights are on or off.
Feedback Mechanisms
Feedback mechanisms serve a pivotal role in creating a loop between the user and the technology, enabling constant interaction and reassessment. Without effective feedback, users may feel lost or uncertain while interacting with speech recognition systems. Some vital feedback mechanisms include:
- Response Confirmation: Indicators that confirm the system has understood and acted on the request can build user confidence. This could be as simple as an audible beep or a visual change on the screen.
- Error Correction Tools: Providing users with options to correct misunderstandings is crucial. If a user inadvertently triggers a wrong action due to miscommunication, giving them an easy way to rectify the mistake enhances the overall experience.
- User Initiated Feedback: Systems should enable users to rate their experiences or provide suggestions. This proactive approach allows for continuous improvement to the system, showing that user input is valuable and appreciated.
"User experience isn’t just about usability. It’s about how it feels to interact with a system. If someone struggles with commands, the technology is rarely adopted."
Challenges Facing Speech Recognition Technology
As speech recognition technology continues to evolve and permeate various domains, understanding the challenges it faces becomes paramount. Challenges are like the weeds in a garden, often obscured by the flowers of progress but must be addressed to attain the full potential of the technology. With more reliance on voice interfaces in our daily tasks—from voice assistants in smart homes to automated customer support—these challenges can’t be sidelined.
Accents and Dialects
Accents and dialects can be a double-edged sword in the landscape of speech recognition. While advancements have improved systems to understand and process language more effectively, the inherent diversity of human speech presents notable hurdles. When a voice recognition software is primarily trained on a specific accent, it can falter with users whose speech patterns diverge. Consider a scenario where an American English-speaking algorithm attempts to decode a Scottish dialect. The result might be less than fluent or accurate, leading to misunderstandings.
Furthermore, even within the same country, variations are plentiful. A system tailored for a Southern American accent may struggle with the nuances of a New York accent. This discrepancy can restrict accessibility for users from various backgrounds, thereby limiting the technology’s inclusivity, which, let's face it, should be a primary goal in any modern tech innovation.
Background Noise Interference
Another formidable challenge lies in background noise interference. Picture this: someone trying to dictate notes in a bustling café. Despite state-of-the-art noise-canceling technology, the chatter and clattering may lead the speech recognition software to misinterpret commands or fail altogether.
Key Factors Contributing to Background Noise Issues:
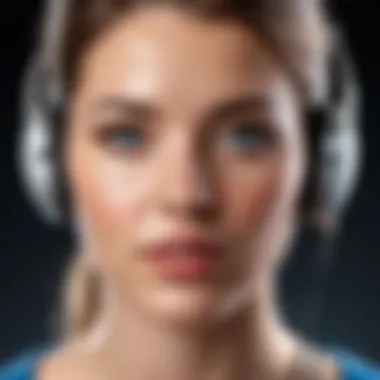
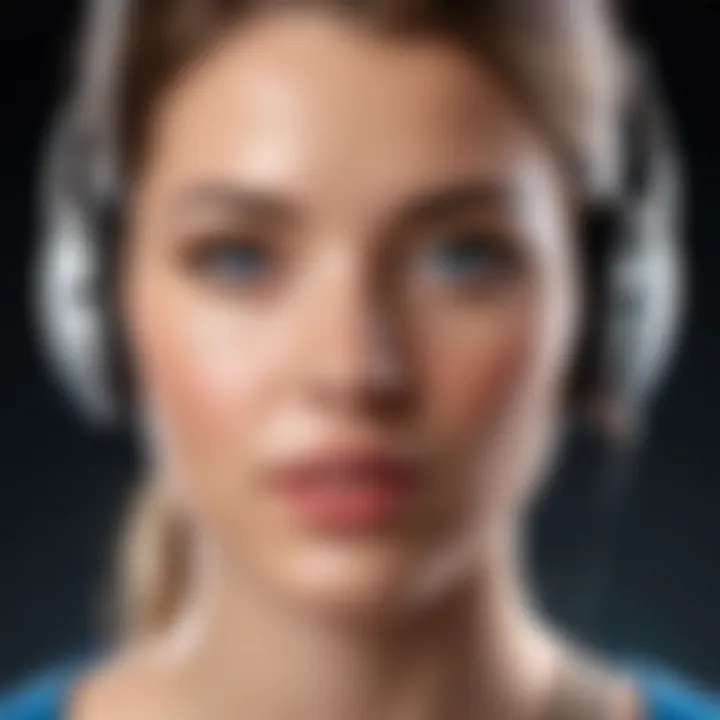
- Environmental Variability: Different settings, from quiet rooms to busy streets, can drastically affect performance.
- Microphone Quality: The type and quality of microphone used can determine the clarity and accuracy of captured audio.
- User Habits: How users interact with the software, like speaking closely or at an angle to the mic, can cause inconsistencies.
Addressing these factors demands continuous innovation and testing, prompting developers to create more robust models adaptable to a variety of auditory challenges. Without addressing background noise, the effectiveness of speech recognition could only be achieved in ideal conditions—hardly suitable for real-world applications.
Data Privacy Concerns
In a world where data breaches and privacy invasions frequently dominate headlines, data privacy concerns surrounding speech recognition cannot be ignored. The software often requires voice data collection to perform at its best, and with this collection comes the looming question of user consent and data usage.
Users may unwittingly surrender their voice data—essentially biometric information—putting their privacy at risk. Important Aspects to Consider:
- Storage and Security: How and where the data is stored is critical. Vulnerabilities in storage solutions can lead to unauthorized access.
- Transparency of Data Usage: Developers must be clear about how personal data is used, from improving algorithms to sharing with third parties.
- Regulatory Compliance: With regulations, such as GDPR in Europe, tightening the noose on data privacy, companies must navigate a complex landscape to remain compliant.
Addressing these concerns is not just about appeasing regulations but also about building trust with users. Users are more likely to engage with technology they feel is transparent and operates in their best interests.
Overall, it’s clear that the road ahead for speech recognition technology is fraught with challenges. Yet, by confronting issues related to accents, background noise, and data privacy head-on, developers can create solutions that not only function effectively but also promote accessibility, security, and user trust.
Future Directions in Speech Recognition
As the landscape of technology continually evolves, the future of speech recognition is teeming with potential. What may have once seemed like fragments of science fiction is rapidly morphing into everyday applications. This section explores the trajectory and significance of emerging trends in speech recognition, which not only enhance user experience but also transform how industries engage with technology.
Integration with IoT Devices
Integrating speech recognition capabilities with the Internet of Things (IoT) devices marks a monumental shift in how users interact with technology. In a world where smart devices are becoming commonplace—from smart thermostats to kitchen appliances—the ability to control these devices through voice commands is not merely a convenience but a necessity. Imagine walking into your home and simply saying, "Turn on the lights" or "Set the thermostat to 72 degrees." This ease of access enhances user experience and promotes higher engagement with technology.
The potential benefits of this integration are numerous:
- Enhanced Accessibility: For individuals with disabilities, voice command functionalities provide crucial assistance, enabling them to navigate their environment more freely.
- Streamlined Interactions: Users can manage multiple devices simultaneously, reducing the need for physical controllers or apps.
- Real-Time Feedback: Devices can be programmed to provide instant acknowledgments or information, improving the immediacy of communication and control.
However, this integration isn't without its pitfalls. Ensuring robust security protocols becomes paramount as increased connectivity can open the floodgates to vulnerabilities. Consequently, developers and manufacturers must focus on creating secure systems that can resist unauthorized access. The aim is to strike a balance between convenience and security, fostering peace of mind while navigating the digital landscape.
Advancements in Multilingual Support
As globalization continues to shape our world, the ability to support multiple languages in speech recognition systems translates directly to broader accessibility and usability. It's not just about recognizing American English or British English anymore; the demand for multilingual capabilities reflects the diverse tapestry of users around the globe.
What makes advancements in multilingual support essential? The answer lies in several factors:
- Wider Adoption: Enabling users to communicate in their native language increases engagement and satisfaction. A feature that recognizes and processes speech in multiple languages invites international users to adopt technology without language barriers.
- Cultural Sensitivity: Language is deeply intertwined with culture. Incorporating local dialects and colloquialisms into speech recognition not only enhances the accuracy of translations but also fosters a sense of representation and respect for diverse cultures.
- Market Expansion: Businesses seeking global reach must consider localized speech recognition functionalities for customer service applications, which can drastically improve user interaction and streamline support services.
Yet, achieving effective multilingual support presents distinct challenges. The variance in phonetics, accents, and speech patterns across different languages complicates the model training process. Developers must navigate these intricacies to ensure fluency and accuracy across languages.
In this fast-paced technological era, remaining responsive to the demand for advanced speech recognition features—particularly in integration with IoT devices and multilingual support—will be critical for fostering a more inclusive and efficient experience across the globe.
In summary, as we look toward the future, both the integration of speech recognition with IoT devices and advancements in multilingual capabilities stand out as essential pillars driving innovation. They not only promise a more seamless interaction with technology but also amplify the potential for understanding and connection among users worldwide. As the technology matures, we can expect these advancements will redefine our interaction with the digital world and reshape industries across the board.
Ethical Considerations
As speech recognition technologies continue to weave their way into the fabric of everyday life, it becomes increasingly important to scrutinize the ethical implications surrounding these advancements. Ethical considerations are not just an afterthought; they form an integral part of the conversation around speech recognition technologies. The impact of these technologies on society, culture, and individuals is profound, and it is essential to address potential pitfalls that might arise.
Bias in Algorithms
One significant ethical issue revolves around bias in algorithms. Machine learning systems, including those driving speech recognition, are only as good as the data they lean on. If the datasets used for training are skewed or unrepresentative, the algorithms can exhibit biases that affect performance. For instance, certain accents may be less recognizable, which leads to lower accuracy rates for users from diverse backgrounds.
- Impacts on User Experience: This can result in a frustrating user experience for those whose speech patterns do not match the algorithm's expectations. Imagine a person from a regional background attempting to use a voice assistant, only to have it misunderstand commands repeatedly. This creates an unequal playing field and reinforces existing disparities in technology use.
- Wider Societal Effects: On a broader scale, biased algorithms can perpetuate societal inequalities. If a speech recognition technology predominantly serves a demographic that is already privileged, it risks alienating other communities. Clients and organizations adopting such technologies must understand the biases of their tools and consider their potential impacts on diverse users.
User Consent and Data Collection
Another critical ethical consideration in speech recognition technologies is user consent and data collection. Most modern systems rely on collecting user data to improve their functionalities. While many individuals may want to enjoy the convenience of voice commands, they often do not fully grasp how their data is being used or stored.
- Transparency in Data Practices: Businesses need to cultivate transparency regarding data collection practices. Users should know what data is being gathered, how it will be used, and who it will be shared with. A lack of transparency can lead to distrust and anxiety among users about the safety of their information.
- Informed Consent: Adequate user consent is crucial. Users ought to actively opt in to data collection rather than being enrolled by default. This ensures that individuals feel empowered and informed about their choice, fostering a healthier relationship between technology and its users.
"Understanding ethical implications is not just good practice; it’s a necessity for sustainable technology development."
Closure
In wrapping up our exploration of speech recognition technologies, it's clear that this field wields significant influence across various facets of modern life. From personal assistants on smartphones to voice-activated systems in cars, the convenience and capabilities provided by efficient speech recognition can’t be overstated. It has irrevocably changed how we interact with technology, allowing for hands-free operations and making information more accessible than ever before.
Summarizing Key Points
Throughout the article, we delved into several key components of speech recognition technology.
- Definition and Importance: Speech recognition allows machines to interpret human speech, revolutionizing user interfaces and accessibility.
- Historical Context: Understanding the foundation, from early developments to modern milestones, highlights the rapid advancement in this area.
- Key Technologies: Elements such as acoustic models, language models, and feature extraction techniques are critical underpinnings of effective speech recognition systems.
- Algorithms: Algorithms like Hidden Markov Models, deep learning approaches, and reinforcement learning are pivotal, driving some of the most significant breakthroughs in recognizing speech accurately.
- Applications: Practical uses span from voice assistants to transcription services, underlining their vast capabilities.
- User Experience: The design and feedback mechanisms must be intuitive, aligning with user needs for optimal interaction.
- Challenges: Issues such as dialect variations, background noise interference, and data privacy are notable hurdles that need addressing.
- Future Directions: The integration of speech recognition with IoT devices seems promising, especially as demand for multilingual support grows.
- Ethical Considerations: With great power comes great responsibility; addressing biases and ensuring user consent is crucial for ethical practices.
In summary, speech recognition is not just another technological gimmick but rather a fundamental tool shaping communication in our digital age.
Implications for Future Development
Looking ahead, the implications of advancements in speech recognition are profound. As technology continues to evolve, we can anticipate:
- Broader Integration: With the Internet of Things, devices will become increasingly interconnected, utilizing voice commands to enhance user experiences across environments—homes, workplaces, and more. This push not only enhances convenience but also fosters more natural interactions with technology.
- Enhanced Multilingual Capabilities: As global connectivity increase, the demand for systems capable of interpreting and generating responses in multiple languages will only grow. This will not simply enable broader market access but will also enhance cultural inclusivity.
- Addressing Ethical Concerns: Companies will need to proactively work on minimizing algorithmic bias and enhancing data privacy measures. Such approaches will not only foster trust among users but also govern the legal frameworks surrounding speech recognition technologies.