Data Analysis and Visualization Software Overview
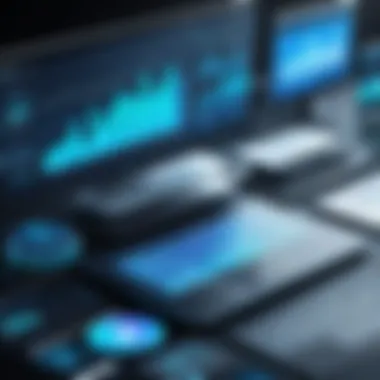
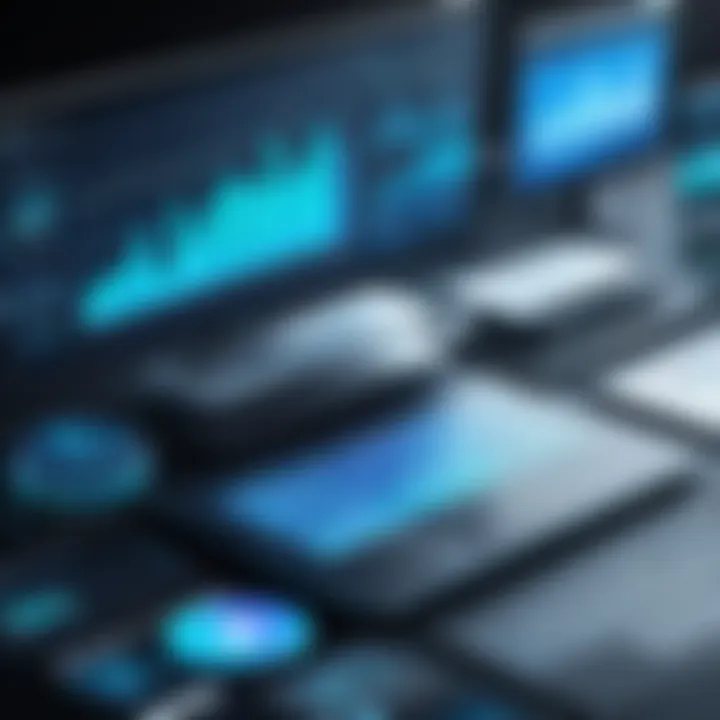
Intro
Data analysis and visualization software plays a significant role in the world of data-driven decision making. As organizations generate and collect vast amounts of data, the ability to analyze and visualize this information effectively becomes essential for making informed choices. These tools are not merely luxuries, but necessities in todayโs competitive environment.
This article will provide a comprehensive overview of various data analysis and visualization software. We will examine the functionality of these tools, notable options available in the market, user considerations, and prevalent trends shaping the industry. The goal is to inform professionals about best practices for selecting suitable software and facilitate a deeper understanding of the field.
Through this exploration, we will underscore the importance of compatibility with different data sources, the user interface's user-friendliness, and future developments in data analytics as technology advances rapidly.
Understanding Data Analysis
Data analysis is a strategic process that involves collecting, inspecting, transforming, and modeling data to discover useful information. The importance of understanding data analysis lies in its ability to inform decision-making processes in various fields. For businesses, utilizing robust data analysis leads to informed choices based on empirical evidence, ultimately improving efficiency and effectiveness. In this digital age, where data is generated at an unprecedented scale, mastering data analysis becomes crucial.
Definition and Importance
Data analysis refers to the systematic application of statistical and logical techniques to describe and evaluate data. It holds significance in drawing conclusions from data sets. The outcome of effective data analysis can lead to better business strategies, informed market understanding, and a clearer path to achieving organizational goals.
Types of Data Analysis
The realm of data analysis encompasses several distinct types, each serving specific purpose and insight generation. Understanding these types allows users to select appropriate methods for their data-related needs.
Descriptive Analysis
Descriptive analysis focuses on summarizing past data to understand trends and patterns. It answers the questions of "what happened" by providing insights into historical data. A key characteristic is its ability to transform complex datasets into understandable visual representations, such as charts or graphs. This form of analysis is popular among businesses when presenting reports to stakeholders.
The unique feature of descriptive analysis lies in its simplicity. It offers clear, concise insights without delving into causation, making it a beneficial choice for many scenarios. However, one disadvantage is that it does not provide explanations for the underlying causes of observed trends.
Diagnostic Analysis
Diagnostic analysis takes steps further by identifying why something happened. It examines causes for past results, looking for correlations and patterns within the data. The key characteristic here is its ability to enhance understanding of previous events, which often leads to informed decisions moving forward.
This type of analysis is beneficial, especially in problem-solving scenarios. It uncovers hidden issues behind data and gives context to the descriptive findings. A downside is the complexity inherent in this approach, requiring more sophisticated statistical methods and expertise.
Predictive Analysis
Predictive analysis aims to forecast future outcomes based on data patterns. Utilizing historical data, it employs statistical models and machine learning techniques to identify potential future events. The key characteristic is its forward-looking perspective, helping organizations anticipate market trends or customer behaviors.
Predictive analysis is powerful as it allows businesses to strategize accordingly. By forecasting future scenarios, organizations can allocate resources wisely. However, one must note that the accuracy of predictions is contingent on the quality of the input data.
Prescriptive Analysis
Prescriptive analysis provides recommendations on actions to take. It uses optimization and simulation algorithms to suggest various courses of action based on predictive outcomes. The key benefit is its ability to aid in decision-making by presenting options based on predicted scenarios.
This analysis type stands out due to its actionable insights. It empowers businesses to make informed choices that can optimize outcomes. However, the complexity and reliance on advanced algorithms can pose challenges for users lacking technical expertise.
Overall, understanding different types of data analysis is essential for selecting the right tools and approaches to transform data into actionable insights.
Prelude to Visualization Software
In the context of data analysis, visualization software plays a critical role. It enables professionals to interpret complex datasets through graphical representations. This transformation of data into visual formats helps in uncovering trends and patterns that may not be apparent through raw data alone. Visualization acts as a bridge between analytical insights and strategic decision-making.
By employing visualization tools, organizations can effectively communicate their findings. This is especially important when presenting data to stakeholders who may not have a technical background. Visualizations can simplify complex information and present it in a more digestible manner. As a result, visualization software becomes indispensable in modern data analysis workflows.
Role of Visualization in Data Analysis
Visualization enhances data analysis by allowing users to see relationships and insights in a more immediate way. For instance, charts and graphs make it easier to identify outliers, correlations, and overall trends. When data is arranged visually, one can quickly assess a situation without delving deeply into numbers.
Moreover, interactive visualizations enable users to engage with the data. They can filter, zoom, and explore different angles of the dataset. This capability supports in-depth analysis and allows for rapid adjustments based on real-time information.
Key Features of Visualization Tools
When selecting visualization software, there are specific features that stand out. These features enhance the usability and effectiveness of the tools, ultimately resulting in better data interpretation.
User-friendly Interface
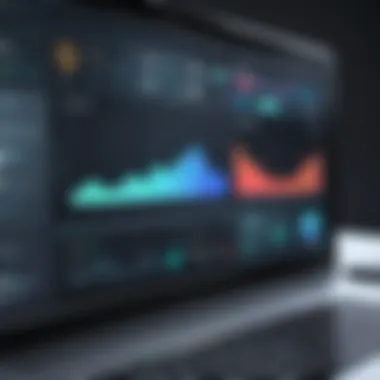
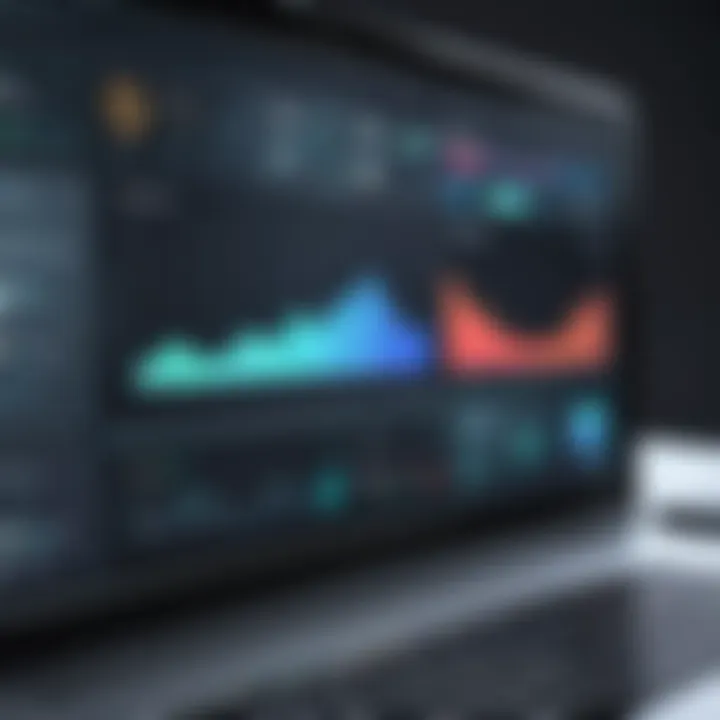
The user-friendly interface is a fundamental characteristic of effective visualization software. This aspect ensures that users can navigate the tool with ease, regardless of their technical ability. A clear and intuitive layout reduces the frustration that may arise from complex menus and functions. It allows users to focus on deriving insights rather than spending time learning how to operate the tool.
Unique features of user-friendly interfaces include drag-and-drop modules and guided workflows. These allow for quick report generation and visualization modifications without requiring extensive training. A responsive interface also contributes to overall user satisfaction.
Real-time Data Processing
Real-time data processing is another significant feature of advanced visualization software. This capability allows users to access and analyze data as it becomes available. In fast-paced business environments, the ability to visualize live data provides immediate insights that can drive decision-making.
Tools that offer this feature often integrate with existing databases or data streams. Such integration ensures that what users see on their dashboards is always up-to-date. However, it is essential to consider the infrastructure and resources required to support real-time processing.
Customizable Dashboards
Customizable dashboards empower users to tailor their visualization experiences according to their needs. This flexibility lets professionals select which metrics and visual styles best convey their data's story. A customizable dashboard can display multiple data sources at once, providing a holistic view of operations.
The advantage of customizable dashboards lies in their adaptability. Users can modify layouts or switch out visual elements based on real-time feedback and preferences. However, excessive customization can sometimes lead to confusion, especially for new users who may struggle to find key metrics.
In summary, visualization software is critical in enhancing data interpretation. Its role is vital as organizations strive to make data-driven decisions.
Through an understanding of the features and significance of visualization tools, users can make informed choices regarding software that aligns with their analytical goals.
Evaluating Data Analysis and Visualization Software
Evaluating data analysis and visualization software is crucial for professionals navigating the complex landscape of data-driven decision making. The right software tools can substantially impact the quality and accuracy of insights derived from data. Therefore, understanding the criteria under which these tools are assessed is essential for choosing the right options. Evaluating different software can streamline processes, enhance productivity, and improve overall outcomes in data analysis.
Criteria for Selection
Functionality
Functionality encompasses the features and capabilities of data analysis and visualization software. This aspect is fundamental as it determines how effectively the software can perform tasks relevant to data analysis, ranging from simple data manipulation to advanced statistical computations. A key characteristic of functionality is its direct influence on analytical outcomes. For instance, robust functionality allows users to conduct deep dives into data without unnecessary complications.
Unique Feature: Advanced data transformation features can create complex analyses easily. This provides significant advantages in scenarios demanding comprehensive reporting and visualization. However, overly complex functionality can deter less experienced users, making it less accessible for some.
Cost Effectiveness
Cost effectiveness evaluates the financial implications of implementing data analysis and visualization software. This criterion is vital since budget constraints often dictate software choices in organizations. A primary characteristic of cost effectiveness is the balance between features offered and the price point. Software that delivers a plethora of features at a reasonable price tends to be favored.
Unique Feature: Subscription models might offer flexibility for businesses while also allowing for scalable solutions. However, hidden costs related to updates or support can be a disadvantage, potentially increasing long-term expenses.
Integration with Existing Systems
Integration with existing systems addresses how well new software can connect and function with current tools and platforms. This is an essential factor as the ability to seamlessly combine new applications with legacy systems can enhance data workflow efficiency. A key characteristic of this integration is its capacity to reduce manual data entry and minimize errors.
Unique Feature: APIs (Application Programming Interfaces) facilitate data exchange and maximize functionality between systems. However, challenges may arise with incompatible systems, requiring additional resources for resolution.
User Experience Factors
Learning Curve
The learning curve pertains to the time and effort required for users to become proficient with the software. This is significant because it directly impacts productivity. A beneficial choice for organizations is software that has a shorter learning curve, allowing users to quickly adapt to new systems without extensive training.
Unique Feature: User-friendly tutorials and resources enhance the learning experience. Conversely, software with steep learning curves can impede initial adoption and frustrate potential users.
Customer Support
Customer support plays a vital role in the user experience. Accessible and responsive customer service can enhance the overall experience with the software, minimizing disruptions to workflow. A primary characteristic is the availability of support channels such as chat, email, or phone.
Unique Feature: Comprehensive documentation and community forums can empower users to solve problems independently. However, insufficient support can lead to user frustration and increased downtime in critical situations.
Community Resources
Community resources refer to the knowledge base and support systems provided by the software community. An active community can foster knowledge sharing and innovation, making it a beneficial aspect for users of all skill levels. A distinctive characteristic of community resources is the exchange of ideas and solutions among users, which can be invaluable for practical insights.
Unique Feature: Online forums and user groups can serve as platforms for problem-solving and idea generation. Nonetheless, reliance on community resources might sometimes lead to misinformation if not properly managed.
Popular Data Analysis Software Options
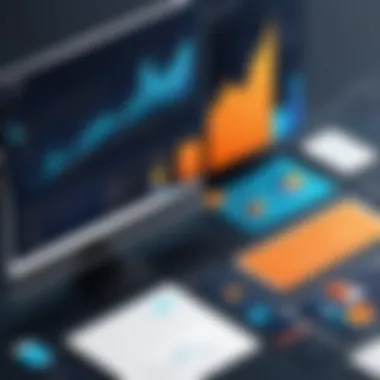
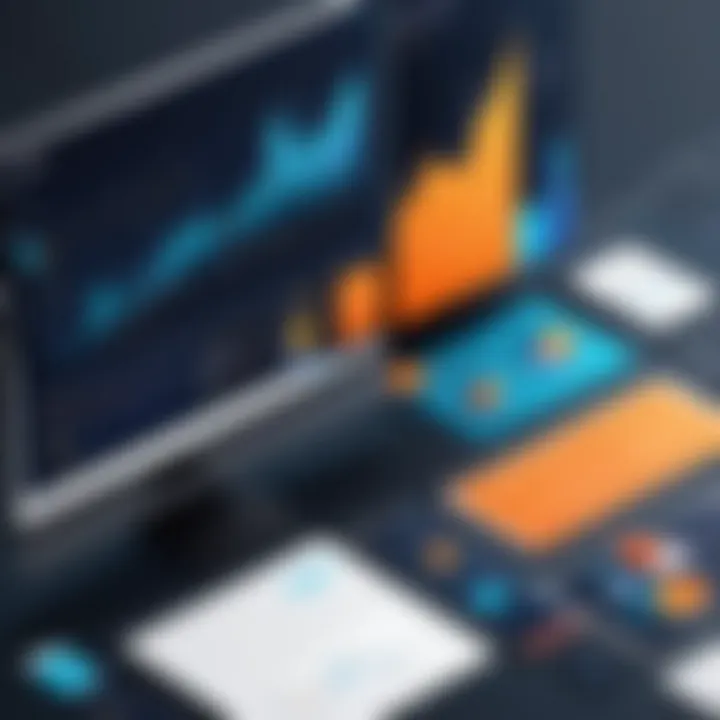
In the current landscape of data-driven decision making, the choice of software for data analysis holds significant weight. This section will delve into some of the most widely recognized options available. Each of these tools offers distinct features, advantages, and limitations that cater to various needs in the field.
Excel
Overview and Features
Microsoft Excel is perhaps the most ubiquitous software used for data analysis across many industries. One of its key characteristics is its user-friendliness, making it accessible for both novice and experienced users. Excel provides a range of functionalities, including pivot tables, data filtering, and basic statistical functions. Its formulas and functions allow users to perform complex calculations with ease. The major advantage of Excel is its widespread use and familiarity among users.
However, while Excel is beneficial for basic data manipulation, it has limitations. It struggles with large datasets and can become sluggish when handling extensive data, which can frustrate users needing to analyze significant amounts of information efficiently.
Use Cases and Limitations
Excel shines in various scenarios. It is commonly utilized for tasks like budget tracking, simple statistical analysis, and small-scale data presentations. Its spreadsheet format allows for organized data input and editing, facilitating quick analysis.
Nevertheless, there are notable limitations. Excel's capabilities in advanced statistical analysis are inferior compared to specialized software. Moreover, collaborative work can be cumbersome, as multiple users cannot efficiently edit a file simultaneously without version control issues.
R
Overview and Features
R is a programming language tailored for statistical computing and graphics. It boasts a rich ecosystem of packages that extend its capabilities far beyond traditional statistical methods. One of R's defining features is its vast library, allowing users to perform complex analyses and data visualizations seamlessly. Its syntactic structure is designed for statistical tasks, making it a favorable option for data scientists.
R's popularity in the academic and professional realms stems from its open-source nature, allowing continuous improvement and contribution from the global community. However, its learning curve can be steep for users unfamiliar with coding.
Strengths in Statistical Analysis
R excels in performing intricate statistical analyses. Its capability to handle various data types makes it versatile. Moreover, R supports advanced methods like machine learning and data mining, showcasing its strength in extracting insights from data.
The unique feature of R is its graphical capabilities. R can produce high-quality graphs and visualizations that are customizable to a great extent. However, users must invest time in learning its intricacies to leverage its full potential effectively.
Python
Overview and Features
Python is another powerful tool widely used in data analysis. Renowned for its readability and versatility, Python has a robust set of libraries dedicated to data manipulation and analysis. Libraries like Pandas and NumPy enhance its capabilities, allowing users to work with complex data structures easily. Pythonโs syntax is straightforward, promoting clean coding practices which many users appreciate.
Due to its general-purpose nature, Python can be integrated into various applications, making it a flexible choice for organizations.
Libraries for Data Analysis
Python's extensive libraries are a crucial feature that sets it apart. Libraries such as Matplotlib and Seaborn facilitate advanced data visualization, while Scikit-learn supports machine learning tasks. The availability of these libraries means that users can create comprehensive pipelines for data analysis.
One significant advantage is that Python fosters a supportive community, ensuring ample resources and documentation for learners. However, as with R, beginners may find it challenging initially to navigate more advanced libraries and functionalities.
Tableau
Visualization Capabilities
Tableau is a leading platform in the data visualization realm. It focuses on providing interactive and shareable dashboards that depict trends and insights in data effectively. One of its key characteristics is its ability to connect with numerous data sources, enabling users to visualize data in a manner that is both intuitive and informative.
Tableau's strength lies in its drag-and-drop interface, which allows users to build complex visualizations without deep technical knowledge, making it very appealing for business users.
Business Intelligence Applications
In the context of business intelligence, Tableau plays a pivotal role. It allows organizations to derive actionable insights from their data, aiding decision-makers in driving strategic initiatives. Its integration capabilities mean it can pull data from various sources, providing a comprehensive view of performance metrics.
The unique feature of Tableau is its focus on storytelling through data. This capability allows even non-technical users to present data compellingly. Nonetheless, the cost of Tableau can be a barrier for some smaller organizations, limiting wider adoption.
Emerging Trends in Data Analysis and Visualization
Emerging trends in data analysis and visualization significantly shape how organizations approach data-driven decision-making. These trends highlight the evolving landscape of technology and the critical role software plays in interpreting data. Professionals in IT and business must stay informed about these developments to remain competitive and efficient in their operations. The three key trends shaping this area include artificial intelligence integration, cloud-based solutions, and data democratization. Each trend presents unique benefits and considerations that can enhance organizational capabilities.
Artificial Intelligence Integration
The integration of artificial intelligence into data analysis and visualization tools represents a powerful shift in how data insights are generated. AI can automate processes such as data wading and cleaning, which are often time-consuming and resource-intensive. By leveraging machine learning algorithms, organizations can analyze vast datasets more efficiently, uncovering patterns and insights that may be missed through traditional analysis techniques.
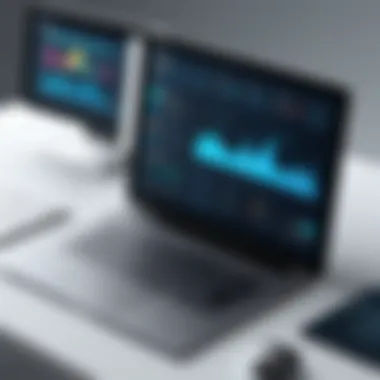
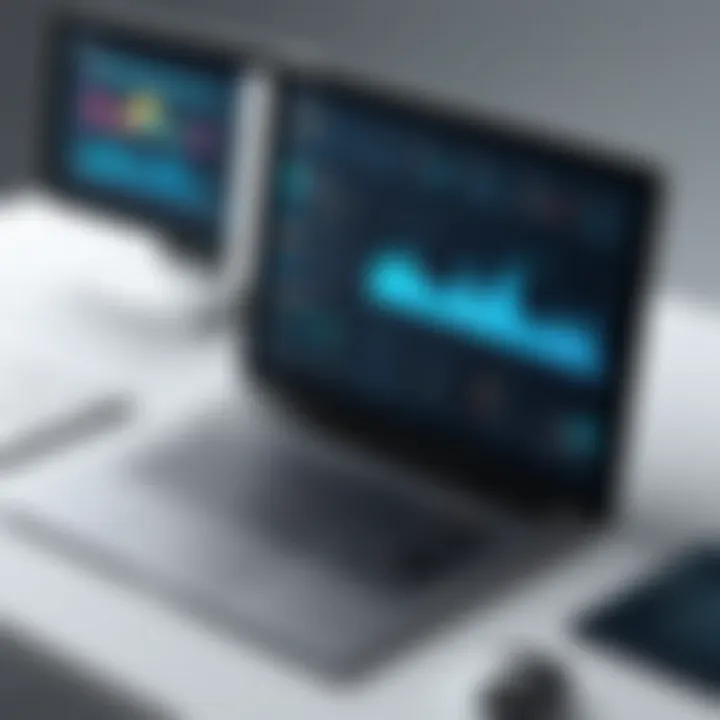
Benefits of AI integration include:
- Enhanced Predictive Analytics: AI can improve forecasting accuracy, allowing businesses to make more informed decisions based on likelihood and risk.
- Automation of Repetitive Tasks: Many menial tasks within data analysis can be automated, freeing up human resources for more strategic roles.
- Improved Visualization Techniques: AI can help create dynamic visualizations that adapt to real-time data, offering users immediate insights into changing trends.
However, organizations must also consider potential biases in AI algorithms. The quality of input data directly influences outputs, hence data quality assurance becomes paramount.
Cloud-Based Solutions
Cloud-based solutions are transforming the way data analysis and visualization software is deployed and managed. These solutions offer flexible access to resources, enabling teams to work collaboratively from anywhere with internet connectivity. This both reduces infrastructure costs and simplifies the maintenance of hardware and software, as providers often handle updates and security issues.
Key advantages of cloud computing in this context include:
- Scalability: Organizations can adjust their resources according to their needs without investing in physical infrastructure.
- Accessibility: Teams can access data and visualization tools simultaneously, facilitating better collaboration and insight sharing.
- Cost-Effectiveness: Many cloud solutions operate on a subscription basis, reducing upfront costs associated with traditional software licensing.
Despite these advantages, organizations must remain aware of security concerns. Data exposure in the cloud demands rigorous security protocols and compliance with data protection regulations.
Data Democratization
Data democratization refers to the process of making data accessible to non-technical users within an organization. This trend is rooted in the belief that everyone should be able to utilize data and insights to inform their decisions, not just those with specialized skills or knowledge.
The implications of data democratization include:
- Increased Empowerment: Employees can directly engage with data, fostering a culture of informed decision-making across all levels.
- Faster Decision-Making: With easier access to data, teams can respond rapidly to developments, enhancing agility and responsiveness in business.
- Enhanced Collaboration: Collaboration improves when diverse teams can access a unified dataset, leading to more thorough analyses and richer discussions.
However, organizations must also establish clear guidelines and training programs. Appropriate governance standards are crucial to ensure data is used ethically and responsibly.
*"Emerging trends such as AI integration and cloud-based solutions are revolutionizing data analysis, empowering firms to make informed decisions swiftly and effectively."
Challenges in Data Analysis and Visualization
In the domain of data analysis and visualization, various challenges can significantly impact the effectiveness and efficiency of processes. Addressing those challenges is paramount for organizations aiming to make the best data-driven decisions. Recognizing potential pitfalls not only enhances software selection but also informs strategies for overcoming these issues. Several key elements such as data quality, scalability, and user experience must be taken into consideration when discussing these challenges.
"Data is not just numbers; it's the story of the world around us."
Data Quality Issues
Data quality remains a fundamental challenge in data analysis and visualization. Inaccurate, incomplete, or inconsistent data can lead to erroneous interpretations and misinformed decisions. The roots of data quality issues can often be traced back to data entry errors, lack of standardized processes, or even the merging of datasets from different sources with varying formats and structures.
To address data quality issues, organizations must invest in robust data governance frameworks. Implementing systematic data cleaning procedures can help identify and rectify inconsistencies. Regular audits and validation checks should be standard practice, ensuring that the data being analyzed truly reflect the metrics needed for informed decision-making.
Moreover, employing automated data quality tools can significantly alleviate the burden of manual corrections. These tools often incorporate machine learning algorithms to detect anomalies and flag potential issues promptly. By enhancing data integrity through proper measures, companies can ensure their analysis produces reliable and actionable insights.
Scalability Concerns
Scalability is another critical concern that organizations face when dealing with data analysis and visualization. As the volume of data grows, tools that performed well under lighter workloads may struggle to maintain their efficiency. The challenge primarily lies in ensuring that the software can handle increased data loads without compromising performance.
Designing systems that are easily scalable requires careful planning and foresight. It is crucial to choose software solutions that allow for seamless upgrades and integration with additional tools or datasets as needed. The use of cloud-based platforms, such as Google Cloud or Amazon Web Services, can help in scaling resources dynamically.
Balancing performance with cost is also a consideration. Organizations need to assess whether to invest in on-premise hardware or leverage cloud solutions that offer more flexibility in resource allocation. Ultimately, having a well-architected data strategy plays a vital role in ensuring the scalability of analysis processes.
In summary, acknowledging and addressing these challenges is essential for efficient data analysis and visualization. By focusing on improving data quality and ensuring scalability, businesses can navigate the complexities of the data landscape more effectively.
Future Outlook for Data Analysis and Visualization Software
The future of data analysis and visualization software is critical to understanding how businesses and organizations will manage their data. As data continues to grow in volume, variety, and velocity, it becomes even more important for software tools to evolve. Companies rely on these tools to make informed decisions. Thus, knowing what to expect in the coming years can provide insights into potential benefits and shape strategic planning.
Innovative Technologies on the Horizon
Several innovative technologies are emerging that will significantly impact data analysis and visualization. One key aspect is advancements in artificial intelligence and machine learning. These technologies allow software to automate complex analytical processes, receiving valuable insights almost instantaneously. Predictive models can analyze past data and provide forecasts with high precision, offering a competitive edge to early adopters.
Additionally, augmented analytics is gaining traction. This concept integrates automation into data preparation, insight generation, and sharing. The software will recommend the best way to visualize specific datasets, making it easier for users to derive meaningful insights without needing advanced analytical skills.
Furthermore, the implementation of natural language processing (NLP) will enhance user interactions. Soon, users might ask questions using everyday language and receive visualizations as responses, thus democratizing data analysis. This capability can significantly reduce barriers for non-technical users, making data accessible to a wider audience.
The Role of User Feedback
User feedback is paramount in shaping the development of data analysis and visualization software. As trends rapidly change, organizations need to align their tools with real-world requirements. Collecting input from users enables developers to identify pain points and areas for improvement.
Surveys, focus groups, and community forums can provide invaluable insights into user experiences. The tech community on platforms such as Reddit can serve as a resource for feedback, offering constructive criticism and innovative ideas from individuals who actively engage with these tools.
Moreover, iterative design practices that integrate user feedback throughout the development process can lead to software that better meets user needs. Customer support interactions can also offer insights, as understanding frequently asked questions can illuminate common challenges users face.