Exploring Decision Support Tools in Healthcare
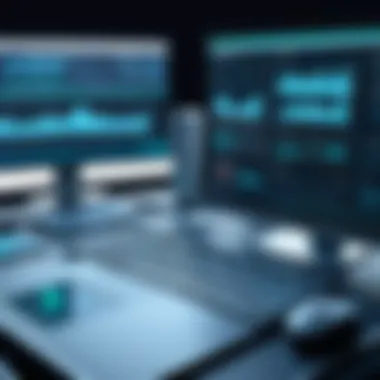
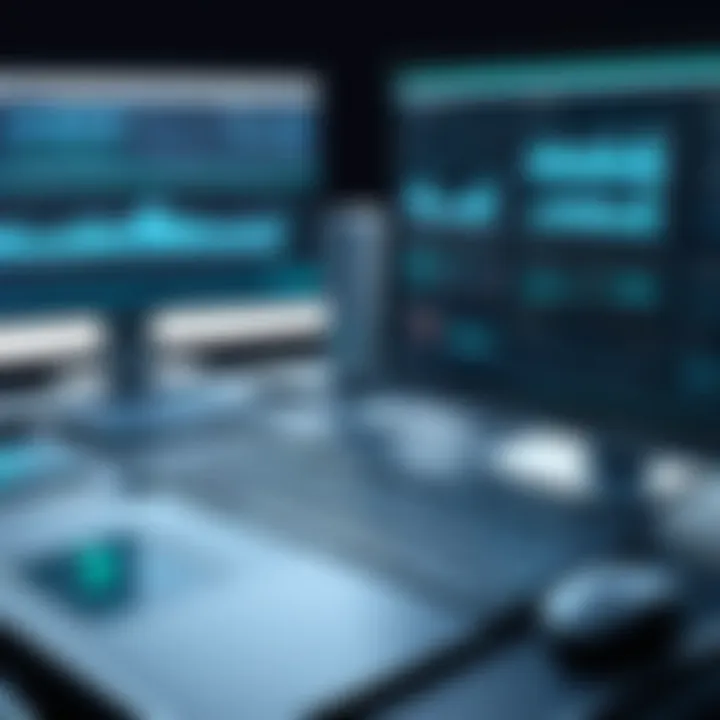
Intro
The integration of decision support tools in healthcare represents a significant shift in how medical practitioners approach patient care. These tools assist healthcare professionals in making informed choices by providing relevant information and analysis at critical moments during patient interactions. As the complexity of healthcare increases, the need for reliable and effective decision support tools becomes ever more pressing.
Decision support systems are not merely add-ons; they are becoming essential components of modern healthcare infrastructure. Their contribution to reducing errors, optimizing treatment pathways, and enhancing patient outcomes cannot be underestimated. Understanding the framework, application, and technology behind these tools is crucial for IT professionals, software developers, and healthcare providers alike.
By examining how decision support tools operate, the technologies that fuel their development, and the challenges associated with integrating them into practical workflows, this article aims to illuminate their significance in today’s healthcare landscape. As the industry evolves, so do these tools, and keeping abreast of the latest trends and developments will ensure that professionals are equipped to harness their full potential.
Prologue to Decision Support Tools
In the evolving landscape of healthcare, decision support tools play a pivotal role in assisting healthcare professionals. These tools enhance clinical decision-making, streamline processes, and improve patient outcomes. Their significance stems from the ability to provide accurate data, relevant insights, and timely recommendations in various healthcare settings.
The growing complexity of medical data necessitates effective decision support. As healthcare faces evolving challenges, integrating technology becomes key to addressing these needs. Through this lens, understanding decision support tools is crucial for professionals seeking to optimize patient care and operational efficiency.
Definition and Purpose
Decision support tools are systems designed to aid healthcare providers in making informed clinical decisions. These tools leverage data, knowledge, and artificial intelligence to support diagnosis and treatment processes. They include a range of applications, from basic data management systems to sophisticated machine learning models.
The purpose of these tools is to enhance the accuracy of clinical assessments, reduce errors, and offer evidence-based recommendations. By synthesizing large volumes of data, they assist in identifying patterns that may not be immediately apparent to the clinician. This ultimately leads to improved patient outcomes and more effective resource utilization.
Historical Context
The origins of decision support tools in healthcare can be traced back to the late 20th century. Initially, these tools consisted of rudimentary systems aimed at assisting healthcare professionals with administrative tasks. Over time, the advent of computing technology paved the way for more advanced systems.
In the 1980s and 1990s, the development of Clinical Decision Support Systems (CDSS) marked a significant milestone. These systems began to incorporate clinical guidelines and patient data to facilitate decision-making. The growth of electronic health records further accelerated the evolution of decision support tools, allowing for more integrated and comprehensive clinical data analysis. Today, tools utilize cutting-edge technologies like artificial intelligence and big data analytics to provide real-time insights, reflecting advancements in both compute power and data accessibility.
Types of Decision Support Tools
Decision support tools are essential in the healthcare landscape. They enhance the quality of care and streamline clinical decision-making. Understanding the different types of these tools can help professionals choose the most effective solutions for their needs. With the right decision support tools, healthcare providers can improve efficiency, accuracy, and overall patient outcomes. Below is an overview of the main types of decision support tools available in the healthcare setting.
Clinical Decision Support Systems (CDSS)
Clinical Decision Support Systems (CDSS) are designed to assist healthcare providers in making informed clinical decisions. These systems analyze data from various sources, including patient records, research findings, and clinical guidelines. In turn, they offer recommendations tailored to individual patient needs.
CDSS can present alerts or reminders for preventive measures, suggest diagnoses, and recommend treatment options. The use of CDSS promotes evidence-based practice, thus reducing the risks of errors.
- Benefits:
- Increased diagnostic accuracy.
- Reduction of medication errors.
- Enhanced patient safety through timely alerts.
The implementation of CDSS can lead to a more supportive environment for healthcare practitioners, allowing them to focus more on patient care rather than solely on data interpretation.
Diagnostic Support Systems
Diagnostic Support Systems play a crucial role in aiding clinicians with diagnoses. These systems utilize algorithms to analyze symptoms, medical history, and test results. They can evaluate potential conditions and suggest possible diagnoses. Notably, these tools can leverage extensive databases of medical knowledge, enhancing the accuracy of assessments.
- Key Considerations:
- Require regular updates to remain accurate.
- Must be used in conjunction with clinician expertise.
Incorporating diagnostic support tools into practice can significantly enhance the clinical evaluation process, allowing practitioners to consider a wider range of possibilities for diagnosis.
Treatment Recommendation Systems
Treatment Recommendation Systems are another essential type of decision support tool. These systems provide healthcare professionals with treatment options based on the latest clinical guidelines and patient information. The recommendations are often generated through complex algorithms that consider a variety of factors, including comorbidities and patient preferences.
- Advantages:
- Streamlined decision-making process.
- Customized treatment plans catering to individual patients.
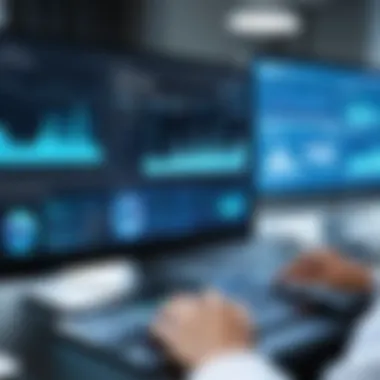
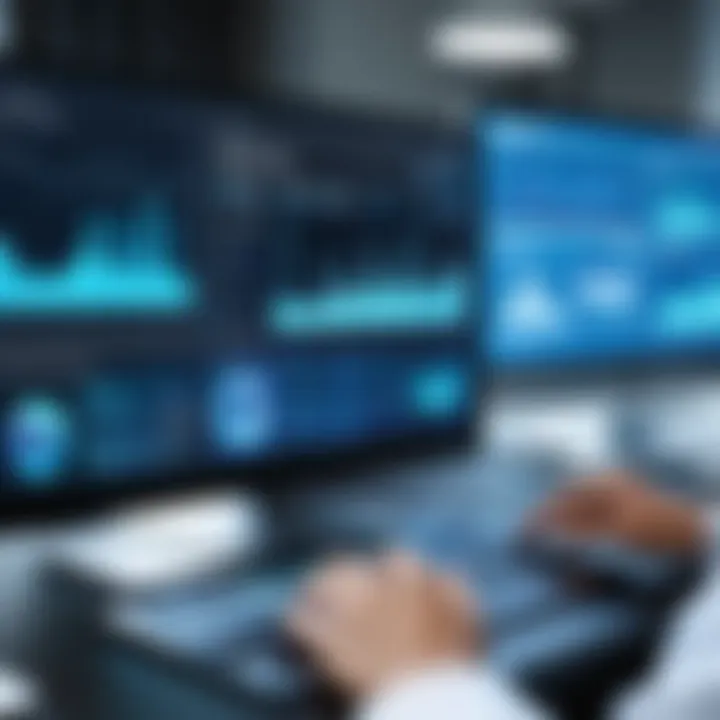
These tools foster a collaborative approach to treatment, ensuring that healthcare professionals engage patients in their care decisions.
Predictive Analytics Tools
Predictive Analytics Tools utilize big data to forecast health outcomes. They analyze patterns from historical data, helping healthcare providers anticipate patient needs and intervene proactively. This type of tool is particularly valuable in managing chronic diseases and improving resource allocation.
- Benefits:
- Enhanced capacity for risk stratification.
- Ability to customize prevention strategies according to patient risk.
As healthcare continues to evolve, the integration of predictive analytics plays a pivotal role in shaping proactive care strategies. Utilizing these tools allows healthcare professionals to be better prepared for patient care demands.
"Effective decision support tools have the potential to transform not only clinical practices but also patient experiences within healthcare systems."
Overall, recognizing and implementing various types of decision support tools is vital for improving decision-making processes in healthcare. The appropriate application of these tools enhances both efficiency and patient care.
Technology Behind Decision Support Tools
Understanding the technology driving decision support tools is crucial to their effective integration and functioning in healthcare systems. Various technological elements contribute to the development and optimization of these tools. They hold the potential to enhance clinical outcomes and improve healthcare service delivery.
Artificial Intelligence and Machine Learning
Artificial intelligence and machine learning form the backbone of many decision support systems in healthcare. These technologies enable the analysis of vast amounts of medical data to deliver insightful recommendations. AI can learn from existing data patterns, which allows it to improve its accuracy over time.
- Automation of Routine Tasks: By automating repetitive processes, healthcare professionals can save time and focus on more critical care activities.
- Predictive Capabilities: Machine learning algorithms can analyze historical patient data to predict future health outcomes, helping in preventive care.
Implementing these technologies involves challenges such as the need for extensive training data and the risk of overfitting, where a model performs well on training data but poorly on new, unseen data. However, the benefits often outweigh the limitations.
Data Analytics and Big Data
Data analytics is integral to decision support tools. The healthcare sector generates enormous volumes of data, known as big data. Harnessing this data requires sophisticated analytical techniques.
Data analytics can uncover trends, correlations, and anomalies that are not immediately visible. This can lead to:
- Better Patient Outcomes: Analytics can help in identifying which treatments are most effective for specific patient profiles.
- Cost Reduction: By making informed decisions based on thorough data analysis, healthcare organizations can avoid unnecessary procedures, thus lowering costs.
However, healthcare organizations must address data quality and interoperability issues when attempting to integrate analytics into their decision support systems. Ensuring high-quality data is essential for accurate insights and recommendations.
Interoperability Standards
Interoperability is critical in a fragmented healthcare environment. Various systems must communicate effectively to allow seamless information sharing.
Adhering to interoperability standards ensures that decision support tools can function across different platforms. This poses several benefits:
- Enhanced Communication: It enables healthcare providers to access patient information from different systems easily.
- Streamlined Workflows: When systems work together, it reduces duplication of efforts and improves workflow efficiency.
To achieve interoperability, standards such as HL7 and FHIR (Fast Healthcare Interoperability Resources) should be upheld by all healthcare systems. They provide guidelines to develop systems that can exchange and use health information appropriately.
"Adopting standardized protocols helps reduce errors and promotes effective care coordination."
Integration into Healthcare Workflows
Integrating decision support tools into healthcare workflows is pivotal for enhancing clinical practice and patient outcomes. Such integration ensures that healthcare professionals have timely access to relevant data and recommendations, fostering informed decision-making. This section delves into the complexities of this integration, emphasizing the various elements, benefits, and significant considerations involved.
Challenges of Implementation
Technical Barriers
Technical barriers are one of the foremost challenges faced when integrating decision support tools in healthcare settings. These barriers often refer to the limitations in existing IT infrastructure, software compatibility, and data interoperability between different systems. The key characteristic of technical barriers lies in the lack of standardization in healthcare technologies. Many hospitals use legacy systems that hinder the seamless implementation of newer decision support tools. This issue is beneficial to discuss as it highlights the need for cohesive strategies in tech adoption.
Unique features of technical barriers include outdated hardware and the rigidity of some healthcare systems which make upgrades difficult. This leads to increased implementation times and added costs. The disadvantage of these barriers is that they can significantly impede the daily workflow, frustrating healthcare professionals who rely on efficient systems for patient care.
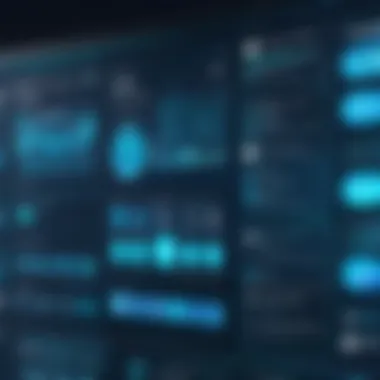
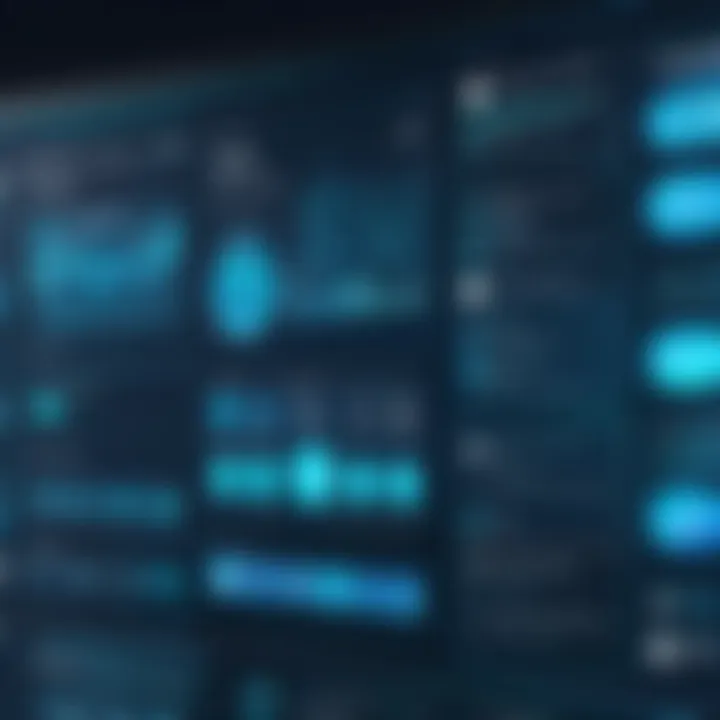
User Resistance
User resistance is another significant aspect of integrating decision support tools. This resistance often stems from fears about change, potential disruptions in workflow, and a general reluctance to adopt new technologies. The key characteristic here is the human element, where comfort with traditional practices can stand in the way of modernization. Discussing user resistance provides insight into behavioral aspects that impact the success of implementation efforts.
The unique feature of user resistance is that it can manifest in various forms, from passive reluctance to active opposition. This resistance can be detrimental, as it leads to underutilization or even rejection of valuable tools designed to improve healthcare delivery. Thus, understanding this resistance is crucial for developing effective change management strategies.
Cost Considerations
Cost considerations play a critical role in the implementation of decision support tools. Hospitals and clinics must evaluate not only the initial costs of technology but also ongoing maintenance and support expenses. The emphasis on cost considerations is beneficial since budgetary constraints often dictate the feasibility of integrating new systems into existing workflows.
A unique feature of cost considerations involves the long-term return on investment (ROI) associated with decision support tools. Although the upfront costs may be significant, the potential for enhanced efficiency, reduced errors, and overall improvement in patient outcomes might provide substantial financial justification. However, the downside includes the possibility of overspending on tools that may not meet the institution's needs.
Best Practices for Integration
Stakeholder Involvement
Involving stakeholders in the integration process is essential for developing effective decision support systems. Stakeholders can include healthcare providers, administrative staff, and even patients. Their involvement ensures that the tools address real-world challenges and that user needs are met. The key characteristic of stakeholder involvement is its ability to create a shared vision for technology adoption.
A unique feature of stakeholder involvement is the collaborative approach that may lead to the identification of unforeseen challenges and innovative solutions. The advantage of this practice is that it fosters a sense of ownership among users, which can directly reduce resistance and increase adoption rates.
Training and Support
Training and support for users are fundamental components of successful integration. Adequate training ensures that healthcare professionals are equipped to use decision support tools effectively. The key characteristic of training and support is that it provides users with the necessary context to understand how tools can enhance their workflows.
The unique feature of comprehensive training is ongoing support that aligns with users' needs. Continuous learning opportunities can make a significant difference in how tools are utilized. However, a disadvantage may arise in the form of resource allocation, as sustained training programs require time and financial investment.
Continuous Evaluation
Continuous evaluation is crucial for maintaining the effectiveness of decision support tools in healthcare workflows. It involves regularly assessing the performance of the tools and making necessary adjustments based on user feedback and clinical outcomes. The key characteristic of continuous evaluation is its iterative nature, which promotes an adaptive approach to technology use.
The unique feature of this practice is the establishment of metrics for success that can guide enhancements over time. The advantage of continuous evaluation is that it ensures decision support tools remain aligned with evolving healthcare standards and practices. Failure to evaluate effectively can lead to stagnation and reduced value of the tools over time.
In summary, effective integration of decision support tools requires addressing technical barriers, user resistance, and cost considerations while following best practices involving stakeholder involvement, training, and continuous evaluation.
These factors create a comprehensive framework that not only facilitates the adoption of technology but also enhances overall patient care in healthcare settings.
Impact on Patient Outcomes
The impact on patient outcomes is a critical area when discussing decision support tools in healthcare. These tools aim to facilitate better clinical decisions, which in turn influence overall patient health. Making informed and precise decisions is essential for improving health outcomes, reducing variability in care, and ensuring standardized treatment processes.
Improvement in Diagnostic Accuracy
Accuracy in diagnosis is fundamental in healthcare. Decision support tools such as Clinical Decision Support Systems (CDSS) utilize algorithms that analyze patient data and symptoms. By integrating medical literature and clinical guidelines, these systems help healthcare professionals identify potential conditions more accurately. Studies show that using these tools can result in a significant decrease in diagnostic errors. Errors that could lead to incorrect treatment or further complications are minimized.
Many healthcare institutions report improvements in diagnostic accuracy when employing these systems. This enhancement is paramount not only for patient satisfaction but also for optimizing resource allocation in hospitals.
Enhancements in Treatment Appropriateness
The appropriateness of treatment is another aspect heavily influenced by decision support tools. These tools can provide evidence-based treatment recommendations that align with clinical pathways. For example, when a doctor prescribes medication, a decision support system can cross-reference the patient’s profile, including allergies and drug interactions. This ensures that the treatment plan is tailored to the individual patient’s needs.
Various research indicates that treatment recommendations backed by these tools lead to better adherence to guidelines. As a result, patients receive treatments that are more aligned with best practices, ultimately leading to improved health outcomes and reduced instances of adverse effects.
Patient Safety and Risk Management
Patient safety is a top priority in healthcare and must be addressed with utmost seriousness. Decision support tools play a significant role in risk management by alerting clinicians to potential hazards. For instance, systems can flag anomalies in medication orders, such as inappropriate dosages or dangerous combinations of drugs.
By leveraging predictive analytics, these tools help in identifying patients at high risk for complications before they occur. This preemptive approach allows healthcare professionals to intervene swiftly, ultimately enhancing patient safety. According to studies, implementing decision support tools correlates with a reduction in hospital readmission rates and medical errors.
"The true potential of decision support tools lies in their ability to transform how clinical decisions are made, leading to safer and more effective patient care."
Overall, the impact of decision support tools on patient outcomes is profound. Improved diagnostic accuracy, enhancements in treatment appropriateness, and increased patient safety form the bedrock of an effective healthcare system.
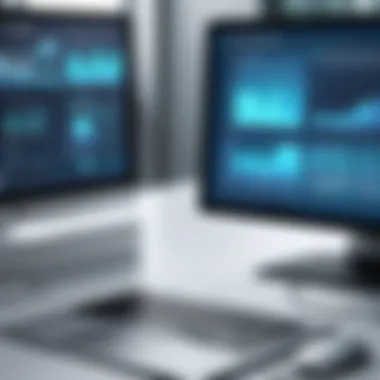
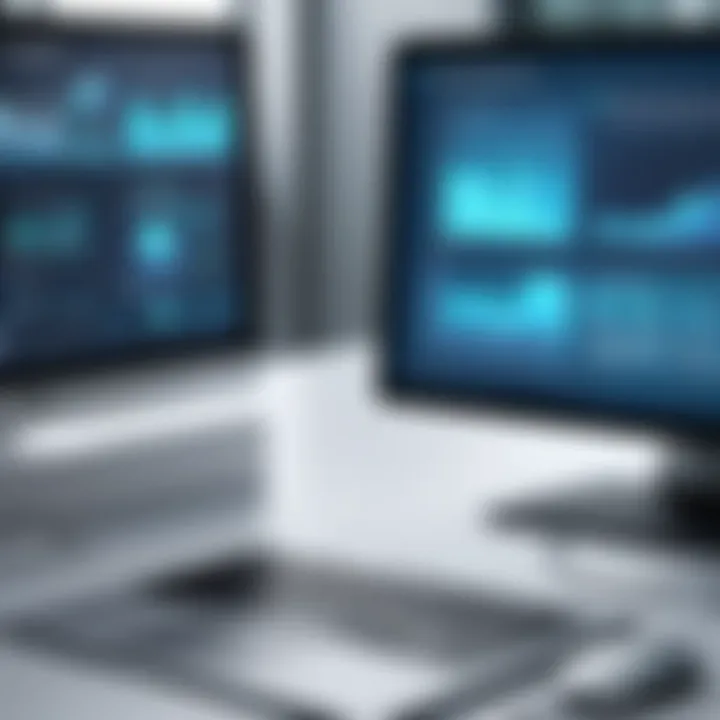
As these tools evolve, their significance in shaping patient care continues to grow. This trend offers promising prospects not only for practitioners but also for patients who stand to gain from advanced, precise decision-making.
Ethical Considerations
The ethical dimensions surrounding decision support tools in healthcare are vital for ensuring they are used appropriately and effectively. As these tools become more integrated into clinical practices, it becomes necessary to examine how they align with ethical standards in patient care. Ethical considerations help clarify the responsibilities of healthcare professionals and organizations. They address the implications of using advanced technologies in sensitive environments, particularly concerning patient autonomy and informed consent.
Data Privacy and Security
Data privacy is a primary concern when implementing decision support tools. These systems often rely on vast amounts of patient data, which requires stringent security measures to protect sensitive information. The Health Insurance Portability and Accountability Act (HIPAA) governs the confidentiality of patient information in the United States. Healthcare organizations must ensure that their decision support systems comply with these regulations. Failure to do so can lead to data breaches, resulting in severe repercussions for both patients and providers.
In addition to compliance, ensuring the security of data also entails implementing robust technical measures. This includes data encryption, secure user authentication, and regular system audits to detect vulnerabilities. Additionally, educating healthcare staff about best practices for data handling is essential. Having a culture that prioritizes data protection can help mitigate risks.
Bias in Algorithms and Data Use
Bias in algorithms presents a significant ethical challenge in the development and deployment of decision support tools. Algorithms often learn from historical data, which may contain biases reflective of existing inequalities in healthcare. If not addressed, these biases can perpetuate disparities in patient care. For example, algorithms trained on limited demographic data might struggle to provide accurate recommendations for underrepresented populations.
To counteract bias, developers should ensure diversity in data sets used for training algorithms. Continuous evaluation of these tools is also vital to identify any unintended consequences. Involving diverse stakeholders in the design and deployment process may broaden the perspectives in addressing bias. Regulatory bodies can set guidelines aimed at minimizing bias and promoting fairness in algorithmic processes.
"Ethical considerations in healthcare technology ensure that innovations support, rather than hinder, patient welfare."
In summary, navigating the ethical landscape of decision support tools is crucial. Data privacy and algorithmic bias are key areas that require scrutiny. By prioritizing ethical considerations, healthcare providers can enhance the reliability of these tools and support equitable patient care.
Future Trends in Decision Support Tools
The landscape of healthcare is evolving rapidly. As technology continues to advance, so too do the decision support tools that assist healthcare professionals. Understanding future trends in decision support tools is vital. It reveals how these innovations can transform patient care and clinical practices.
Decision support tools will likely incorporate more intuitive interfaces. This development aims to enhance usability for healthcare providers. A user-friendly design can lead to quicker decision-making and improved patient outcomes. Moreover, there is a growing emphasis on personalized medicine. Tools that adapt to an individual patient's data can provide tailored recommendations. This shift will facilitate a more targeted approach to healthcare.
Additionally, health data interoperability remains a crucial point of focus. As healthcare systems strive to communicate effectively with one another, decision support tools must ensure they harness and share data seamlessly. Such advancements in data connectivity will lead to comprehensive insights across various platforms. This is essential for effective decision-making and continuity of care.
"The future of healthcare signifies a shift towards greater integration of technology and human judgment."
Healthcare professionals should also expect to see an increased use of analytics and artificial intelligence. These technologies provide predictive insights. The ability to anticipate patient needs can significantly optimize treatment pathways. They also enhance risk management capabilities. The fusion of AI with decision support tools assists in identifying potential complications early on.
Furthermore, telemedicine plays an ever-increasing role in shaping decision support systems. As more patients turn to remote care, the integration of telehealth capabilities within decision support tools can streamline operations and improve accessibility. This aspect addresses the pressing need for flexible healthcare delivery, especially in rural or underserved regions.
Role of Telemedicine
Telemedicine represents a pivotal shift in healthcare delivery. It enhances accessibility and convenience for patients. With the rise of telemedicine, decision support tools must adapt accordingly to ensure effective care. Remote consultations demand that information be readily available to healthcare providers. This facilitates informed decision-making, even in virtual settings.
Telemedicine can also strengthen data collection. Providers can tap into real-time patient data, enriching the databases that decision support tools rely on. Furthermore, patients can receive immediate feedback on their health status through these platforms. This timely response empowers patients, enhancing their engagement in their own care.
Importantly, telemedicine helps to bridge gaps in communication between patients and providers. Decision support systems must not only include clinical data but also incorporate patient-reported outcomes. This integrative approach ensures that the tool addresses the specific needs of each patient.
Evolving Patient Engagement Platforms
Patient engagement is increasingly seen as a cornerstone of effective healthcare. As engagement platforms evolve, they are becoming more interactive and user-friendly. Decision support tools can capitalize on this trend by integrating features that encourage patient participation.
Incorporating mobile applications into decision support systems can empower patients to track their health. These platforms can provide reminders for medication adherence and appointments. They can also allow users to log symptoms and access educational resources. Engaging patients this way leads to their active involvement in treatment decisions. Patients that are more informed tend to have better health outcomes.
Furthermore, evolving patient engagement platforms often leverage gamification techniques. By introducing game-like elements, these tools can motivate patients to adhere to treatment plans. This innovative approach can reshape patient experiences and promote better health behaviors.
Also, patient feedback must be routinely collected and analyzed. This continuous input can create a feedback loop. It allows healthcare providers to adapt decision support tools to meet patient needs effectively.
End
The conclusion serves a critical role in the exploration of decision support tools in healthcare. Summing up the information discussed throughout the article is essential for reinforcing the understanding of how these systems influence clinical practices and patient outcomes. In this section, we highlight the key themes of the article, emphasizing the significance of integration and future advancements.
Decision support tools are not merely technological novelties; they have become integral to improving healthcare delivery. They aid practitioners in making informed, data-driven decisions, which enhances the overall standard of care. Moreover, their capacity to synthesize vast amounts of data into digestible insights cannot be overstated. This capacity directly correlates with several benefits. For instance, improved diagnostic accuracy can lead to timely interventions, while enhanced treatment recommendations promote the appropriateness of care plans.
"Decision support systems are transformative in redefining pathways to better healthcare delivery."
While recognizing the potential benefits, it is important to consider the challenges highlighted earlier in the article. Obstacles such as user resistance, costs, and technical barriers can impede the successful implementation of these tools. Hence, attention to best practices during integration is essential for overcoming these challenges.
Looking ahead, the trends in decision support tools suggest a maturation of technology that will continue to inform how healthcare is practiced. Innovations such as predictive analytics and the merging of telemedicine are anticipated to further enhance the decision-making landscape.
In summary, the concluding segment of this article encapsulates the depth of exploration into decision support tools, articulating their crucial role in healthcare systems today and their evolving nature in the future. The insights actionable are relevant for IT professionals, software experts, and stakeholders in the healthcare arena. With an understanding of these tools' capabilities and challenges, professionals are better equipped to navigate the dynamic landscape of healthcare technology.