Value-Based Analytics: Enhancing Organizational Outcomes
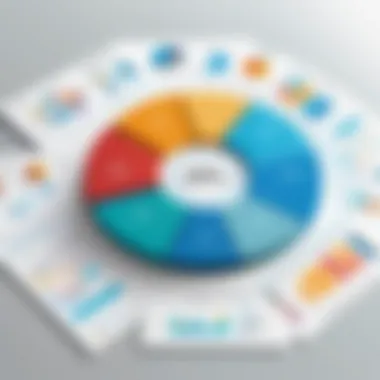
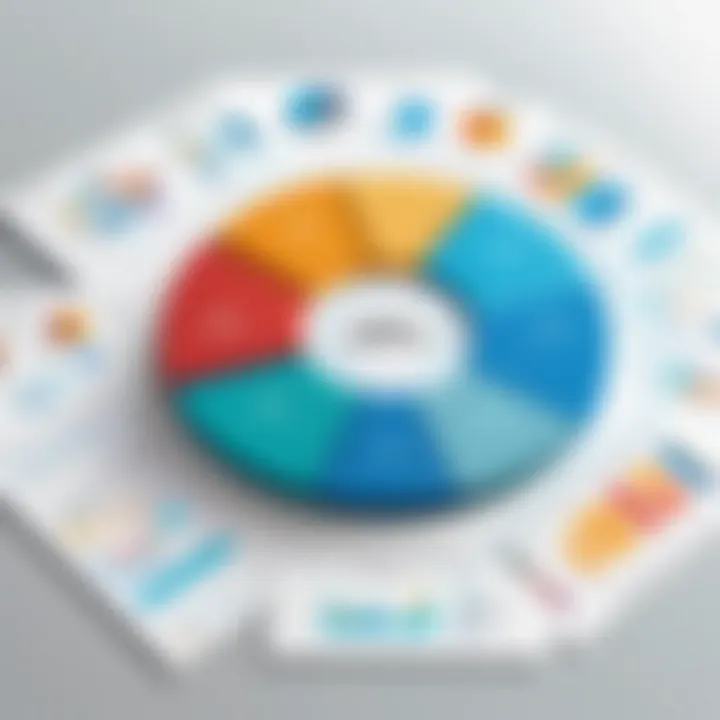
Intro
Value-based analytics represents a critical shift in the way organizations approach data analysis and decision making. It emphasizes not just the collection of data, but the transformation of that data into actionable insights which can drive improved outcomes across various sectors. Whether in healthcare, finance, or retail, organizations leveraging value-based analytics can expect to enhance their performance, optimize resource allocation, and ultimately improve their bottom line.
With the need for informed decision-making at an all-time high, businesses are increasingly turning to value-based analytics as a solution to harness the power of data. This article will explore the fundamental concepts of value-based analytics, its core components, strategies for implementation, challenges faced during adoption, and best practices to navigate these issues. Through this exploration, we will provide readers a comprehensive guide tailored particularly for IT professionals, software experts, and decision-makers within small and large businesses.
Understanding Value-Based Analytics
Value-based analytics plays a crucial role in the contemporary landscape of decision-making. This approach transcends traditional methods that rely on raw data alone. Instead, it emphasizes the integration of data insights into the organization's broader strategic framework. By understanding value-based analytics, organizations can transform their operations, making informed decisions that optimize outcomes.
Definition and Scope of Value-Based Analytics
Value-based analytics can be defined as a methodology that combines data analysis with an emphasis on delivering value to stakeholders. This often involves measuring performance, analyzing customer feedback, and employing statistical techniques to assess the impact of various choices. The scope of value-based analytics extends across industries, catering to sectors such as healthcare, finance, and manufacturing.
Organizations leverage various techniques such as predictive analytics, performance metrics, and qualitative assessments to ensure that decisions are informed and aligned with strategic goals. The needs of each business can shape how value-based analytics is implemented. For example, a healthcare provider might focus on patient outcomes, while a financial institution may prioritize investment returns.
Historical Context and Evolution
The roots of value-based analytics can be traced back to the evolution of data analysis tools and techniques over the past few decades. Initially, organizations collected data primarily for compliance and reporting purposes. However, as data became more accessible, companies began to recognize its potential for enhancing decision-making.
The rise of technology, particularly big data and machine learning, further accelerated this evolution. Companies started to employ sophisticated algorithms to analyze vast amounts of data in real time. The focus gradually shifted from merely collecting data to utilizing it effectively to drive improvements. The concept of value-based analytics emerged, offering a structured method for organizations to harness data strategically.
Importance in Modern Decision-Making
In today's fast-paced business environment, the ability to integrate value-based analytics into decision-making processes is non-negotiable. This integration allows organizations to:
- Make data-informed choices that cater to stakeholder needs.
- Enhance operational efficiency by identifying areas for improvement.
- Foster a culture of accountability by measuring outcomes against pre-defined targets.
- Predict future trends and behaviors through advanced analytical techniques.
By employing value-based analytics, organizations can navigate complexities more effectively. It equips leaders with insight into market dynamics, customer preferences, and operational efficiencies, thus supporting strategic objectives while ensuring sustainability.
As data continues to grow in importance, understanding and implementing value-based analytics not only drives better outcomes but also provides a competitive edge in the market.
Key Components of Value-Based Analytics
The foundation of value-based analytics lies in its essential components. Each part plays a crucial role in weaving together insights from data, ultimately guiding organizations toward better decision-making and improved outcomes. It is vital for any organization keen on harnessing the power of data to understand these components thoroughly and implement them effectively.
Data Collection Strategies
Data collection strategies are the backbone of value-based analytics. They define how data is gathered, which directly influences the quality and relevance of insights that can be drawn. Organizations must consider various sources for data collection, such as customer interactions, transaction records, surveys, and system logs. By employing both quantitative and qualitative methods, businesses gain a comprehensive view of trends and behaviors.
A combination of structured and unstructured data sources often provides richer insights. For example, structured data may include numeric reporting metrics, while unstructured data might encompass user comments on social media. Investing in automated data collection tools can enhance efficiency and accuracy, ensuring that important metrics are not overlooked.
Moreover, establishing clear guidelines for data governance is essential. Organizations need to ensure data quality, consistency, and security. A well-defined strategy for data collection enables businesses to obtain reliable data, essential for making informed decisions.
Analytical Techniques and Tools
After data is collected, the next step involves applying various analytical techniques and tools. These can range from basic statistical methods to complex algorithms powered by artificial intelligence. Using tools like R, Python, or specialized software can lead to more profound insights and support advanced analytics.
A critical aspect of analytical techniques is data visualization. By presenting analytical results visually, organizations can understand patterns quickly and make rapid decisions based on evidence. Dashboards and visual reportings are useful to track key performance indicators effectively.
Additionally, adopting machine learning techniques can significantly enhance predictive analytics capabilities. Predictive models refine the decision-making process by estimating future trends based on historical data. This is especially crucial in sectors like healthcare or finance, where timely insights can mitigate risks and optimize resources.
Integration with Business Intelligence Systems
The integration of value-based analytics with business intelligence systems cements its utility within an organization. Business intelligence tools enable organizations to absorb, process, and analyze data efficiently. Their seamless integration with value-based analytical methods creates a unified approach to data analysis and decision-making.
Organizations should focus on creating a coherent ecosystem of tools that facilitate data flow between analytics and business intelligence platforms. This helps maintain continuity in insights generation. By connecting systems such as SAP, Tableau, or Microsoft Power BI with analytics pipelines, businesses can ensure the consistency and relevance of their insights.
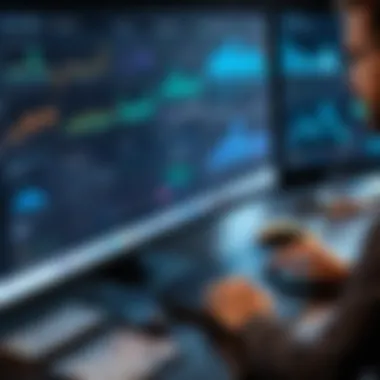
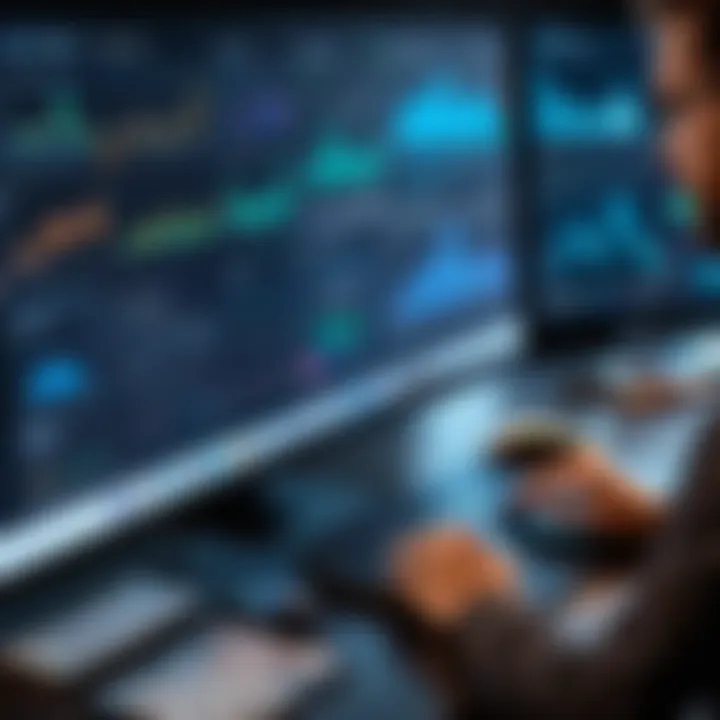
Furthermore, continuous monitoring and updating of integration processes are vital. As business needs and data sources evolve, so should the connections between value-based analytics and business intelligence systems. This proactivity enhances the capacity to respond quickly to market changes, client needs, and operational challenges.
"Integration is not about adding tools; it’s about creating an environment where insights inform actions across all levels of an organization."
The key components of value-based analytics drive its implementation and directly affect the value extracted from data. Organizations that optimize these components position themselves advantageously in their respective industries, facilitating data-driven decision-making that can significantly improve outcomes.
Implementing Value-Based Analytics
Value-based analytics plays a crucial role in the progress of organizations. This section discusses essential elements that must be considered for effective implementation.
Value-based analytics aids organizations in making informed decisions. By integrating data into business processes, it fosters outcomes that genuinely resonate with client needs. When businesses embrace analytics in decision-making, they not only heighten efficiency but also enhance service quality. Consequently, this implementation influences overall organizational effectiveness, promoting a culture inclined towards data-focused strategies.
Framework for Adaptation
Adopting value-based analytics involves establishing a structured framework. This framework helps organizations adapt to changing environments.
First, organizations should identify their specific goals. Setting clear objectives assists in focusing the analytic approach. Organizations should also invest in the right tools and technologies. Systems like Tableau or Microsoft Power BI facilitate data visualization and interpretation.
Second, businesses must assess their data infrastructure. Evaluating existing data resources among teams will ensure that gaps are identified early. Merging disparate data sources into one accessible platform enhances the analytic process.
Lastly, a stepwise approach to implementation is recommended. This approach allows businesses to progressively refine their techniques, ensuring sustainable long-term adoption.
Role of Leadership in Implementation
Leadership plays a vital role in driving the adaptation of value-based analytics. When leaders actively participate and champion initiatives, it encourages employees to adopt new practices.
Clear communication is paramount. Leaders should articulate the importance of data-driven decisions across all levels of the organization. They must also facilitate necessary training programs. This training helps staff develop the skills required to analyze and interpret data.
Furthermore, leaders should support a culture of experimentation. Encouraging teams to trial different analytic approaches can yield new insights, enhancing decision-making processes. When leadership is engaged, it creates an environment where innovation thrives.
Building a Data-Driven Culture
Creating a data-driven culture requires systematic efforts. Organizations need to establish values that prioritize analytics and evidence-based decisions.
Training and education are essential. Workers who understand data concepts contribute to a culture that recognizes the significance of insights derived from analytics. Consider organizing workshops or continuous learning programs tailored to employee needs.
Moreover, leadership must recognize and reward data-driven behaviors. Acknowledging teams who utilize analytics successfully fosters motivation and encourages others to follow suit.
Lastly, open communication is critical. Create forums where teams can share analytic findings. By doing so, organizations promote collaboration and inspire collective problem-solving. This collective effort cements the role of analytics in everyday decisions, influencing the entire organization's approach to various challenges.
"Value-based analytics turns data into actionable insights, allowing organizations to stay ahead in a competitive landscape."
Applications of Value-Based Analytics
In today's data-driven world, the applications of value-based analytics span various industries. The significance of these applications cannot be understated. Organizations employ this framework to analyze data, drive better strategic decisions, and enhance their operational efficiency. Value-based analytics provides actionable insights, allowing businesses to prioritize initiatives that meet their objectives. With a focus on specific sectors, this section reviews how various fields leverage value-based analytics to achieve tangible outcomes.
Healthcare Sector Applications
In healthcare, value-based analytics is transformative. It helps providers assess clinical practices, manage patient outcomes, and streamline operational processes. Hospitals can analyze patient data to lower readmission rates and improve care quality. For instance, using analytics tools, healthcare settings can track and predict disease outbreaks, thus enhancing preventive measures. Furthermore,
- Cost Reduction: Organizations pinpoint areas to reduce expenses while maintaining quality.
- Personalized Care: Analytics leads to customized treatment plans, which enhance patient satisfaction.
Overall, the integration of data into healthcare practices creates a foundation for improved decision-making and healthier patient outcomes.
Finance and Investment Strategies
The finance sector greatly benefits from value-based analytics. Investment firms utilize it to enhance portfolio management and risk assessment. The analysis allows for the identification of potential investment opportunities based on market trends and historical performance. Key aspects include:
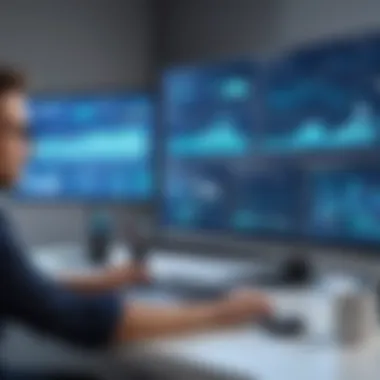
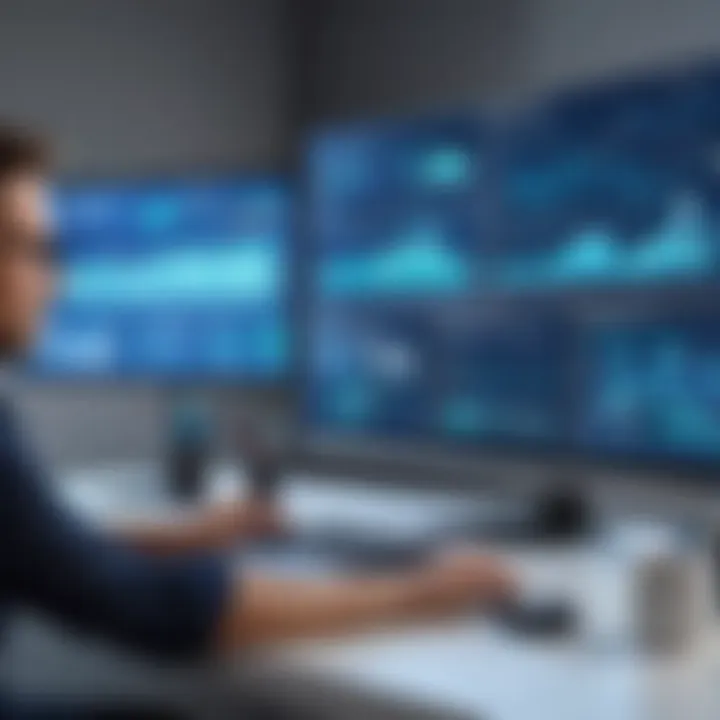
- Risk Management: Organizations assess financial risks through predictive analytics.
- Customer Insights: Financial institutions gain a deeper understanding of customer behaviors and preferences, leading to enhanced service offerings.
By applying value-based analytics, firms can better align their strategies with market realities, thus maximizing returns for stakeholders.
Marketing Analysis and Customer Insights
In marketing, data insights drive campaigns and boost customer engagement. Value-based analytics enables marketers to precisely target audiences and measure campaign effectiveness. Through various techniques, businesses can optimize their strategies. Considerations include:
- Segmentation: Detailed customer data analysis allows for precise market segmentation.
- ROI Measurement: Companies evaluate the return on investment from marketing efforts, enabling recalibrations for better results.
This analytical approach is fundamental for businesses to remain competitive in a crowded market.
Manufacturing and Supply Chain Optimization
Manufacturers face the challenge of efficiency and cost reduction. Value-based analytics offers solutions to streamline operations and enhance supply chain performance. Key trends reveal:
- Inventory Management: Companies can predict inventory needs, reducing overstock and stockouts.
- Production Efficiency: Analytics identifies production bottlenecks, leading to timely adjustments.
By leveraging data, manufacturing firms can ensure smoother operations, resulting in reduced costs and improved service delivery.
In all these sectors, the consistent application of value-based analytics fosters a culture of data-driven decision-making, ultimately leading to superior outcomes for organizations.
Challenges in Value-Based Analytics
Implementing value-based analytics is not without its challenges. Organizations face several hurdles that can impede their ability to make informed decisions based on data insights. Successfully navigating these challenges is crucial for achieving the desired outcomes of value-based analytics. Understanding these challenges helps in devising effective strategies that ensure the successful application of data analytics in various sectors.
Data Silos and Integration Issues
Data silos present a major challenge in value-based analytics. Organizations often deal with fragmented data streams that exist in isolated systems. These silos limit the ability to gain a comprehensive view of the organization’s data landscape. Integrating data from disparate sources is essential for generating accurate insights. Without proper integration, analyses may lead to poor decision-making.
The importance of addressing data silos cannot be overstated. Organizations must invest in data integration tools and practices. These tools help unify data from various sources, making it accessible and usable across departments. Therefore, breaking down these silos is vital for comprehensive analytics, allowing for a clearer, more holistic understanding of data.
Skill Gaps and Training Requirements
Another significant hurdle is the skill gaps that professionals may face when adopting value-based analytics. The field of data analytics is evolving rapidly, and organizations often find themselves lacking the necessary expertise. Employees may require upskilling or reskilling to efficiently work with analytical tools and methodologies.
Focusing on training is essential for mitigating this challenge. Organizations should create training programs that enhance employees' analytical competencies. This involves structured learning paths that cover both foundational concepts and advanced techniques. Investing in human capital ultimately enhances the organization's capacity to leverage data-driven insights.
Ethical Considerations and Data Privacy
Ethical concerns related to data privacy are increasingly significant in the realm of value-based analytics. Organizations must navigate the complex landscape of data governance and ensure compliance with regulations, such as the General Data Protection Regulation (GDPR). Failing to address these considerations can lead to severe consequences, including legal repercussions and reputational damage.
It is crucial for organizations to establish clear policies about data usage. This involves defining ethical standards for data collection and analysis. Stakeholder transparency builds trust and assures customers that their data is used responsibly. Implementing robust data governance frameworks helps in maintaining ethical practices in data analytics, keeping compliance and consumer trust intact.
"Data governance is not just about compliance; it's about building trust with stakeholders."
These challenges are integral to the discourse on value-based analytics. Organizations must confront these obstacles to harness the potential of their data. A proactive approach is necessary, ensuring that organizations are well-equipped to derive insightful analytics for better decision-making.
Best Practices for Successful Implementation
Successful implementation of value-based analytics is essential for maximizing the benefits from data insights. Best practices guide organizations in effectively integrating analytics into their decision-making frameworks. Effective practices lead to improved outcomes, better resource allocation, and increased competitive advantage. Organizations can harness the full potential of their data when they follow a structured approach to implementation.
Establishing Clear Objectives
Setting clear objectives is the foundation of any successful analytics initiative. Without defined goals, teams may struggle to focus their efforts, leading to wasted resources and ineffective use of data. Objectives must align with the organization's strategic vision. Teams should consider what success looks like in measurable terms. Key performance indicators (KPIs) can provide tangible criteria against which the effectiveness of analytics efforts can be evaluated.
For example, in healthcare, a clear objective might focus on reducing patient readmission rates. In a retail context, it could involve increasing customer retention through personalized marketing campaigns. By setting these clear benchmarks, organizations can enhance accountability and ensure that all stakeholders understand the purpose of data-driven initiatives.

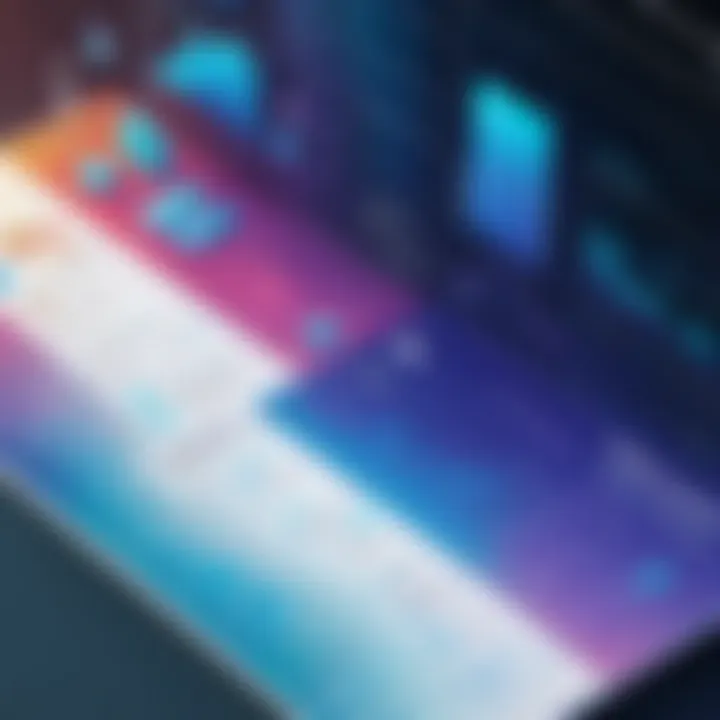
Continuous Monitoring and Evaluation
Continuous monitoring and evaluation are critical steps in maintaining the relevance and effectiveness of value-based analytics. Regular checks allow organizations to assess the ongoing performance of their analytics projects and make necessary adjustments. This proactive approach not only helps in fine-tuning analytical models but also identifies areas where improvements can be made.
Establishing routines for assessment at different stages can spotlight trends and patterns that may require attention. Adopting a framework for feedback can foster a culture of constant improvement. Key insights derived from data should be regularly shared with stakeholders to emphasize the positive impacts of analytics on organizational objectives. This transparency can enhance trust in the analytics process.
Fostering Collaboration Across Departments
Collaboration across departments enhances the implementation of value-based analytics. When different teams work together, they share diverse perspectives and expertise that enrich the analytical insights drawn from data. Often, data is distributed across various silos. By fostering inter-departmental collaboration, organizations can ensure that relevant data is shared and utilized effectively.
Creating cross-functional teams that include members from IT, marketing, finance, and operations can lead to more holistic insights. Regular meetings and collaborative projects can bridge the divide between departments, helping everyone to contribute to analytics goals. This cohesive approach not only enhances problem-solving abilities but also aligns the whole organization around common objectives.
"Collaborative efforts maximize data's potential and lead to more informed decision-making."
These best practices provide a framework for organizations to implement value-based analytics effectively. Establishing clear objectives, engaging in continuous evaluation, and fostering collaboration create a strong foundation for success, enabling organizations to harness the power of data insights.
Future Trends in Value-Based Analytics
The evolution of value-based analytics reflects ongoing advancements in technology and changing organizational needs. Understanding these future trends is vital for businesses to leverage data effectively, maintain competitiveness, and optimize decision-making processes. Through the exploration of these trends, organizations can identify new opportunities, refine their strategies, and align with best practices that will shape their future landscapes.
Advancements in Artificial Intelligence and Machine Learning
As organizations increasingly rely on data, the role of artificial intelligence (AI) and machine learning (ML) gains prominence. These technologies facilitate deeper insights from complex datasets, allowing for more sophisticated analyses. Machine learning algorithms can identify patterns that may not be evident through traditional statistical methods, enabling organizations to make data-driven decisions. For instance, AI helps automate data preprocessing, leading to faster and more accurate analytics processes.
Moreover, businesses can employ AI for predictive modeling, which enhances operational efficiency and customer engagement. When organizations leverage AI and ML in their analytics framework, they stand to gain significant advantages in resource allocation, market prediction, and personalized services.
The Growing Role of Predictive Analytics
Predictive analytics encompasses techniques that analyze current and historical data to anticipate future outcomes. Its prominence is rising as organizations seek to stay ahead of market trends. By integrating predictive analytics into their value-based frameworks, companies can forecast customer behavior, optimize resource distribution, and reduce operational risks.
The application is diverse, spanning across industries such as finance, where risk assessments become more precise, or healthcare, where patient outcomes can be anticipated. This trend underscores the need for analytics professionals to focus on predictive models that are adaptable to the specific challenges of their industries.
Integration of Real-Time Data for Decision-Making
The demand for real-time data integration is becoming essential in value-based analytics. Immediate access to data not only enhances decision-making speed but also improves accuracy. Real-time data allows organizations to react quickly to changes in their environment, whether in customer preferences or operational efficiencies.
Organizations can utilize technologies like IoT (Internet of Things) to gather and analyze data in real-time. The capability to track metrics on-the-go generates timely insights, driving informed decisions that align with corporate goals. The use of real-time data will continue to expand as businesses recognize its importance in maintaining an agile and responsive approach.
"Organizations that embrace real-time data integration will likely see a marked improvement in their adaptability and strategic planning."
These future trends in value-based analytics highlight the importance of continuous evolution and adaptation. By staying abreast of advancements in AI, predictive analytics, and real-time data integration, businesses can foster a data-informed culture that enhances their overall effectiveness.
End: The Impact of Value-Based Analytics
Value-based analytics represents a transformative approach within data utilization. This methodology promotes an alignment of analytical efforts with strategic business goals. By leveraging data insights, organizations can enhance operational efficiency and drive innovation. The impact of value-based analytics is manifold, influencing various aspects of decision-making and performance metrics.
Summarizing Key Insights
In examining value-based analytics, several key insights emerge. First, the integration of analytical processes into core decision-making frameworks is essential. Organizations adopting this approach see improved outcomes as data informs every aspect of their operations. Effective data collection strategies, alongside advanced analytical techniques, lay the groundwork for successful implementation. Organizations ought to continually refine these methods, ensuring they adapt to evolving market demands and technologies.
Moreover, fostering a culture that values data-driven decision-making is paramount. When leaders emphasize a data-centric mindset, employees at all levels become more engaged with analytical tools. This facilitates better collaboration across departments, promoting a holistic view of organizational performance.
Implications for Businesses and Organizations
The implications of value-based analytics are profound. For businesses, leveraging analytics can lead to a competitive edge. Understanding customer behavior through data insights allows companies to tailor their strategies effectively. This not only enhances customer satisfaction but also drives loyalty, ultimately contributing to sustained revenue growth.
Additionally, organizations in sectors like healthcare and finance significantly benefit from this analytical approach. They can streamline their services, reduce costs, and improve patient outcomes or portfolio performances. However, the transition towards value-based analytics also comes with challenges. Companies must navigate data silos and ensure compliance with privacy regulations while investing in training for their workforce.
The Path Forward
Moving forward, organizations must prioritize the implementation of value-based analytics. This involves establishing clear objectives that align with their strategic vision. Continuous monitoring is crucial; it allows organizations to evaluate their systems and make necessary adjustments to remain innovative.
Investment in technology, such as artificial intelligence and machine learning, will further enhance data analysis capabilities. By leveraging real-time data, organizations can respond swiftly to market changes. This agile approach will prove essential in an increasingly data-driven world.