Understanding Seismic Data Management: Key Insights
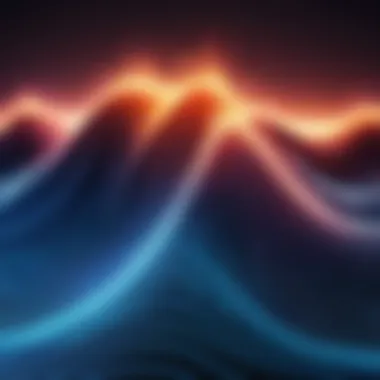
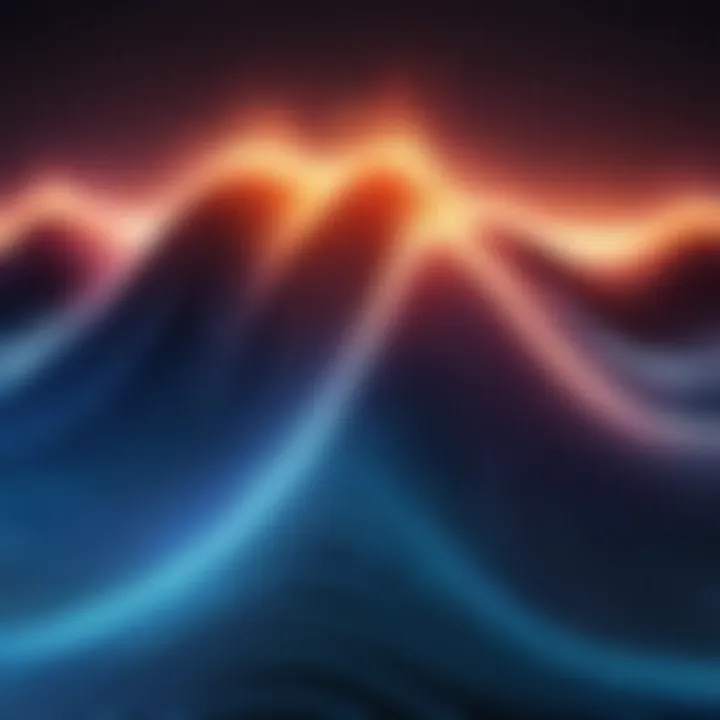
Intro
Seismic data management plays a vital role in the field of geosciences. With the increasing demand for effective natural resource exploration, the management of seismic data is becoming indispensable. This article will cover essential aspects of seismic data, including how it is acquired, processed, archived, and interpreted. A clear understanding of these processes will enhance the ability to make informed decisions in geological analyses and exploration activities.
The management of seismic data is not a standalone process; it intertwines various disciplines and technologies. By understanding the methodologies and technologies involved, professionals will improve their capacity to handle large volumes of data systematically. Moreover, this article will identify current trends within seismic data management, keeping the reader updated with contemporary practices.
Brief Description
Seismic data management involves the usage of specialized software that assists in the acquisition, processing, and archiving of seismic data. Such software is crucial for operational efficiency and the effectiveness of geological studies.
Overview of the Software
Seismic data management software enables geoscientists to interact effectively with data. Tools like Schlumberger's Geoframe, Halliburton's Landmark, and Ikon Science's Jason are some examples that provide platforms for coherent data handling. Such tools ensure that data flows seamlessly from acquisition through processing to dissemination.
Key Features and Functionalities
- Data Acquisition: These tools support integration with field devices, facilitating seamless data collection.
- Data Processing: Advanced algorithms are applied to enhance the quality of seismic signals, identifying relevant geological features.
- Data archiving: Efficient storage solutions are provided for future reference, allowing historical data retrieval and analysis.
- Interpretation tools: These tools assist in visualizing complex seismic datasets, making geological insights achievable.
System Requirements
To run seismic data management software effectively, certain system requirements must be met. Understanding these will ensure optimal performance and minimize downtime.
Hardware Requirements
- Processor: A multi-core processor to handle extensive computations.
- Memory: A minimum of 16 GB RAM is recommended for handling large datasets efficiently.
- Storage: SSDs are preferred due to their faster read/write speeds, with at least 1 TB available for data storage.
Software Compatibility
It is crucial that the seismic data management software is compatible with various operating systems and integrates seamlessly with other analytical tools. Compatibility with systems like Windows, Linux, and MacOS is often advertised by leading software developers.
This foundational understanding sets the stage for exploring deeper into the principles and practices that govern seismic data management. As we proceed, the article will address methodologies, best practices, and future advancements in the field.
Intro to Seismic Data Management
Seismic data management encompasses a vital aspect of geoscience. Its primary objective is to methodically manage seismic data through all its stages: acquisition, processing, storage, and analysis. As the demand for detailed geological insights continues to grow, effective data management practices become indispensable. The ability to handle seismic data efficiently supports critical decisions in resource exploration, environmental assessments, and even seismic risk mitigation.
Definition and Importance
Seismic data management can be defined as the systematic control and organization of seismic data throughout its life cycle. This encompasses the collection of raw data, processing it into usable formats, and ensuring its integrity and accessibility. The importance of this discipline is underscored by its role in informing scientific research and industrial applications. Accurate seismic data can detail subsurface geological structures, enabling better decision-making. As industries increasingly rely on data analytics, robust seismic data management practices are emerging as pivotal.
"Effective management of seismic data not only enhances the reliability of geological interpretations but also drives innovation in data analysis techniques."
In practical terms, this means creating protocols to ensure data is not only stored but also preserved and updated to reflect technological advancements. For IT professionals, familiarity with seismic data management processes can yield advantages in data analysis, machine learning applications, and cloud integration.
Historical Context
The historical evolution of seismic data management reflects significant technological advancements. Initially, data collection relied predominantly on analog methods, which were labor-intensive and brought limited efficiency. Over the decades, digital technologies revolutionized the seismic field, paving the way for faster data processing and improved storage solutions.
In the early 1970s, the first digital seismic data recording systems were introduced, enabling researchers to capture data with unprecedented detail. As computer technology evolved through the late 20th century, seismic data management systems became more sophisticated, employing various software solutions to facilitate data interpretation and sharing across networks.
Today, the landscape of seismic data management is shaped by ongoing advancements in big data, artificial intelligence, and cloud computing. Modern systems focus not only on storage but also on ensuring data quality, integration across platforms, and compliance with industry regulations. Understanding this historical context provides useful insights into current challenges and the direction of future innovations.
Types of Seismic Data
Seismic data serves as a foundation for geological exploration and analysis. Understanding the different types of seismic data is crucial for professionals in the field, as it allows them to choose the appropriate data for various applications. Each type of data contributes uniquely to seismic studies, affecting how researchers interpret geological structures and make informed decisions based on analysis.
Raw Seismic Data
Raw seismic data is the initial output obtained from seismic surveys. It consists of the unprocessed signals recorded during field data acquisition. These signals are collected through sensors, which capture ground motion caused by seismic waves. The significance of raw seismic data lies in its authenticity. Being the original form of data, it contains all the nuances of the seismic events. This raw data is vital for understanding the actual conditions and variations in the subsurface environment.
The benefits of analyzing raw data include the ability to apply various processing techniques tailored to specific research goals. However, this data comes with challenges, like its volume and the need for expertise in processing techniques to ensure meaningful interpretation.
Processed Seismic Data
Processed seismic data is derived from the initial raw data after applying signal processing techniques. This stage often involves filtering, stacking, and enhancing the signal. The purpose is to improve signal-to-noise ratio, making the data clearer and easier to interpret.
The processing of seismic data transforms it into a more usable form. This includes creating seismic profiles and images that highlight geological features. The processed data offers actionable insights for geoscientists and engineers. However, it is important to credit the original methodologies used in processing to maintain the integrity of the data analysis. The observer effect can sometimes influence outcomes during this phase, making proper techniques vital for maintaining data quality.
Interpreted Seismic Data
Interpreted seismic data is the final stage, where processed data undergoes interpretation to extract geological insights. Geoscientists use specialized software tools and methodologies to analyze the data for identifying and characterizing subsurface formations. The value in this stage lies in revealing structural and stratigraphic details crucial for exploration and resource management decisions.
Interpreted data often serves as the bridge between raw data and practical application. This interpretation can lead to discovering potential oil or gas reserves, guiding drilling activities, and assessing geological hazards. However, it requires skilled professionals with a deep understanding of both geology and the seismic dataset at hand. Employing best practices in interpretation significantly enhances the reliability of findings.
Importance: Each type of seismic data is crucial for providing a comprehensive understanding of subsurface conditions. The transition from raw to interpreted data emphasizes the importance of methodological rigor and demonstrates the depth of geological insights available.
Data Acquisition Techniques
Data acquisition techniques are fundamental to the field of seismic data management. They involve methods and technologies used to gather seismic data from the Earth's subsurface. Correctly applied, these techniques can lead to more accurate interpretations of geological formations, therefore guiding exploration and research decisions.
Field Data Collection
Field data collection is the backbone of seismic studies. It includes processes designed to capture raw seismic data in real environments. This typically involves deploying sensors such as geophones or accelerometers that record ground movement. Each sensor converts vibrations caused by geological events into electrical signals. The signals are then digitized for storage and analysis.
Key elements of field data collection include:
- Selection of Sites: Choosing appropriate locations for seismic surveys is crucial. The site should ideally allow for undisturbed data collection, minimizing noise.
- Equipment Calibration: Proper calibration of equipment ensures the accuracy of recorded data. Miscalibrated sensors can produce significant errors in seismic analysis.
- Survey Design: This involves planning the layout of sensors in a grid pattern which helps in covering a significant area while optimizing data quality.
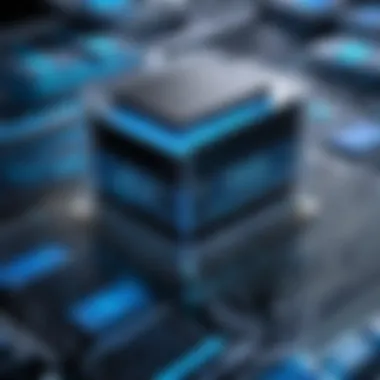
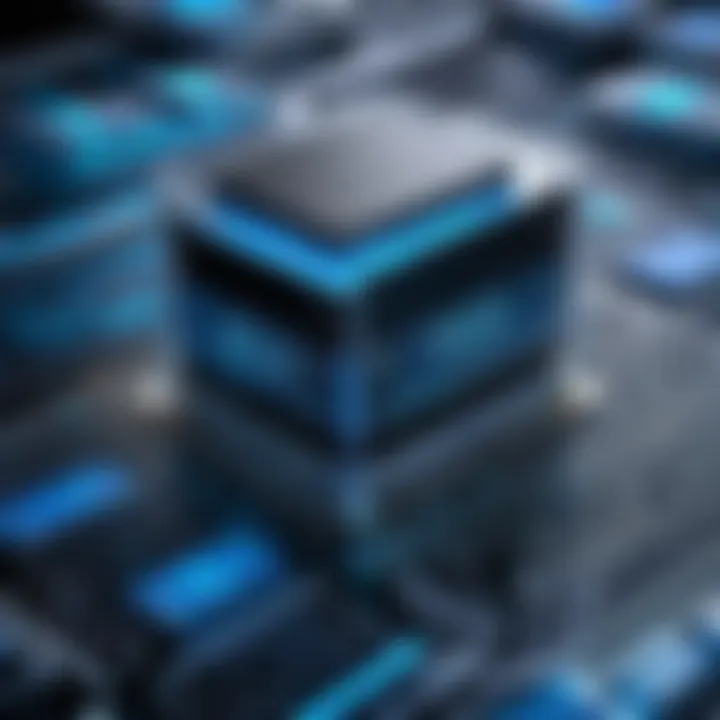
Advantages of effective field data collection include:
- Increased data quality leading to better results in subsequent processing and interpretation.
- Reduced costs associated with redoing fieldwork due to the collection of faulty data.
Considerations such as weather conditions and terrain can impact field data collection. Thus, project teams must be well-prepared to adapt to sudden changes in environment or equipment failures which can arise during the surveying process.
Remote Sensing in Seismic Data
Remote sensing is a technique that employs satellite or aerial technology to collect seismic data. It offers a different approach to data acquisition, enabling the capture of geological features and changes over broad areas, often inaccessible by traditional means.
Key aspects of remote sensing include:
- Overview: Remote sensing uses various technologies such as LiDAR, drones, or satellite imagery to gather data. These tools can provide information on surface conditions and changes, aiding in subsurface analysis.
- Advantages: This technique covers extensive area quickly and can capture dynamic processes over time. For instance, it allows monitoring of land deformation post-earthquake.
- Data Integration: Combining remote sensing data with field data enhances the clarity of geological interpretations. When fused, these two data types enable a more cohesive understanding of the subsurface environment.
There are also specific challenges and considerations when applying remote sensing:
- The resolution of the data may vary based on technology. Higher resolution often comes with higher costs, needing balance in project planning.
- Environmental factors might influence data accuracy and must be accounted for in analysis.
Data Processing and Analysis
Data processing and analysis is a cornerstone of seismic data management. The integrity and utility of seismic data depend heavily on how effectively it is processed and analyzed. This section focuses on the significant techniques and methodologies that professionals in the field employ to convert raw seismic data into valuable insights, facilitating geological assessments and informed decision-making.
Signal Processing Techniques
Signal processing is crucial for enhancing the quality of seismic data. By applying various techniques, professionals can extract useful information from the raw seismic signals that often contain noise, interference, and artifacts. Some of the prominent signal processing techniques include:
- Filtering: This is fundamental for removing unwanted frequencies from the data. High-pass and low-pass filters help isolate signals of interest, improving the clarity of seismic events.
- Fourier Transform: This technique converts time-domain data into frequency-domain data, making it easier to analyze complex signals. Understanding the frequency components allows for better interpretation of subsurface structures.
- Waveform Analysis: By analyzing the shape and characteristics of seismic waves, geoscientists can infer information about the material properties of geological formations.
Implementing these techniques requires specialized software and tools, such as MATLAB or Seismic Unix. Effective use of signal processing techniques leads to improved data quality, which, in turn, affects the reliability of interpretations made from the seismic data.
Data Interpretation Methods
Once the data has been processed, the next crucial step is interpretation. Seismic data interpretation involves making assumptions and conclusions regarding subsurface structures and potential resources. Various methods are employed:
- Manual Interpretation: Experts use their knowledge and experience to assess data visually, analyzing wave patterns and anomalies. This method is subjective but leverages human intuition.
- Automated Interpretation: Advanced algorithms and machine learning models are increasingly used to automate the interpretation process, allowing for consistent analysis of large datasets. By using techniques such as clustering and classification, software can identify patterns that could indicate oil and gas reservoirs or fault lines.
- Modeling and Simulation: Creating models of subsurface geology is essential for predicting seismic responses. Software tools simulate how seismic waves travel through the Earth, providing insights into geological formations and helping validate interpretations.
The synergistic relationship between processing and interpretation enhances the overall seismic data management process, leading to more accurate geological analyses.
Through structured processing and systematic interpretation, the potential of seismic data management can be fully realized. Understanding these techniques and their applications is vital for IT professionals, software developers, and businesses involved in geosciences and exploration.
Seismic Data Storage Solutions
The management of seismic data necessitates a robust storage system. As data volume expands, seismic data storage solutions become increasingly critical. These systems are designed not only to house large datasets but also to ensure data integrity, accessibility, and security.
In seismic data management, the choice of storage solution can have significant implications on analysis and decision-making processes. Efficient storage enables geoscientists to perform timely evaluations and fosters collaboration across different institutions, enhancing research outcomes. On the other hand, inadequate storage can lead to data loss, corruption or difficulty in accessing required datasets, thus hindering ongoing projects.
Efforts to optimize storage solutions can greatly improve workflows within seismic data management. It is vital to consider several factors, such as the type of data being stored, the expected frequency of use, and budget constraints when deciding on a storage solution.
Traditional Storage Techniques
Traditional storage techniques often involve physical media like tapes and hard drives. These methods have their advantages, particularly in data security and controlled environments.
- Durability: Physical media can be incredibly durable if stored correctly, which may prevent data loss.
- Cost-Effectiveness: For some organizations, traditional storage can be more economical, especially when large upfront investments in technology are not feasible.
- Limited Networking Needs: These systems can function independently, which mitigates concerns of network failures.
However, traditional storage techniques come with limitations as well:
- Space Constraints: As data grows, physical storage space can become a serious issue. Finding room for expanding datasets can be challenging and costly.
- Access Speed: Retrieving data can be slower compared to modern digital solutions, hampering quick decision-making.
- Maintenance Challenges: Maintaining physical systems and ensuring data backups can require considerable IT resources.
Cloud-Based Storage
Cloud-based storage solutions represent a significant advance in data management strategies. They allow users to store and access seismic data through the internet, providing flexibility and scalability.
- Accessibility: Users can access data from any location, fostering collaboration among different teams and organizations.
- Scalability: As data needs grow, cloud storage can be easily scaled to accommodate additional data without the need for upfront investment in hardware.
- Cost Efficiency: Most cloud storage options operate on a pay-as-you-go model, which can be more budget-friendly for many organizations.
- Data Security: Cloud providers typically invest in high-level security protocols, which can often exceed the capabilities of traditional storage techniques.
However, cloud storage does have its downsides:
- Internet Dependency: Users must rely on stable internet connections to access the data.
- Data Migration Challenges: Transitioning from traditional storage to cloud solutions can be complex and may pose challenges regarding data integrity during transfer.
- Common Security Concerns: Despite advancements in security, concerns about data breaches and unauthorized access remain prevalent.
As organizations navigate through various storage solutions, it is essential to carefully weigh these factors to determine the most suitable approach for their specific needs. Understanding both traditional and cloud-based options helps in creating a balanced storage strategy that enhances overall data management.
Data Management Systems
Data management systems are vital in the field of seismic data management. They serve as the backbone for organizing, storing, and retrieving vast amounts of seismic data efficiently. These systems allow professionals to handle data effectively, ensuring their research and exploration efforts are backed by robust and reliable information. The importance of these systems cannot be overstated as they enhance data accessibility and integrity, which are crucial for making informed decisions in geological analysis.
Overview of Management Systems
Management systems in seismic data management employ structured practices and advanced technologies to process data from different sources. They enable the integration of raw, processed, and interpreted data into a single platform, promoting seamless workflows. Key components of management systems include:
- Data Storage: Securely storing seismic data in a centralized location.
- Access Control: Managing who can view or edit the data to ensure its integrity.
- Data Retrieval: Providing efficient tools for users to query and retrieve relevant datasets.
- Version Control: Keeping track of data revisions and changes over time.
These systems benefit organizations by optimizing their data handling processes, thus improving operational efficiency and reducing costs.
Software Solutions for Seismic Data
Various software solutions are available to facilitate seismic data management. Each solution brings unique features tailored to the specific needs of the industry. Some notable options include:
- Geosoft Oasis montaj: Known for its powerful geospatial data management, allowing for effective visualization and analysis of seismic data.
- Schlumberger’s Petrel: Offers comprehensive tools for data integration, enabling the interpretation and modeling of subsurface data.
- Paradigm’s SYSName: A versatile data management platform that provides tools for data visualization and workflow automation.
- Halliburton’s Landmark Software: Facilitates collaborative usage of seismic data across teams, enhancing project efficiency.
When selecting software solutions, organizations should assess their specific requirements in terms of data types, user accessibility, and integration capabilities.
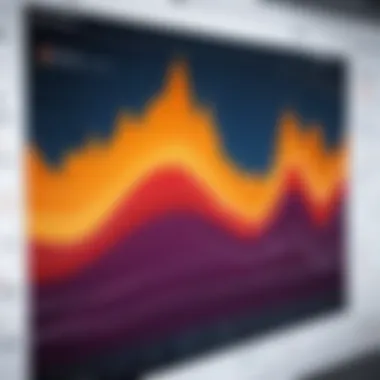
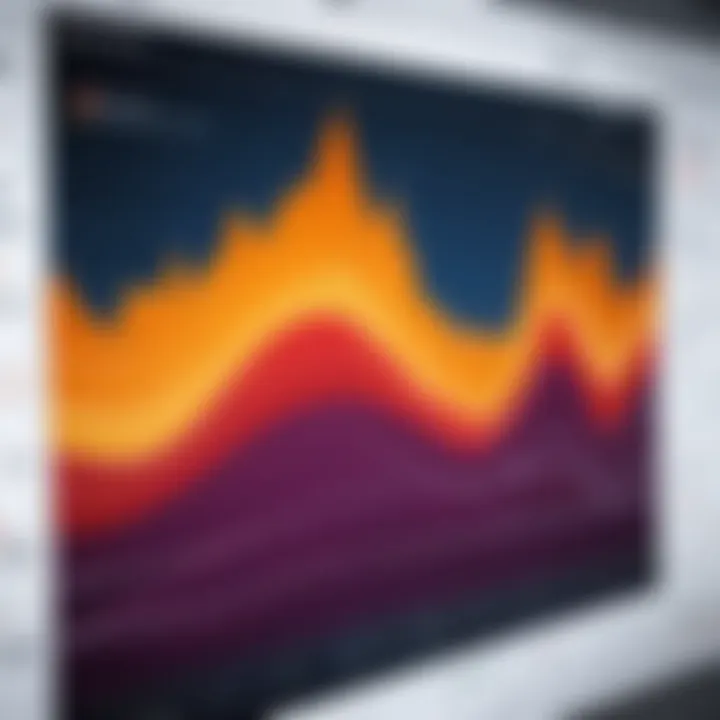
"Robust software solutions for seismic data management increase productivity and enhance collaboration among teams."
By leveraging these software tools, companies can ensure that they are making the most of their seismic data assets, leading to better-informed decisions in exploration and research.
Best Practices in Seismic Data Management
Effective seismic data management requires the integration of best practices that ensure the integrity, quality, and accessibility of data. As the volume of seismic data grows, maintaining standards in data handling enhances both operational efficiency and research quality. Professionals in the field must prioritize these practices to ensure valuable insights can be derived while minimizing the risks associated with poor data management.
Data Integrity and Quality Control
Data integrity is a cornerstone of seismic data management. It refers to the accuracy and consistency of data throughout its lifecycle. Poor data integrity can lead to erroneous interpretations, ultimately affecting decision-making processes during exploration and research.
To ensure data integrity, organizations can implement various strategies:
- Regular Audits: Scheduled checks on data can help identify inconsistencies. Such audits can include comparing raw data against processed outcomes.
- Validation Techniques: Utilizing automated validation routines during data processing can prevent errors from propagating.
- Redundancy Measures: Keeping multiple copies of data in different locations safeguards against data loss. This approach not only protects data integrity but also enhances its availability.
Furthermore, employing quality control protocols is essential. These protocols should encompass:
- Standardization: Establishing clear guidelines for data capture, processing, and storage ensures uniformity across datasets.
- Training: Investing in ongoing education for personnel will help them stay abreast of emerging technologies and methodologies. Well-trained staff are better equipped to uphold quality standards in data management.
"The importance of maintaining high standards in data quality cannot be overstated. It is the bedrock upon which successful seismic analysis is built."
Effective Documentation Strategies
Effective documentation is vital in seismic data management. It provides context and clarity, allowing future users to understand the data's lineage, processing history, and any limitations.
Key elements of a robust documentation strategy include:
- Data Provenance: Maintaining thorough records of where data originates, how it has been processed, and which tools were used ensures transparency. This traceability fosters trust among stakeholders.
- Metadata Standards: Implementing consistent metadata standards aids in categorizing and retrieving data efficiently. Consideration should be given to including details about acquisition, processing tools, and analytical methods.
- Version Control: Tracking changes and updates to seismic data ensures that users are working with the most current information. Utilizing version control systems can help manage edits collaboratively.
Moreover, organizations should encourage a culture that values documentation. Regular training sessions and easy access to documentation tools can enhance compliance across teams. In practice, effective documentation strategies facilitate smarter data usage, ultimately leading to advancements in geological understanding and exploration strategies.
Legal and Ethical Considerations
Legal and ethical considerations in seismic data management are critical to ensuring compliance with regulations and maintaining public trust. With the vast amount of data collected, organizations must navigate various legal frameworks that dictate how data is acquired, stored, and utilized. Ethical issues also arise from the use of data, impacting both the integrity of the research and the rights of individuals involved.
Regulations Impacting Data Management
Regulatory frameworks surrounding seismic data management are established to protect sensitive information and promote responsible usage. Key regulations include:
- Data Protection Laws: Laws such as the General Data Protection Regulation (GDPR) in Europe govern the collection and processing of personal data. Companies must ensure that their seismic data practices align with these laws.
- Environmental Regulations: Agencies like the Environmental Protection Agency (EPA) often require seismic surveys to comply with environmental assessments. This ensures that data collection does not adversely affect ecosystems or communities.
- Intellectual Property Rights: Seismic data can be subject to copyright or patent protections. Organizations should respect these rights to avoid legal disputes.
Understanding and adhering to these regulations are essential for any organization engaged in seismic data management. Non-compliance can lead to hefty fines and damage to reputations.
Ethical Data Use
Ethical data use is paramount in fostering trust and accountability in seismic data management. Here are several key considerations:
- Transparency: Organizations should communicate openly about how seismic data is collected and used. This promotes trust among stakeholders, including the public and regulatory bodies.
- Consent: Obtaining consent from individuals affected by seismic surveys is not merely a legal requirement; it is an ethical obligation. It is critical to respect individuals' rights to make informed decisions about their data.
- Data Security: Protecting seismic data from breaches is essential. Organizations must implement robust security measures to safeguard sensitive information.
"Ethical considerations should be embedded in the core values of seismic data management."
Emerging Technologies in Seismic Data Management
Emerging technologies are transforming the field of seismic data management. As the demand for precise geological analysis grows, it is crucial to embrace these advancements to enhance data acquisition, processing, and analysis. Today’s seismic environments are increasingly complex, necessitating innovative solutions. By integrating cutting-edge technologies, organizations can maximize the value of their seismic data.
Artificial Intelligence Applications
Artificial intelligence (AI) offers invaluable tools for interpreting seismic data. AI algorithms can process vast volumes of data rapidly compared to traditional methods. For instance, companies now utilize AI to detect patterns in seismic waves. This capability aids geoscientists in identifying potential oil and gas reservoirs more efficiently.
There are several key benefits to using AI in seismic data management:
- Speed: AI systems can analyze data in real-time, significantly reducing interpretation time.
- Accuracy: Advanced algorithms improve detection rates of geological features which may be missed by human analysts.
- Automation: Manual tasks can be automated, freeing up human resources for more strategic decision-making.
- Predictive analytics: AI can model potential outcomes based on historical data, assisting in risk assessment and exploration strategies.
In adopting AI technologies, organizations should be mindful of the following considerations:
- Data Quality: The accuracy of AI applications relies heavily on the quality of input data. Poor quality data can lead to misleading results.
- Interdisciplinary Expertise: Implementing AI requires collaboration between geoscientists and data scientists, ensuring that algorithms align with geological insights.
- Ethical Concerns: As with any AI implementation, ethical considerations regarding data use and bias should not be overlooked.
Machine Learning for Data Analysis
Machine learning (ML), a subset of AI, is particularly significant in seismic data analysis. ML algorithms learn from data and improve their performance over time. This is crucial in seismic studies where historical data is abundant and complex. By identifying trends and establishing correlations, machine learning enables deeper insights into subsurface structures.
The applications of machine learning in seismic data include:
- Clustering: Grouping similar seismic events or geological formations helps in the identification of patterns that inform exploration decisions.
- Classification: ML can categorize seismic signals, distinguishing between natural phenomena and potential risks.
- Regression Analysis: This technique is useful for predicting quantitative outcomes based on input variables, such as estimating the probability of finding resources in a given area.
Organizations should consider certain factors when deploying machine learning techniques:
- Training Data: The effectiveness of ML models depends on well-curated training datasets. Better datasets yield better outcomes.
- Model Maintenance: Regular updates to models are necessary to adapt to new data and trends, ensuring the relevance of findings.
- Interoperability: ML solutions should integrate seamlessly into existing seismic data management workflows to maximize efficiency.
"The integration of emerging technologies like AI and ML is essential for the advancement of seismic data management. They not only enhance efficiency but also improve the quality of geological insights."
Future Trends in Seismic Data Management
The landscape of seismic data management is evolving. Professionals in the geoscience field must stay abreast of these changes. Future trends promise to reshape how we approach seismic data collection, interpretation, and usage. Emerging technologies and methodologies offer benefits that can enhance accuracy, efficiency, and collaboration in seismic studies. Working with seismic data becomes more intuitive as innovations take hold. Below, we explore two significant trends shaping the future of seismic data management, namely the increasing importance of big data and the integration with other geoscience data.
Increasing Importance of Big Data
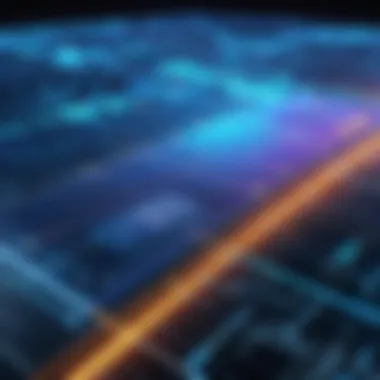
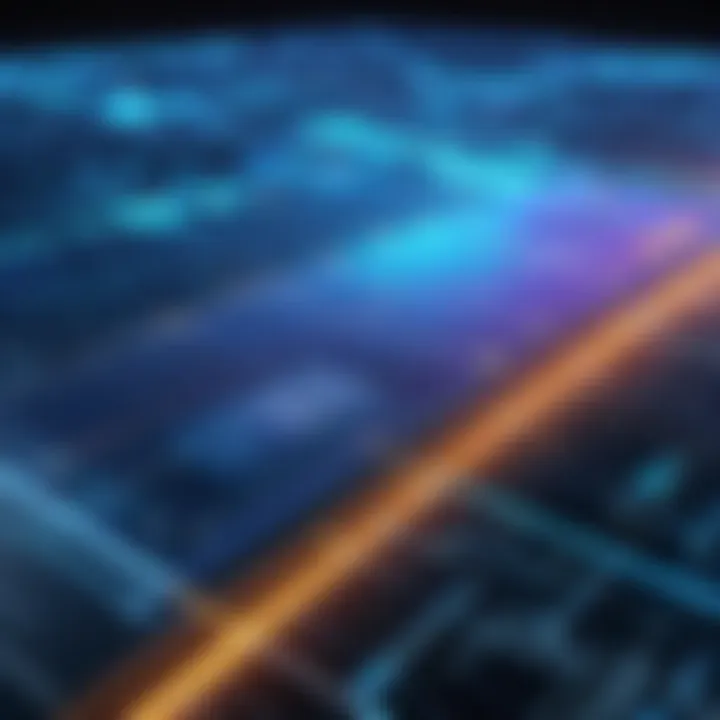
The seismic industry is experiencing an influx of large datasets from various sources. This surge is primarily due to advancements in technology and increased data collection capabilities. Harnessing this big data is vital for extracting meaningful insights.
Big data analytics can improve prediction models and provide deeper geological insights. By leveraging tools and techniques tailored for large datasets, geoscientists can enhance their analyses. This results in more accurate findings regarding subsurface structures and behaviors.
Key aspects of utilizing big data in seismic management include:
- Data Processing: Efficient algorithms are needed to manage and analyze vast amounts of data quickly.
- Storage Solutions: Advanced storage options must accommodate data growth while ensuring accessibility.
- Real-time Analytics: Capturing and processing seismic data in real-time can facilitate immediate decision-making, crucial during exploration and emergency response situations.
With the adoption of big data, seismic data management stands to gain from improved workflows that can streamline operations. This trend not only gives professionals new tools but also elevates the standard for data-driven decision-making.
Integration with Other Geoscience Data
As industries evolve, collaboration between disciplines becomes more pronounced. In seismic data management, integrating with other geoscience datasets can enable a more holistic view of geological phenomena. This integration typically includes combining seismic data with data from geochemistry, satellite imagery, and geological surveys.
Benefits of integrating various geoscience data types include:
- Enhanced Data Analysis: Correlating seismic data with geological information can refine interpretations and hypotheses.
- Broader Context: Understanding seismic events within a larger geoscience framework can influence exploration strategies and risk assessments considerably.
- Collaborative Platforms: Creating shared platforms for data access encourages teamwork among scientists, fostering innovation through shared knowledge.
In the future, the seismic data management landscape must emphasize collaboration and data integration. This trend will likely result in more comprehensive explorations and advancements in the geosciences as a whole.
"The integration of seismic data with other earth science disciplines will open new avenues for discovery and understanding."
Collaborative Approaches to Data Management
The advent of collaborative approaches in seismic data management has reshaped how organizations interact and share valuable information. This section elucidates the pivotal role of collaboration in enhancing data accessibility and application. In a landscape where geological data plays a vital role in exploration, resource management, and environmental assessments, the integration of collective efforts not only optimizes operational efficiency but also broadens the scope of analysis.
Inter-Organizational Sharing
Inter-organizational sharing of seismic data offers considerable advantages. When different organizations share their findings, it leads to a richer, more comprehensive understanding of geological issues. Such collaboration fosters a culture of openness and resource utilization.
- Enhanced Data Quality: Organizations can mitigate errors present within their individual datasets by cross-referencing and validating shared data. This process significantly improves the overall quality, which is essential for accurate analyses and reports.
- Wider Range of Data: By pooling together various seismic datasets from multiple organizations, researchers and analysts gain access to diverse data. This comprehensive dataset allows for broader insights and fosters innovation in analysis techniques.
- Cost Efficiency: Data sharing reduces duplication of efforts and associated costs. Instead of many organizations collecting similar data independently, they can collaborate in data collection and analysis, saving time and resources.
In summary, fostering inter-organizational partnerships in seismic data management not only enhances data quality but also promotes a cooperative spirit that drives technological and analytical advancements.
Community-Driven Data Initiatives
Community-driven data initiatives represent another critical facet of collaborative approaches to seismic data management. Local communities, researchers, and stakeholders working together significantly impact data acquisition and application, enhancing relevance and accessibility.
- Local Expertise: Community members often possess unique knowledge of the geographic and geological contexts. Their contributions can illuminate patterns and insights that may elude traditional analysis methods. This localized knowledge becomes invaluable in tailoring seismic data interpretation to specific regional challenges.
- Open Data Platforms: Initiatives that promote open data access encourage a wider audience to engage with seismic data. Such platforms not only facilitate data sharing but also inspire collaboration among independent researchers, educators, and students who can use the data for various applications, from academic studies to practical projects.
- Engagement and Education: Community-driven initiatives often involve educational components, empowering local entities with the skills to analyze and utilize seismic data. This engagement fosters a sense of ownership and responsibility towards regional geological insights and problem-solving efforts.
Case Studies in Seismic Data Management
Case studies in seismic data management are critical for understanding the practical application of theories and methods within the field. They provide real-world examples that illustrate the challenges and successes encountered during seismic data management initiatives. By analyzing these cases, professionals can gain insights into best practices, technological advancements, and strategic approaches that lead to successful outcomes. The significance of these case studies lies not just in the successes achieved, but also in the lessons gleaned from setbacks. This pragmatism enhances the collective knowledge of stakeholders in the seismic domain.
Successful Implementations
Successful implementations of seismic data management systems serve as benchmarks for the industry. These cases often highlight the integration of advanced technologies such as cloud storage and machine learning algorithms. For instance, a major oil and gas company utilized cloud-based seismic data storage solutions to streamline their data processes. By doing so, they significantly reduced data retrieval times and enhanced collaboration between teams across different geographical locations. This implementation not only increased efficiency but also facilitated the sharing of insights and findings in real-time.
Another example involves the use of seismic interpretation software that integrates artificial intelligence. Companies that adopted this technology reported improved accuracy in identifying potential drilling sites. The software analyzes vast amounts of seismic data to detect patterns and anomalies that are often missed through manual observations. This case exemplifies how implementing cutting-edge tools can lead to superior decision-making and reduced operational risks.
Lessons Learned
The process of evaluating case studies reveals several crucial lessons for professionals in the field. Firstly, it is evident that comprehensive training for personnel is paramount. Implementations that included extensive training programs for users tended to achieve higher success rates. Moreover, understanding user needs and ensuring that technology aligns with those needs is essential for fostering user adoption.
Secondly, proper planning and phased execution can significantly mitigate risks associated with seismic data management projects. In one case, a company faced substantial setbacks due to inadequate project management. This reinforced the importance of employing project managers who understand both the technical and operational aspects of seismic data handling.
Thirdly, maintaining robust data governance and security protocols cannot be overlooked. Data breaches in one case led to significant financial and reputational damage, underscoring the necessity of implementing strict access controls and regular audits.
"Successful seismic data management relies not only on the technology used but also on the people and processes behind it."
Networking and Professional Development
Networking and professional development are crucial for individuals engaged in seismic data management. These aspects allow professionals to exchange knowledge, share experiences, and gain insights into the latest trends and technologies affecting the field. Effective networking fosters relationships that can lead to collaboration, mentorship, and career advancement—all essential in a rapidly evolving discipline.
Professionals in seismic data management can benefit significantly from attending industry conferences and workshops. Such events provide platforms for learning from experts, understanding new methodologies, and engaging with leading technologies. They also serve as venues for significant moments of shared learning, where practitioners discuss practical challenges and solutions surrounding data management and processing.
Industry Conferences and Workshops
Industry conferences and workshops are pivotal in creating an environment conducive to professional growth. These events typically feature presentations from key figures in the field, including industry leaders and academic researchers. The exchange of ideas and sharing of case studies during these gatherings allow professionals to reflect on best practices and potential pitfalls in seismic data management.
Moreover, hands-on workshops offer practical experiences. Participants can learn new software tools, data analysis techniques, and data management practices. These learning opportunities can be applied immediately in professional settings, promoting improved workflows and data integrity in operations.
Networking during such events can also lead to valuable contacts. Professionals can meet with peers, potential collaborators, and industry experts. Establishing these connections may provide invaluable resources for career development and project success.
Online Resources and Communities
In today's digital age, online resources and communities enable professionals to continue their learning and networking even outside of formal events. Various platforms and forums provide access to invaluable information, discussions, and support networks.
- Online Forums: Websites like Reddit host specific subreddits focused on geosciences and seismic data. Such platforms allow professionals to engage in discussions, pose questions, and share insights on current challenges.
- Webinars and Online Courses: Many organizations offer webinars and courses that focus on seismic data management, covering topics from data acquisition to advanced processing techniques. These educational resources are crucial for continuous professional development.
- Social Media Groups: Platforms like Facebook host groups where professionals can share experiences and resources related to seismic data management. Members can post articles, ask for advice, or discuss industry trends.
Utilizing these online resources helps professionals remain connected, informed, and engaged. This ongoing interaction fortifies their understanding of the field and enhances their capability to contribute effectively to seismic data management.
Finale
The conclusion of this article encapsulates the significant insights gleaned from exploring seismic data management. This discipline stands out as a pivotal element in geosciences, where understanding and utilizing seismic data can reshape exploration strategies and deepen geological analysis. The importance of effective data management cannot be overstated, as it impacts both the integrity of the data collected and the validity of the interpretations drawn from it.
Recap of Key Points
In reviewing the core aspects discussed throughout the article, several key points emerge:
- Definition and Importance: Seismic data management involves the systematic approach to acquiring, processing, archiving, and interpreting seismic data. Its relevance grows alongside the complexities of modern geological studies.
- Types of Data: The distinctions between raw, processed, and interpreted seismic data have been well-established, highlighting the importance of each stage in the data's lifecycle.
- Techniques and Technologies: Various data acquisition techniques, coupled with advanced processing and analysis methods, ensure that the gathered data transforms into actionable insights effectively. Technologies like cloud-based storage solutions enhance the accessibility and efficiency of seismic data management.
- Legal, Ethical, and Best Practices: Adhering to legal regulations and ethical considerations is crucial for data integrity. Implementing best practices further ensures data quality, security, and reliability.
- Future Trends: As Big Data continues to evolve, its importance within seismic data management will only increase, promising more integrative approaches across geoscientific disciplines.
Final Thoughts on Future Directions
Looking ahead, the landscape of seismic data management is poised for transformative advancements. With the integration of emerging technologies, such as artificial intelligence and machine learning, the potential for sophisticated analysis is substantial. These tools not only enhance data interpretation but may also lead to predictive modeling, which can significantly affect exploration strategies. Furthermore, there is a growing necessity for inter-organizational collaboration, fostering an environment where data sharing and mutual learning can thrive.